Stn Area Detection Using K-Nn Classifiers For Mer Recordings In Parkinson Patients During Neurostimulator Implant Surgery
20TH ARGENTINEAN BIOENGINEERING SOCIETY CONGRESS (XX ARGENTINE BIOENGINEERING CONGRESS AND IX CONFERENCE OF CLINICAL ENGINEERING), (SABI 2015)(2016)
摘要
Deep Brain Stimulation (DBS) applies electric pulses into the subthalamic nucleus (STN) improving tremor and other symptoms associated to Parkinson's disease. Accurate STN detection for proper location and implant of the stimulating electrodes is a complex task and surgeons are not always certain about final location. Signals from the STN acquired during DBS surgery are obtained with microelectrodes, having specific characteristics differing from other brain areas. Using supervised learning, a trained model based on previous microelectrode recordings (MER) can be obtained, being able to successfully classify the STN area for new MER signals The K Nearest Neighbours (K-NN) algorithm has been successfully applied to STN detection. However, the use of the fuzzy form of the K-NN algorithm (KNN-F) has not been reported. This work compares the STN detection algorithm of K-NN and KNN-F. Real MER recordings from eight patients where previously classified by neurophysiologists, defining 15 features. Sensitivity and specificity for the classifiers are obtained, Wilcoxon signed rank non-parametric test is used as statistical hypothesis validation. We conclude that the performance of KNN-F classifier is higher than K-NN with p<0.01 in STN specificity.
更多查看译文
关键词
parkinson patients
AI 理解论文
溯源树
样例
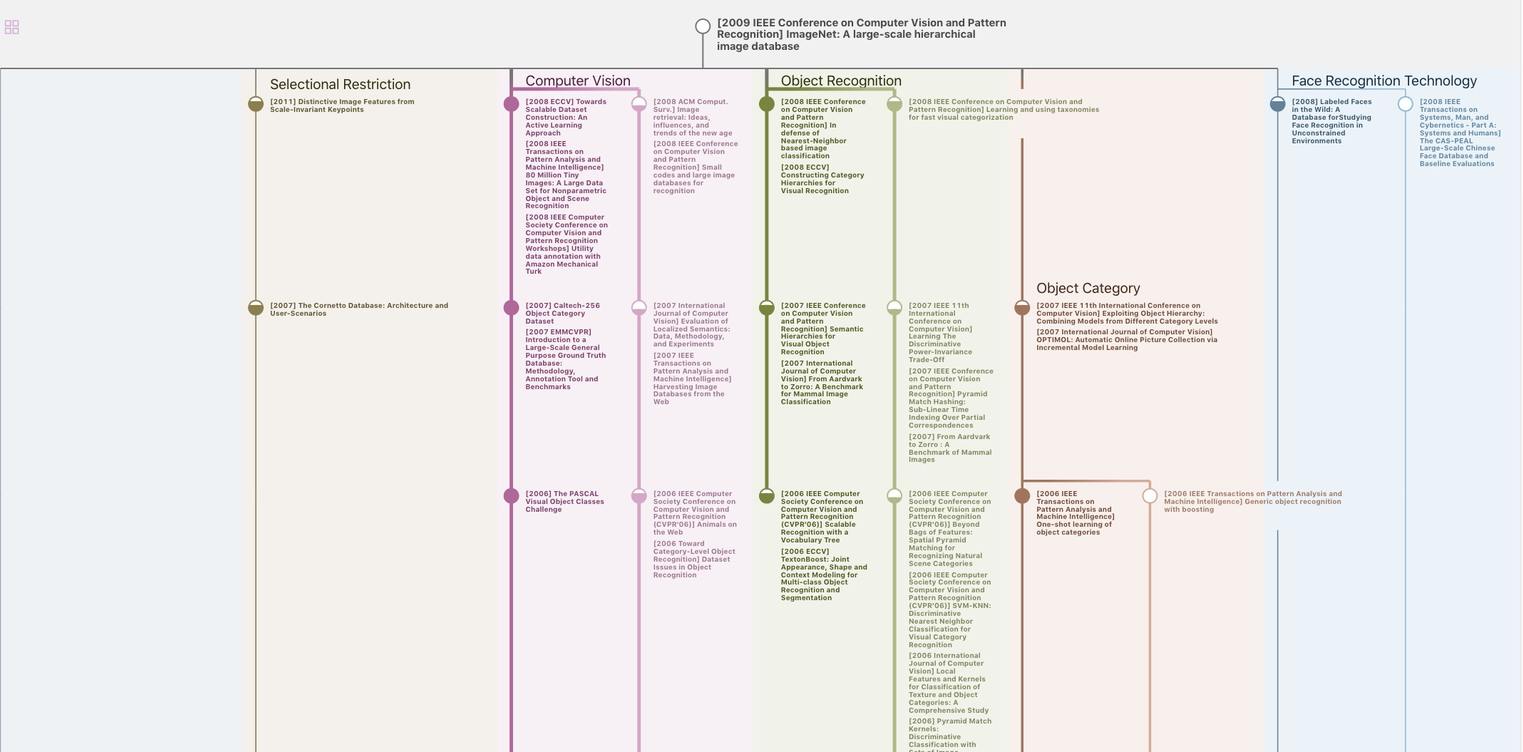
生成溯源树,研究论文发展脉络
Chat Paper
正在生成论文摘要