In Silico Adme Techniques Used In Early-Phase Drug Discovery
TRANSLATING MOLECULES INTO MEDICINES: CROSS-FUNCTIONAL INTEGRATION AT THE DRUG DISCOVERY-DEVELOPMENT INTERFACE(2017)
Abstract
The process of drug discovery and development is time consuming and expensive. In silico tools, in combination with in vitro and in vivo models, provide a valuable resource to improve the efficiency of this process. In this chapter, we provide an overview of various in silico tools and models used to identify and resolve absorption, distribution, metabolism, and excretion (ADME) challenges in drug discovery. In general, structure-based in silico techniques such as docking and molecular dynamics simulations have limited applicability in the ADME space due to the promiscuity of many ADME targets and the limited availability of high-resolution 3-D structures. Pharmacophore models, a ligand-based in silico method, can be used to identify key structural features responsible for the interaction with the target of interest. However, due to broad ligand specificity and the probability of multiple binding sites in many ADME targets, pharmacophore models have limited prospective applicability across structurally diverse chemical scaffolds. Conversely, quantitative structure-property relationship (QSPR) models are capable of extracting knowledge from a wide variety of chemical scaffolds and have prospectively shown utility as predictive models for many ADME endpoints measured in the pharmaceutical industry. QSPR models, especially those based on machine learning techniques, are known to have limited interpretability. To address this challenge, the use of QSPR models is typically coupled with information derived from trends between ADME endpoints and physicochemical properties (e.g., lipophilicity, polar surface area, number of hydrogen bond donors, etc.) during drug discovery. Furthermore, knowledge extracted by the matched molecular pair analysis (MMPA) of ADME data provides insight that is used to identify fragment replacements to improve the ADME characteristics of compounds. In conclusion, an effective amalgamation of in silico tools is necessary to influence the design of compounds that will possess favorable ADME properties. Finally, in silico tools should never be used in isolation; they make up one arm of the integrated and iterative learning cycle that is comprised of in silico, in vitro, and in vivo models that we recommend using to effectively drive a drug discovery project.
MoreTranslated text
Key words
In silico ADME, Quantitative structure-property relationship models, Matched-molecular pair analysis, Predictive models, Physico-chemical properties
AI Read Science
Must-Reading Tree
Example
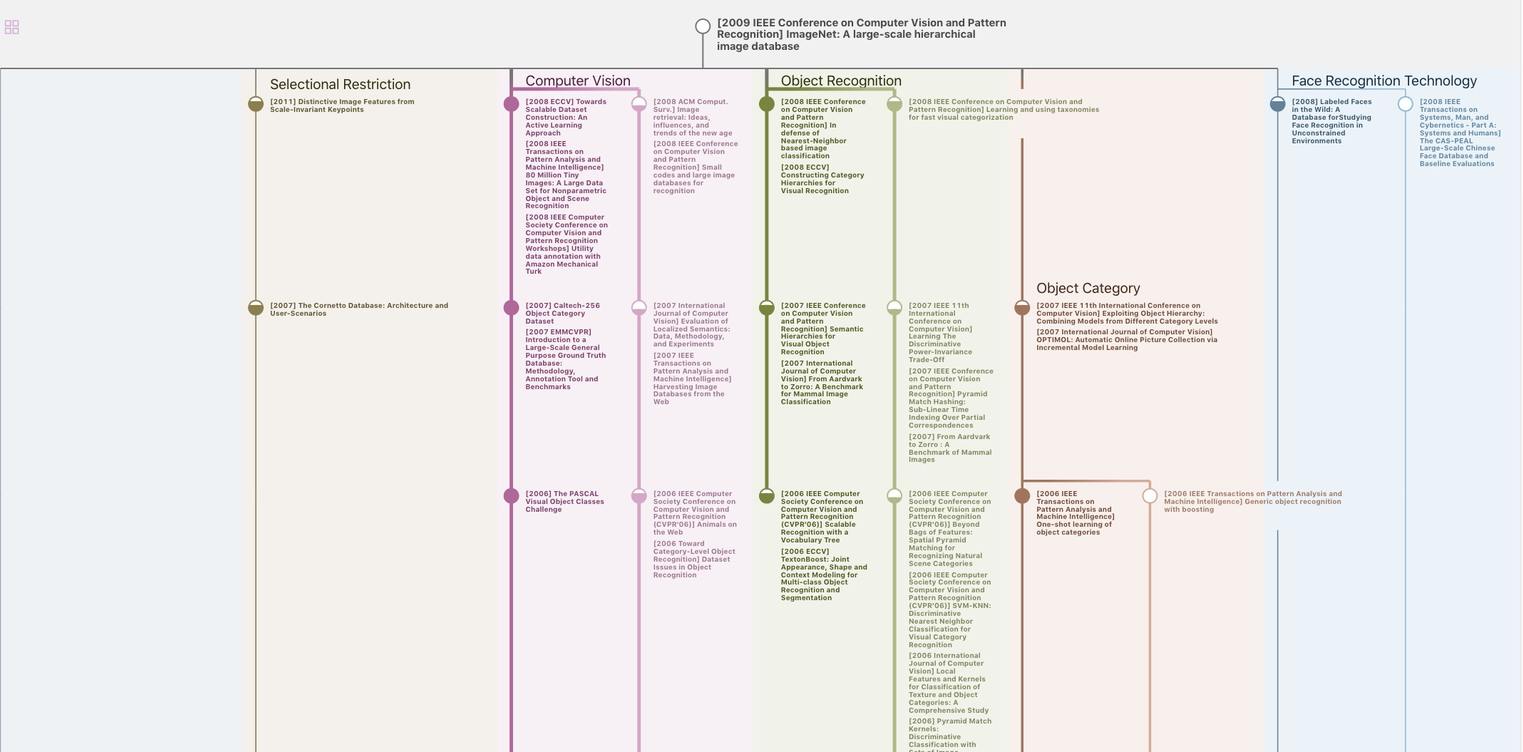
Generate MRT to find the research sequence of this paper
Chat Paper
Summary is being generated by the instructions you defined