Coded Aperture Optimization In X-Ray Tomosynthesis Via Sparse Principal Component Analysis
ANOMALY DETECTION AND IMAGING WITH X-RAYS (ADIX) V(2020)
摘要
In this paper, a coded aperture optimization approach based on sparse principal component analysis (SPCA) is proposed to maximize the information sensed by a set of cone-beam projections. The variables in the CT system matrix correspond to observations of the attenuation characteristics of X-ray projections. An adjusted joint variance is used to update the variables and thus the overlapping information of the kth principal component is constrained by the previous k - 1 principal components. Since the coded aperture matrix is diagonal and binary, an efficient algorithm is proposed to reduce the complexity by one order of magnitude. Simulations using simulated datasets, 3D Shepp-Logan phantom, show significant gains up to 23.5dB compared with that attained by random coded apertures. Singular value decomposition (SVD) of the optimized coded apertures is used to analyze the performance of the proposed coded aperture optimization method based on SPCA.
更多查看译文
关键词
X-ray tomosynthesis, Coded aperture optimization, Sparse principal component analysis
AI 理解论文
溯源树
样例
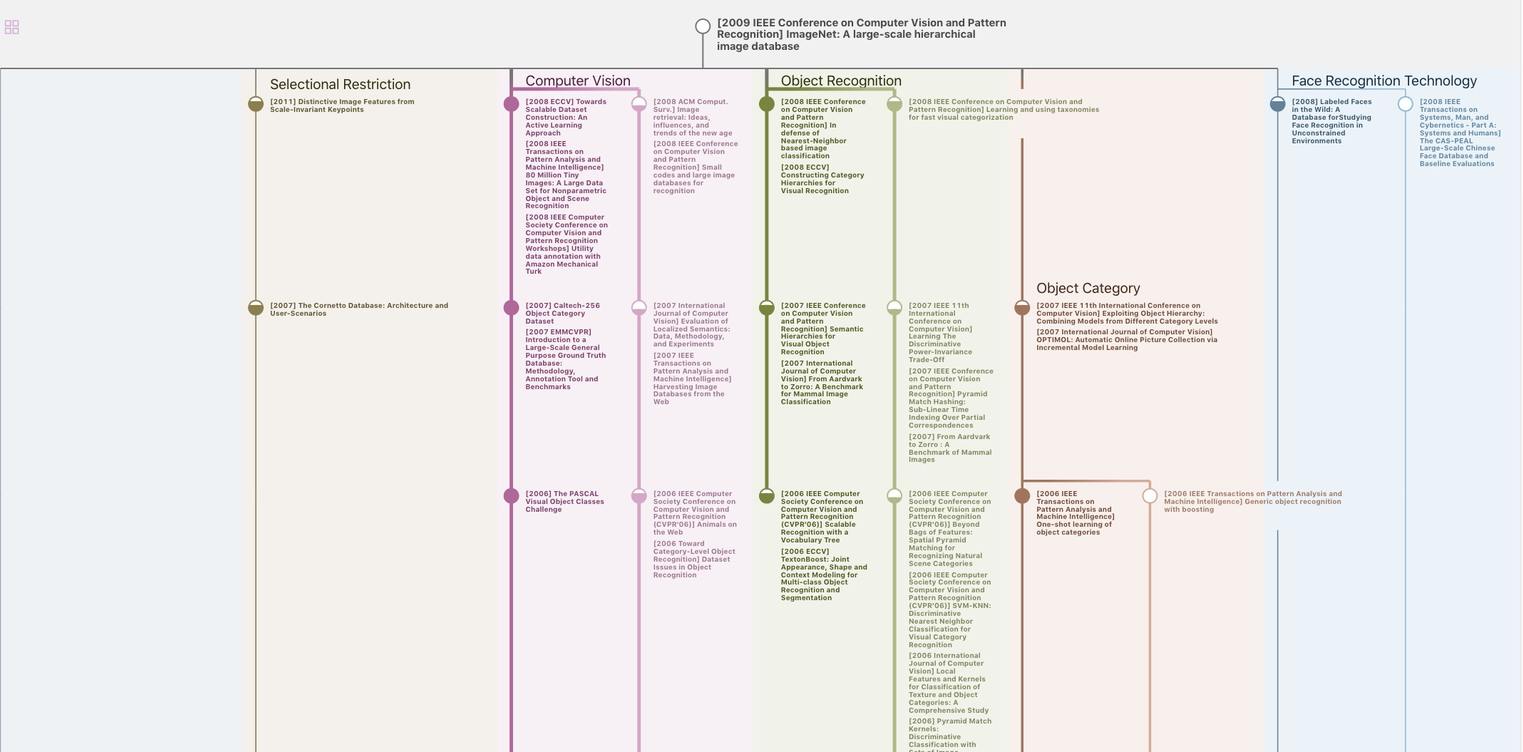
生成溯源树,研究论文发展脉络
Chat Paper
正在生成论文摘要