Optimization of K-medoids Algorithm for Initial Clustering Center
2020 4TH INTERNATIONAL CONFERENCE ON CONTROL ENGINEERING AND ARTIFICIAL INTELLIGENCE (CCEAI 2020)(2020)
摘要
This paper studies the k-medoids of the partitioning clustering algorithm. A variance-based density optimization algorithm is proposed to solve the problem of random selection of initial clustering centers, slow convergence speed and unstable clustering results in K-medoids algorithm. Based on the mean square deviation and distance mean of the sample set, the density radius of the sample set is calculated according to the size of the sample set. Under the same density radius, the samples in dense regions have high density. By dynamically selecting the samples are selected as initial clustering centers from different dense regions, in the clustering process local optimization is used to accelerate the convergence speed. These operations solve the shortcomings of K-medoids algorithm. In order to test the clustering effect, this algorithm is applied to data set of UCI machine learning. The experimental results show that the initial clustering centers selected by the algorithm are located in the dense area of the sample set, which is more in line with the original distribution of the data set. The algorithm has higher clustering accuracy, more stable clustering results and faster convergence speed on data sets.
更多查看译文
关键词
initial clustering center,algorithm,optimization,k-medoids
AI 理解论文
溯源树
样例
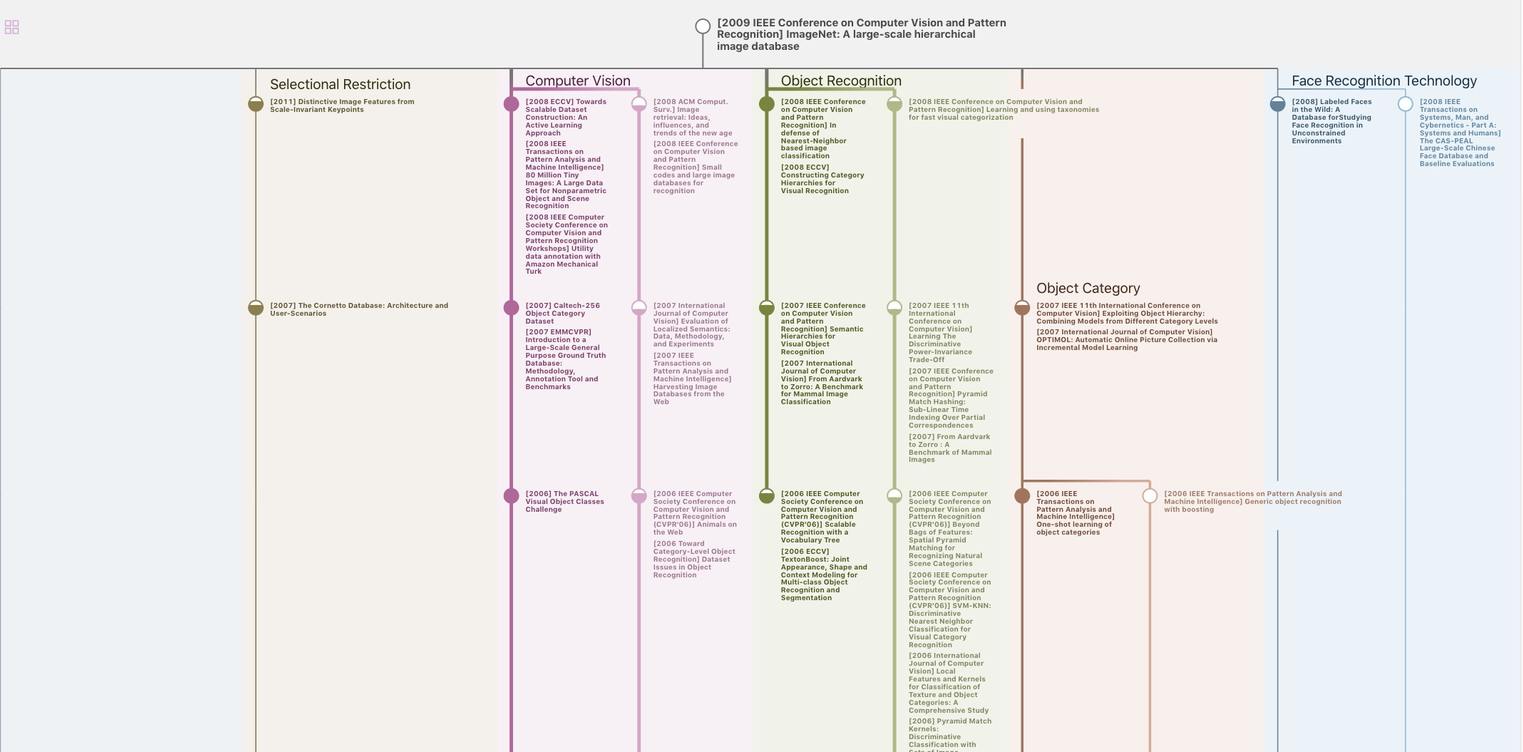
生成溯源树,研究论文发展脉络
Chat Paper
正在生成论文摘要