Hand Movement Detection From Surface Electromyography Signals By Machine Learning Techniques
VIII LATIN AMERICAN CONFERENCE ON BIOMEDICAL ENGINEERING AND XLII NATIONAL CONFERENCE ON BIOMEDICAL ENGINEERING(2020)
摘要
Surface electromyography (sEMG) signals offer information on the natural control of muscle contraction but struggle to identify temporal pattern parameters for several degrees of motion of voluntary hand movements. The complex nature of these signals renders the movement prediction task difficult; therefore, feature extraction and selection algorithms are a natural choice to transform time domain data into a new space domain to enhance recognition. The purpose of this work was to conduct an analysis of a former forearm sEMG database to improve a model to classify 15 defined hand movements. A simpler classification model was created from algorithms, such as naive Bayes (NB), linear discriminant analysis (LDA), and quadratic discriminant analysis (QDA). Also, novel preprocessing of the EMG signal data was employed and modeled the movement in virtual simulation software. In the preprocessing, outliers were eliminated, and a scatter matrix algorithm was used to transform the data into a new space to increase the differentiation between distinct classes. The processing window was 62.5 ms to generate a classification and integrate one video frame movement. Experiments yielded promising results, achieving a 93.76% recognition rate in an independent test set. The biomechanical wrist model available in OpenSim was completed by adding the missing degrees of freedom of the fingers to simulate the movement generated from the proposed classification model. The sequence of movement was converted to a biomechanical model and constructed into a video object with the potential for real time use.
更多查看译文
关键词
Electromyography, Stochastic signal, sEMG array, Scatter matrix, Probabilistic model
AI 理解论文
溯源树
样例
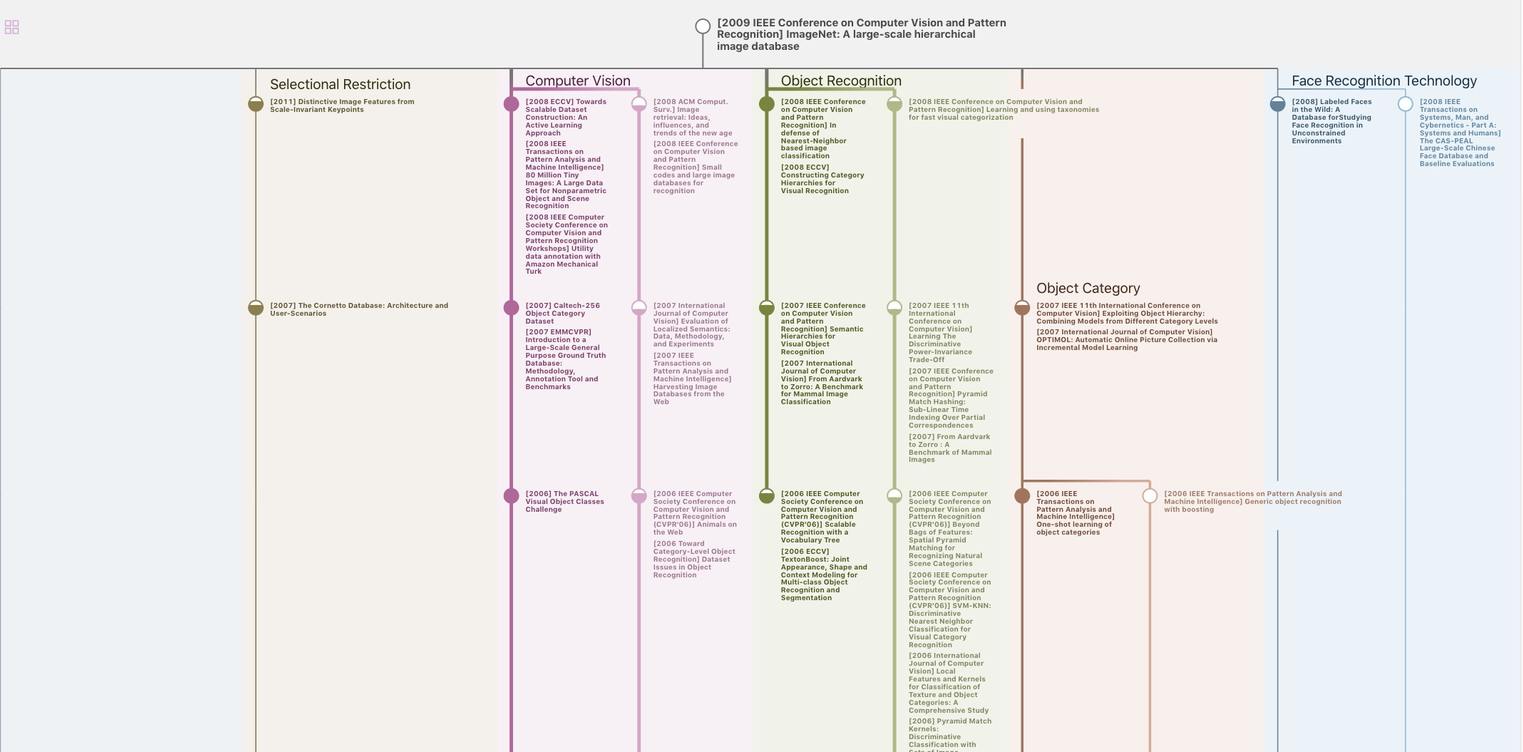
生成溯源树,研究论文发展脉络
Chat Paper
正在生成论文摘要