Matrix Capsule Network For Hyperspectral Image Classification
AOPC 2020: OPTICAL SENSING AND IMAGING TECHNOLOGY(2020)
摘要
Convolution neural network (CNN) has been know as a state-of-the-art technique for hyperspectral image (HSI) classification. However, the basic CNN architecture still has obstacles to deal with the intraclass diversity caused by spatial variability of spectral signature, especially in the case of highly limited training samples. Furthermore, they also have challenges to exploit the relationships between different features of the same object. In this paper, we propose a new network architecture based on matrix capsule network (MatCapNet) to alleviate these problems. To extract spectral-spatial features, the proposed network takes the 3-D neighboring blocks as input data. Specifically, the network consists of two conventional convolution layers, a primary matrix capsule layer, and a convolution matrix capsule layer. The proposed network uses spread loss to maximize the gap of activation values between the target class and other wrong classes. To learn more relevant instantiation parameters of the input data, a decoder network is used to reconstruct the input center spectral signature. Finally, the reconstruction loss and the spread loss are added at a certain ratio to optimize network parameters. Besides, in order to solve the problem of unbalanced sample sizes, the sample weight coefficient is utilized. The proposed approaches are carried out on two well-known HSI data sets. Compared with other state-of-the-art methods, the experiment results exhibit that the proposed network could provide a competitive advantage in terms of classification accuracy.
更多查看译文
关键词
hyperspectral image classification, matrix capsule network, spread loss, reconstruction loss
AI 理解论文
溯源树
样例
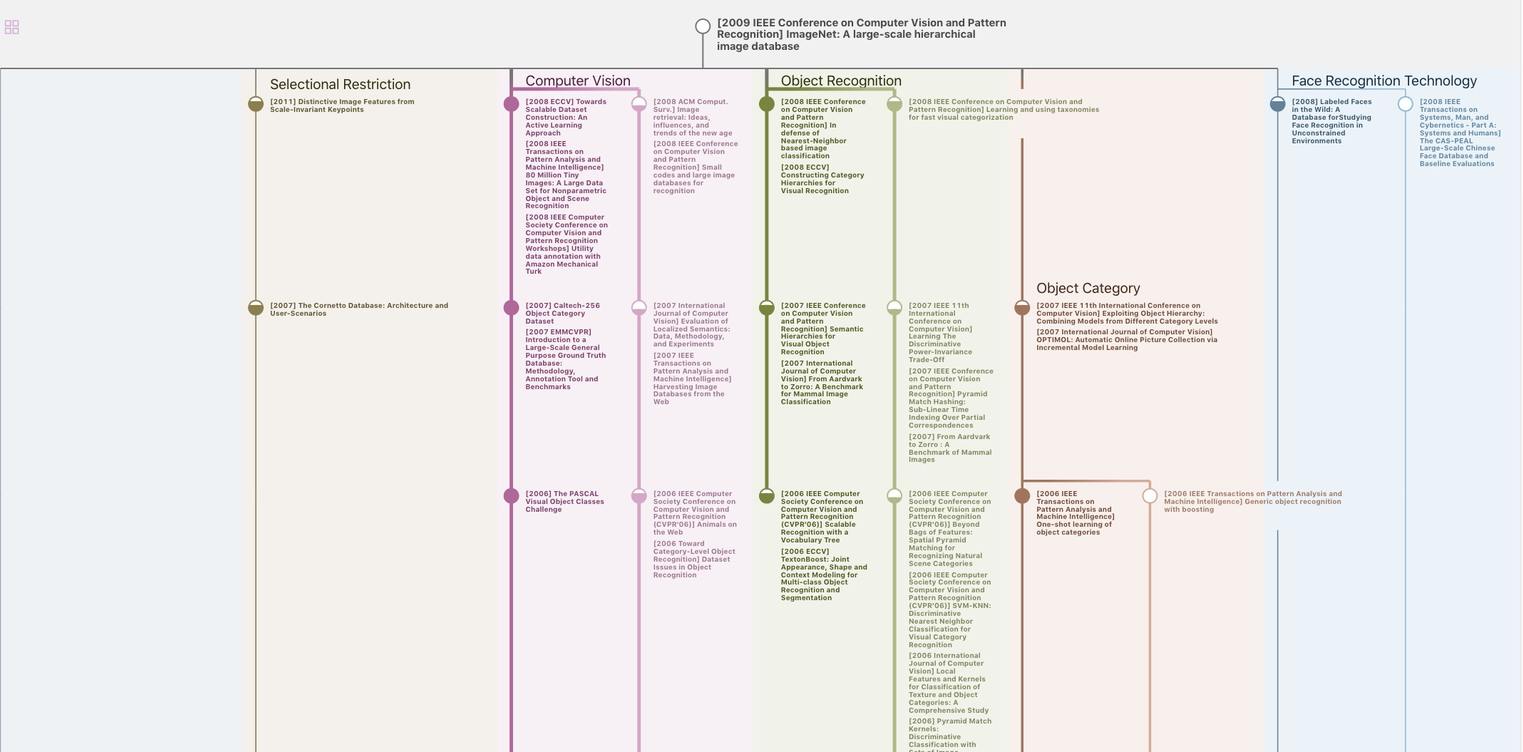
生成溯源树,研究论文发展脉络
Chat Paper
正在生成论文摘要