An Improved K-Means Clustering Algorithm Based On An Adaptive Initial Parameter Estimation Procedure For Image Segmentation
INTERNATIONAL JOURNAL OF INNOVATIVE COMPUTING INFORMATION AND CONTROL(2017)
摘要
Image segmentation is of great importance in the field of image processing. K-means clustering algorithm is widely used in image segmentation because of its computational simplicity. However, the clustering results obtained from K-means heavily depend upon the initial parameters. Mostly, these initial parameters are selected through hit and trial rule, which leads to inconsistency in the image segmentation results. In this paper, an improved K-means clustering algorithm is proposed for image segmentation, employing an adaptive histogram based initial parameter estimation procedure. Being a histogram based technique, the proposed approach is computationally inexpensive and is able to deal with the large size images effectively, which suits the real-time image segmentation scenario very well. Additionally, the proposed algorithm requires less user interaction to determine K-means initialization parameters. Some experiments are conducted based on various grey images to test the proposed approach. The experiment results show that the proposed approach can improve the K-means based image segmentation results. Finally, image segmentation results obtained through the proposed method are objectively compared with other membership based clustering algorithms such as fuzzy C-means and K-medoids. The results prove that the overall performance of the proposed K-means method is better than other membership based clustering algorithms for image segmentation.
更多查看译文
关键词
K-means clustering, Image segmentation, Fuzzy C-means, Image processing
AI 理解论文
溯源树
样例
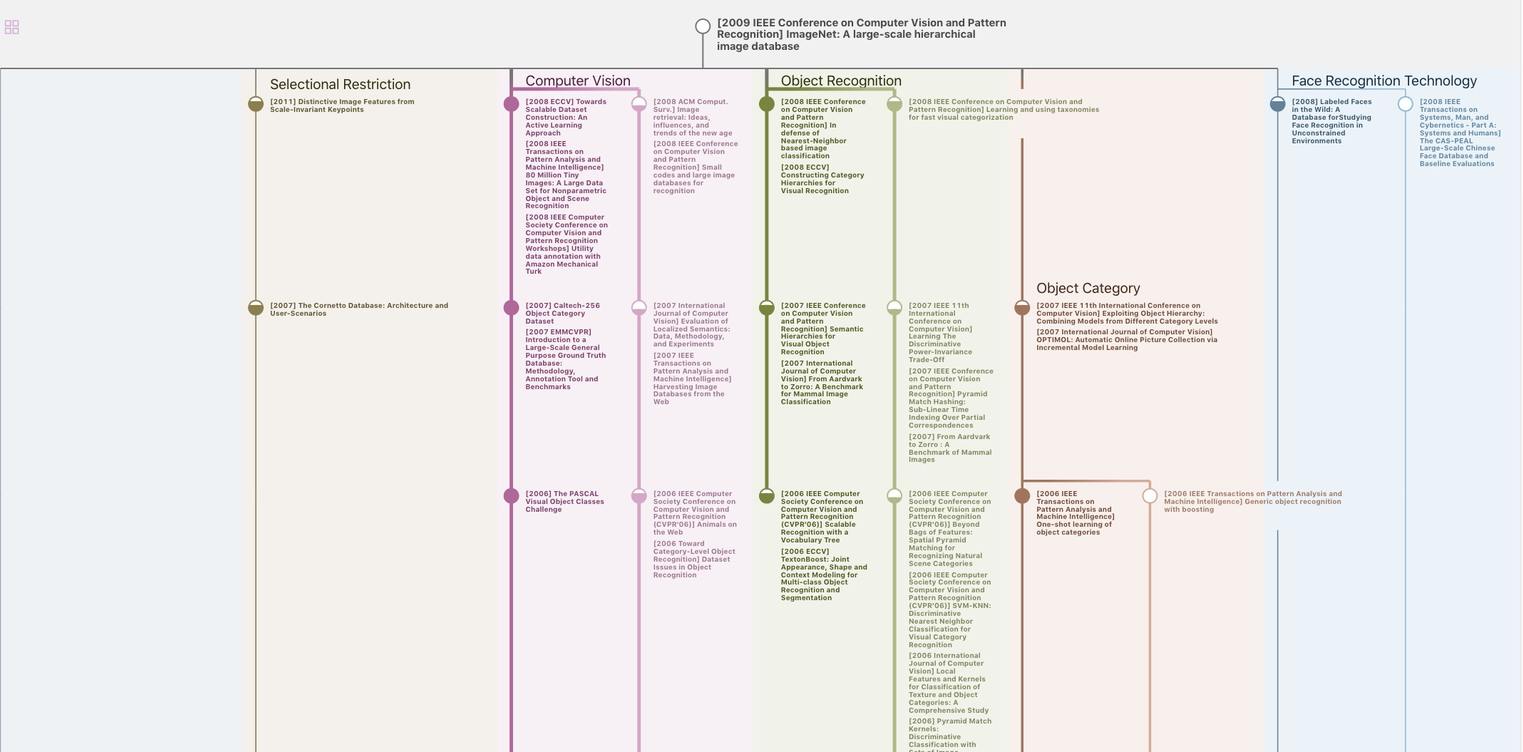
生成溯源树,研究论文发展脉络
Chat Paper
正在生成论文摘要