Using Conditionally Specified Joint Distribution To Simultaneously Model Discrete And Continuous Data
STATISTICS AND APPLICATIONS(2020)
摘要
Often, in practice, conditionals are easier to model and interpret while the joint distribution itself is either intractable or not available in a closed form. Conditionally specified statistical models offer several advantages over joint models. Conditionally specified models are intuitively appealing and enrich our ability to build interpretable models in practice. In this paper, we derive the likelihood of a joint distribution obtained from Binomial and Bivariate Normal conditionals. Properties of maximum likelihood estimates and pseudolikelihood estimates are explored using a simulation. A conditionally specified model is obtained by assuming that closing prices are conditionally normally distributed and that the buy-sell recommendation by an Analyst follows a logistic regression model given the prices.
更多查看译文
关键词
Conditionally specified models, Compatibility, Maximum likelihood, Pseudolikelihood, Gibb's sampling
AI 理解论文
溯源树
样例
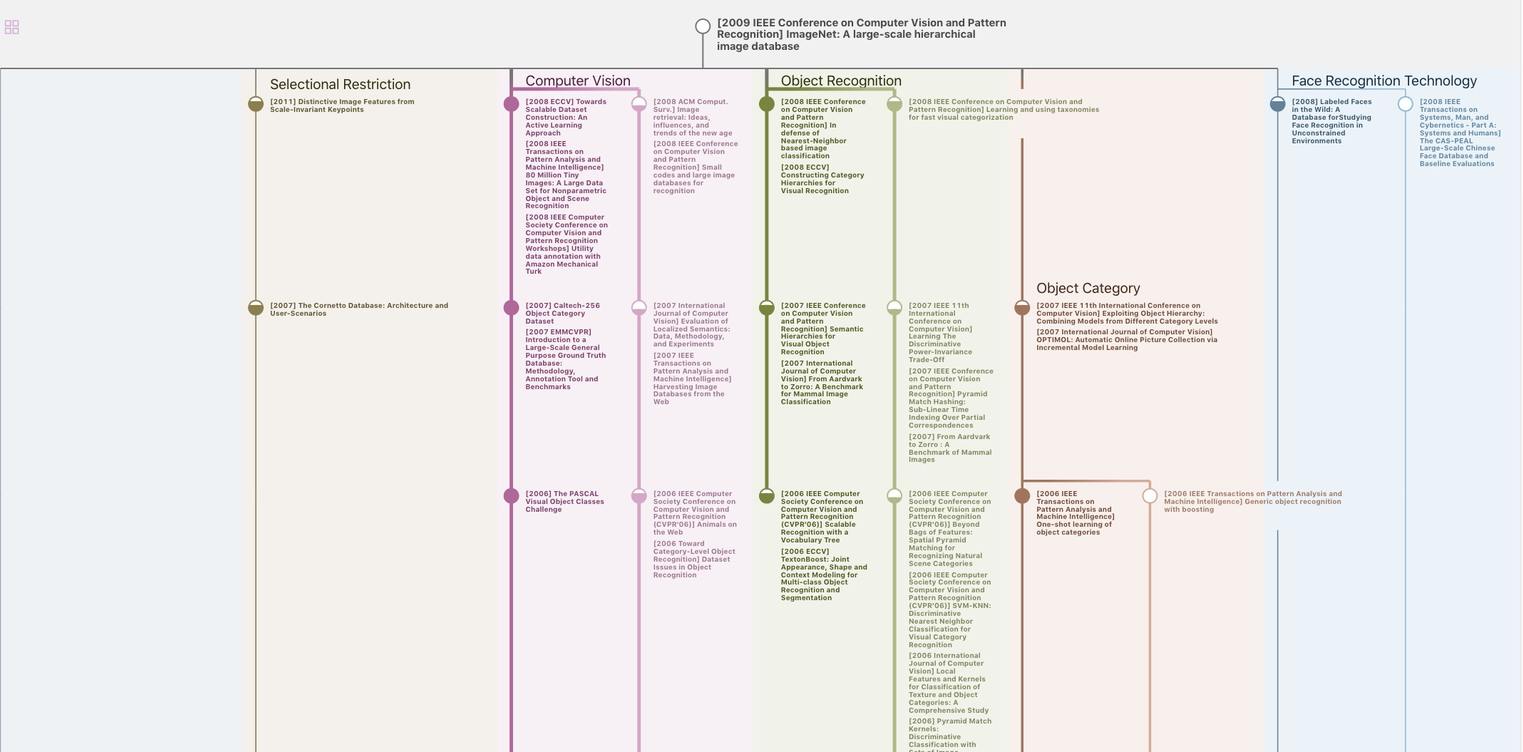
生成溯源树,研究论文发展脉络
Chat Paper
正在生成论文摘要