Inverse Modeling: A Strategy To Cope With Non-Linearity
HANDBOOK OF BIG DATA ANALYTICS(2018)
Abstract
In the big data era, discovering and modeling potentially non-linear relationships between predictors and responses might be one of the toughest challenges in modern data analysis. Most forward regression modeling procedures are seriously compromised due to the curse of dimension. In this chapter, we show that the inverse modeling idea, originated from the Sliced Inverse Regression (SIR), can help us detect nonlinear relations effectively, and survey a few recent advances, both algorithmically and theoretically, in which the inverse modeling idea leads to unforeseeable benefits in nonlinear variable selection and nonparametric screening.
MoreTranslated text
Key words
Correlation pursuit, Multiple index models, Nonparametric screening, Sliced inverse regression, Sufficient dimension reduction, Sub-Gaussian
AI Read Science
Must-Reading Tree
Example
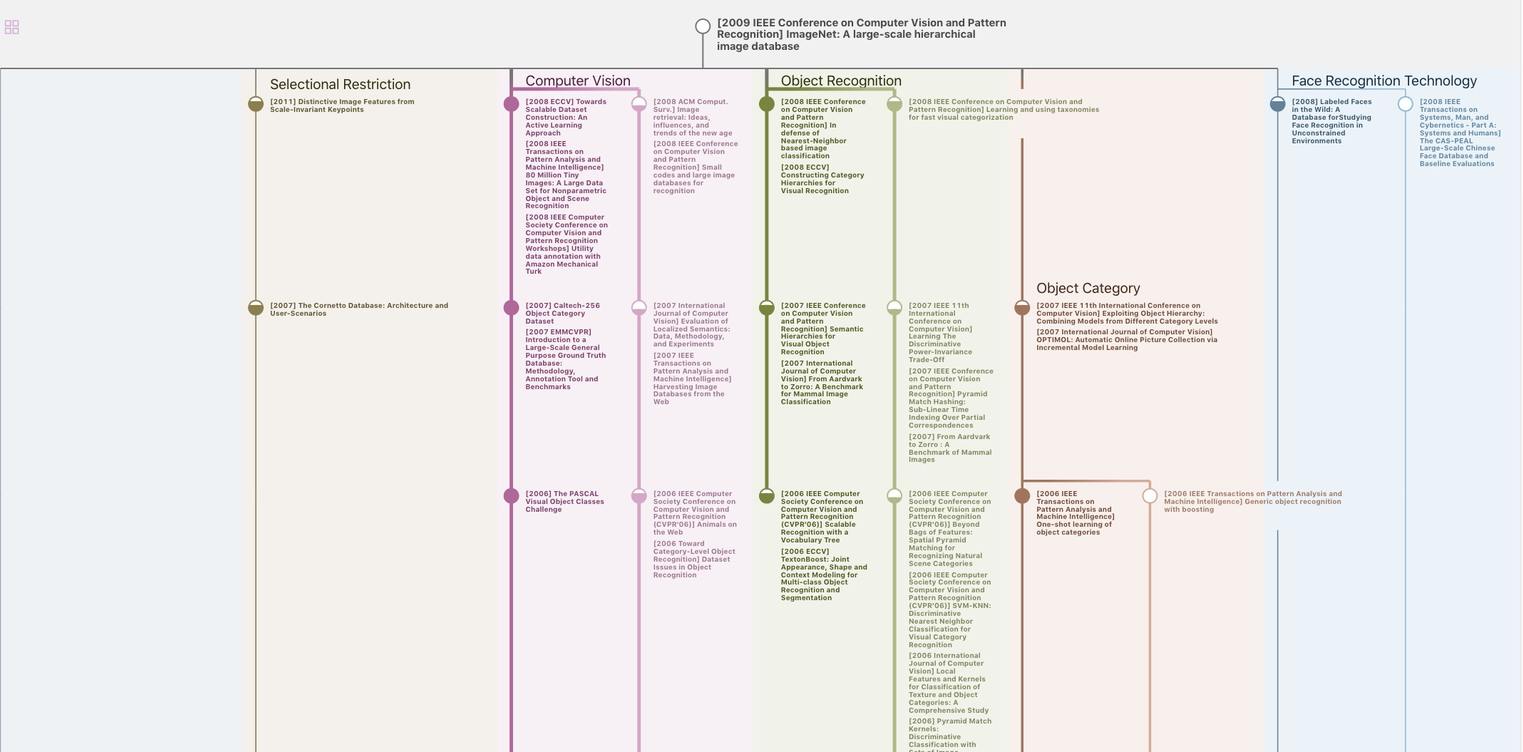
Generate MRT to find the research sequence of this paper
Chat Paper
Summary is being generated by the instructions you defined