Quantifying The Uncertainty Of Event Detection In Full Motion Video
COUNTERTERRORISM, CRIME FIGHTING, FORENSICS, AND SURVEILLANCE TECHNOLOGIES II(2018)
摘要
Algorithms for the detection and tracking of (moving) objects can be combined into a system that automatically extracts relevant events from a large amount of video data. Such a system (data pipeline), can be particularly useful in video surveillance applications, notably to support analysts in retrieving information from hours of video while working under strict time constraints. Such data pipelines entail all sort of uncertainties, however, which can lead to erroneous detections being presented to the analyst. In this paper we present a novel method to attribute a confidence of correct detection to the output of a computer vision data pipeline. The method relies on a data-driven approach. A machine learning-based classifier is built to separate correct from erroneous detections. It is trained on features extracted from the pipeline; The features relate to both raw data properties, such as image quality, and to video content properties, such as detection characteristics. The validation of the results is done using two full motion video datasets from airborne platforms; the first being of the same type (same context) as the training set, the second being of a different type (new context). We conclude that the result of this classifier could be used to build a confidence of correct detection, separating the True Positives from the False Positives. This confidence can furthermore be used to prioritize the detections in order of reliability. This study concludes by identifying additional work measures needed to improve the robustness of the method.
更多查看译文
关键词
Uncertainty, Full Motion Video, Detection, Tracking, Machine Learning
AI 理解论文
溯源树
样例
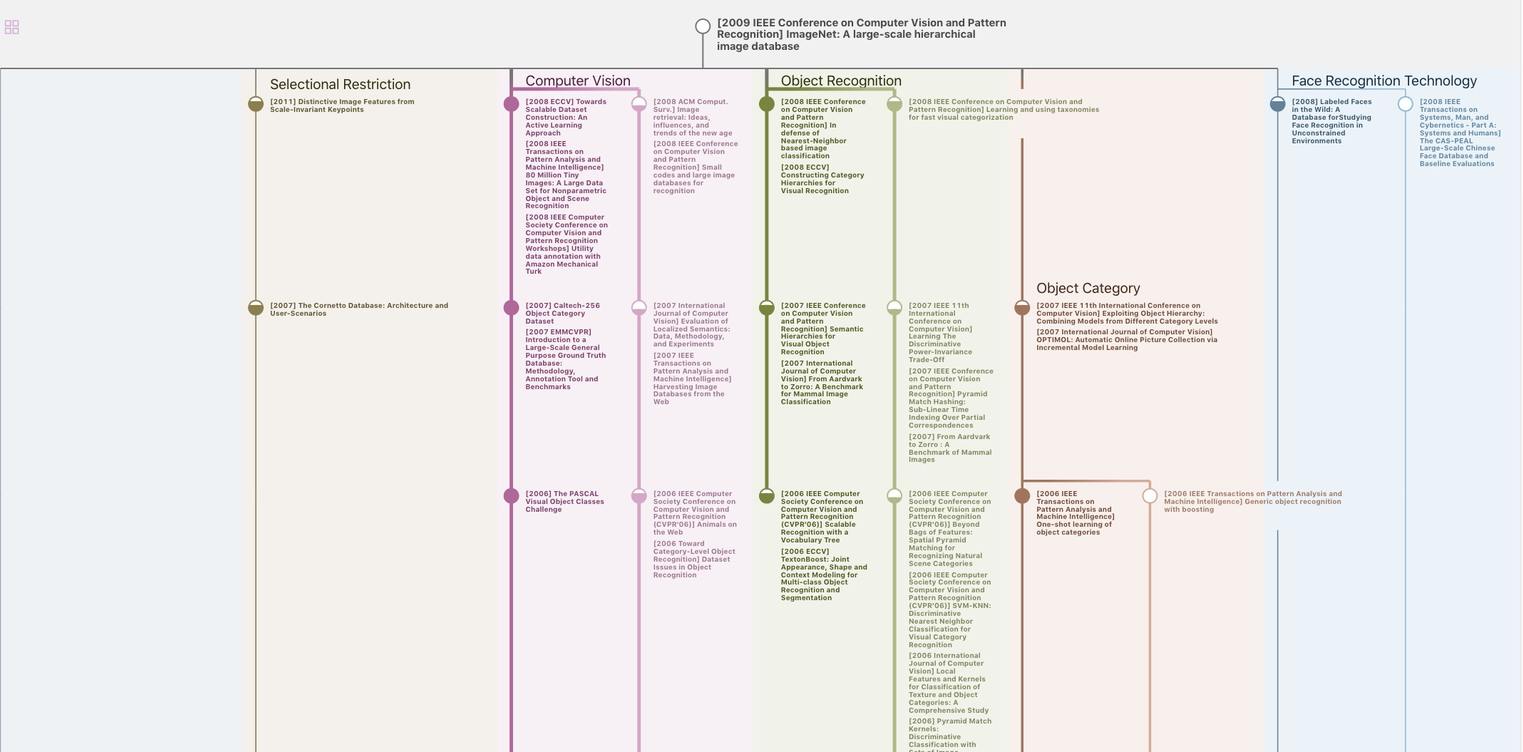
生成溯源树,研究论文发展脉络
Chat Paper
正在生成论文摘要