Novel Sparse Kernel Manifold Learner for Image Classification Applications
wos(2017)
摘要
This chapter presents a sparse kernel manifold learner framework for different image classification applications. First, a new DAISY Fisher vector (D-FV) feature is created by computing Fisher vectors on densely sampled DAISY features. Second, a WLD-SIFT Fisher vector (WS-FV) feature is developed by fusing the Weber local descriptors (WLD) with the SIFT descriptors, and the Fisher vectors are computed on the fused WLD-SIFT features. Third, an innovative fused Fisher vector (FFV) feature is developed by integrating the most expressive features of the D-FV, the WS-FV and the SIFT-FV features. The FFV feature is then further assessed in eight different color spaces and a novel fused color Fisher vector (FCFV) feature is computed by integrating the PCA features of the eight color FFV descriptors. Finally, we propose a sparse kernel manifold learner (SKML) method for learning a discriminative sparse representation by considering the local manifold structure and the label information based on the marginal Fisher criterion. The objective of the SKML method is to minimize the intraclass scatter and maximize the interclass separability which are defined based on the sparse criterion. The effectiveness of the proposed SKML method is assessed on different image classification datasets such as the Painting-91 dataset for computational art painting classification, the CalTech-101 dataset for object categorization, and the 15 Scenes dataset for scene classification . Experimental results show that our proposed method is able to achieve better performance than other popular image descriptors and learning methods for different visual recognition applications.
更多查看译文
关键词
Convolutional Neural Network, Scene Category, Sift Descriptor, Fisher Vector, Weber Local Descriptor
AI 理解论文
溯源树
样例
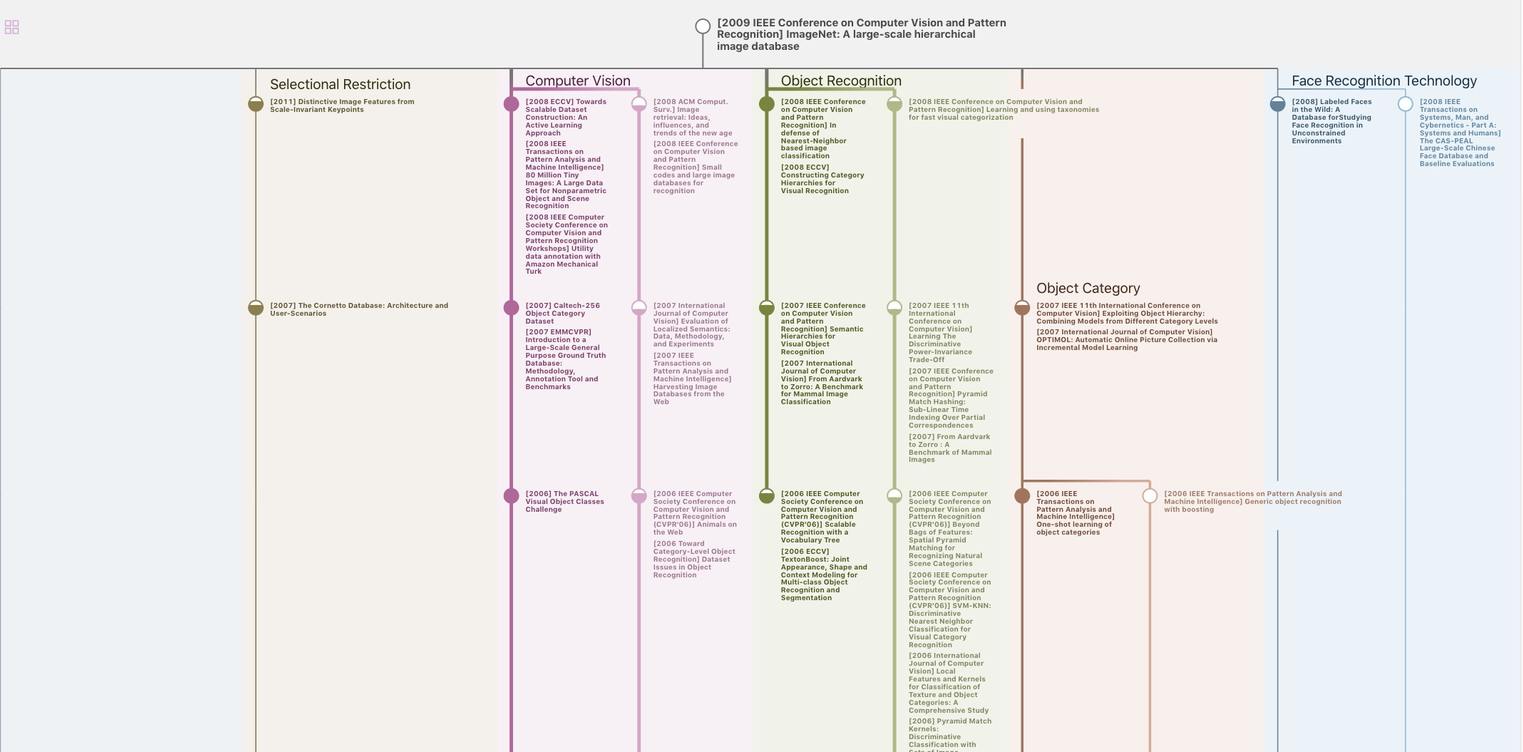
生成溯源树,研究论文发展脉络
Chat Paper
正在生成论文摘要