Image super-resolution based on structural dissimilarity learning dictionary
2017 International Conference on the Frontiers and Advances in Data Science (FADS)(2017)
摘要
Image super-resolution based on learning dictionary has recently attracted enormous interests in the field of image super-resolution. In the general, learning dictionary is trained from a large number of training samples. In this paper, we proposed to use the nonlocal self-similarity to filter blocks that are not similar in structure. Based on the judgment method of structural dissimilarity, we can get a small set of dissimilar training samples and then obtain a pair of high quality Structural Dissimilarity Learning Dictionary (SDLD). Extensive experiments on image super-resolution (SR) are carried out to validate the generality, effectiveness and robustness of the proposed method. Extensive experiments on image super-resolution are carried out to validate the generality, effectiveness and robustness of the proposed method. Experimental results demonstrate that our method can not only reduce the training time largely, but also achieve better results of SR.
更多查看译文
关键词
super-resolution,structural dissimilar dictionary,nonlocal self-similarity,sparse representation
AI 理解论文
溯源树
样例
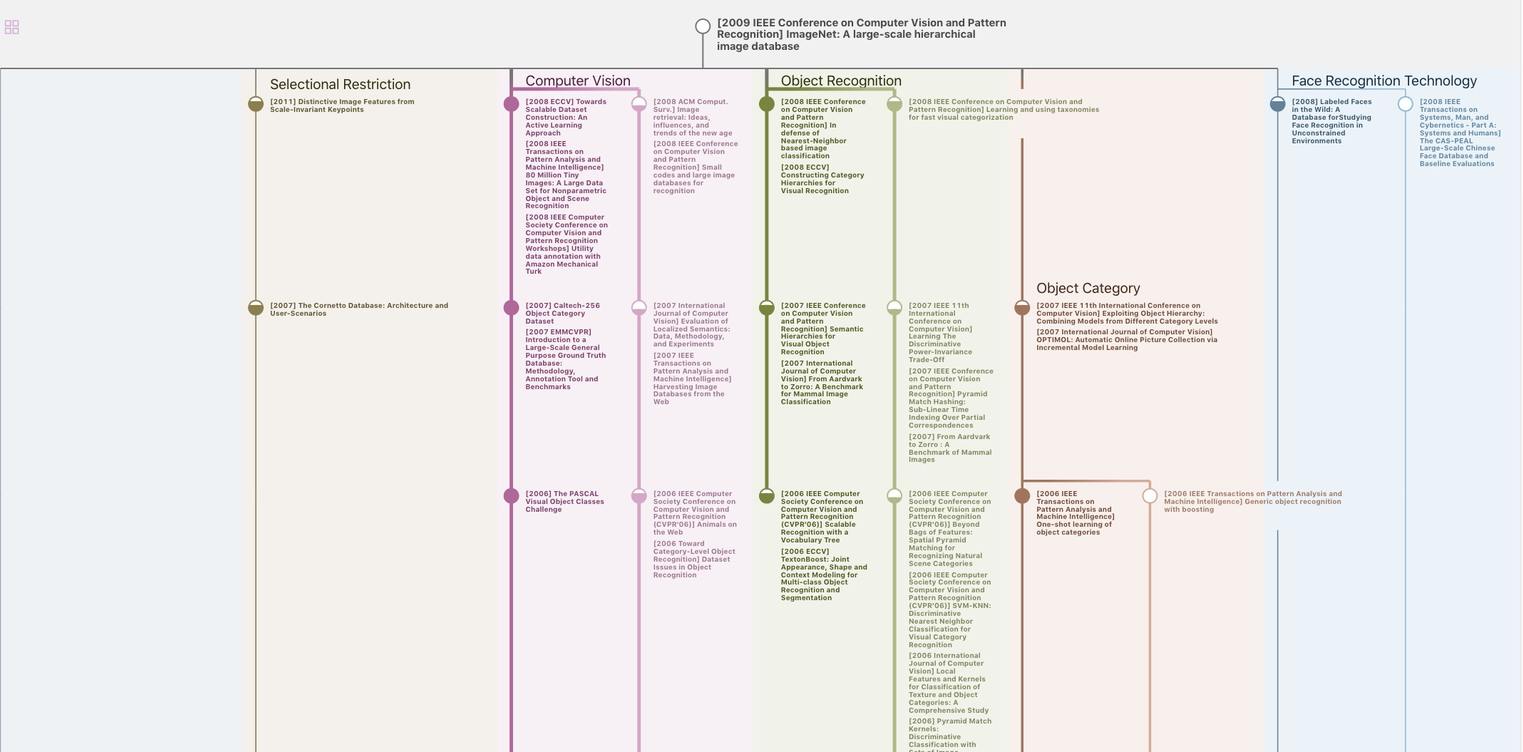
生成溯源树,研究论文发展脉络
Chat Paper
正在生成论文摘要