New Malware Detection Framework Based On N-Grams And Svdd With Smo
JOURNAL OF INFORMATION ASSURANCE AND SECURITY(2016)
摘要
Malware is a sequence of instructions that has the potential to harm any computer system or computer network. Thus, detecting malware especially new ones is a critical topic in today's software security profession. Traditional signature based detection performs well against known malicious programs but can't deal with new ones where signatures are not available. Furthermore, this approach is generally regarded as ineffective against attacks like code polymorphism and metamorphism used by malware writers to obfuscate their code. To overcome this problem new techniques have been developed using data mining and machine learning. In this paper we present a new framework to detect new malicious programs, it's based on two techniques: N-grams to extract features from suspicious files and an improved version of Support Vector Domain Description called SSPV-SVDD to classify the extracted features and detect new malware. Furthermore, we use Sequential Minimum Optimization (SMO) to solve the quadratic programming problem arising from SSPV-SVDD. We preprocessed and classified several hundred of computer viruses and clean programs to confirm the feasibility and the effectiveness of the proposed method.
更多查看译文
关键词
Information Security, Malware detection, N-grams, Support Vector Domain Description, Sequential Minimum Optimization
AI 理解论文
溯源树
样例
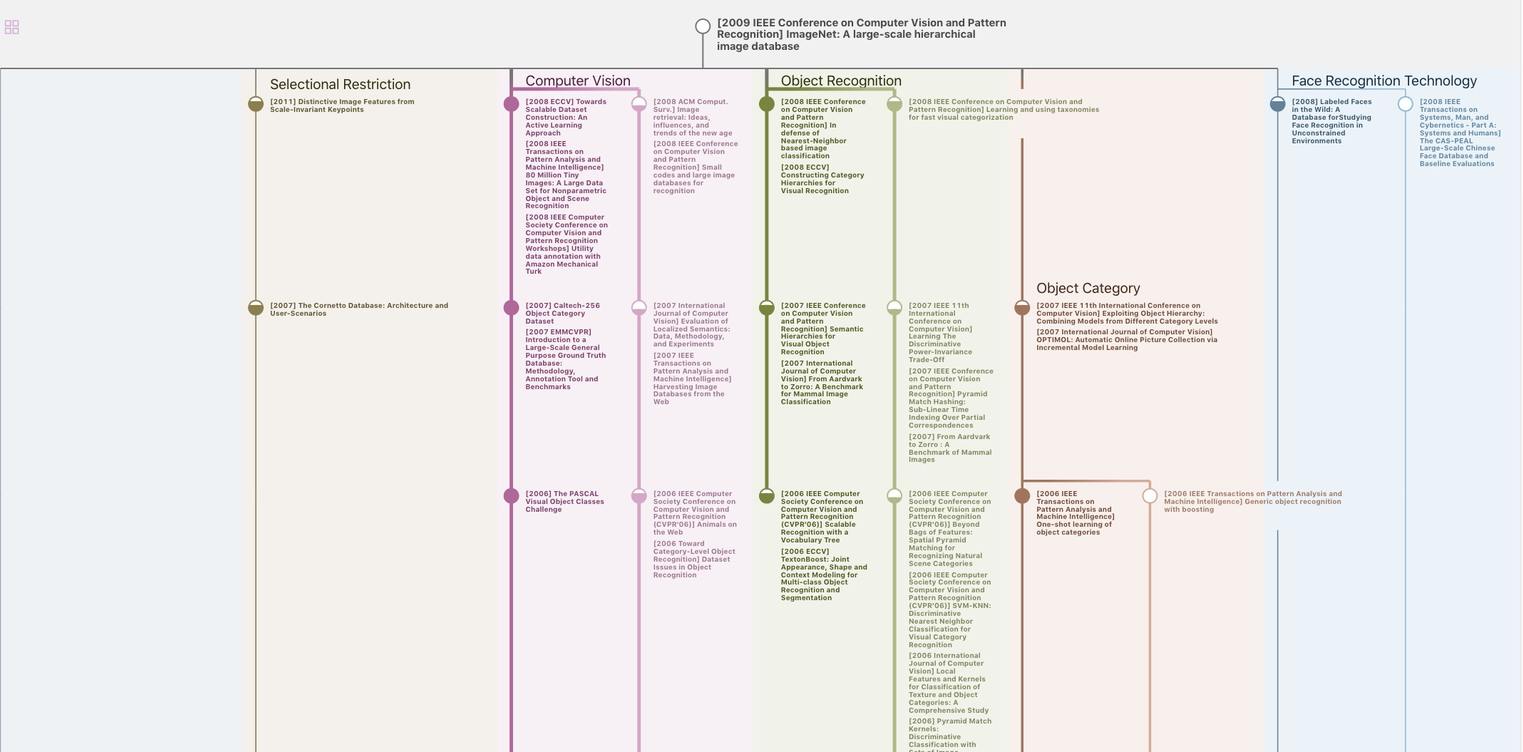
生成溯源树,研究论文发展脉络
Chat Paper
正在生成论文摘要