Electrocorticographic Signals Classification For Brain Computer Interfaces Using Stacked-Autoencoders
APPLICATIONS OF MACHINE LEARNING 2020(2020)
摘要
Neuro-degenerative diseases can break brain's common output pathways of peripheral nerves and muscles in an individual, inhibiting his ability to perform daily tasks. Brain Computer Interfaces BCI make decoding-encoding of brain signals into control instructions for external devices. This work proposes the use of stacked autoencoders and a softmax layer for classification of visual stimuli from Electrocorticographic (ECoG) signals as an input to the BCI control system. Experimental results show that the proposed method has a good classification performance (average accuracy across subjects 0.95 +/- 0.05), compared to state-of-the-art approaches as Support Vector Machines SVM. Furthermore, the proposed network architecture allows analysis of the weights learned by the classifier making it possible to obtain insights of what signal features the classifier uses to discriminate the visual stimulus.
更多查看译文
关键词
BCI, Electrocorticographic ECoG, brain signal classification, stacked autoencoders, machine learning
AI 理解论文
溯源树
样例
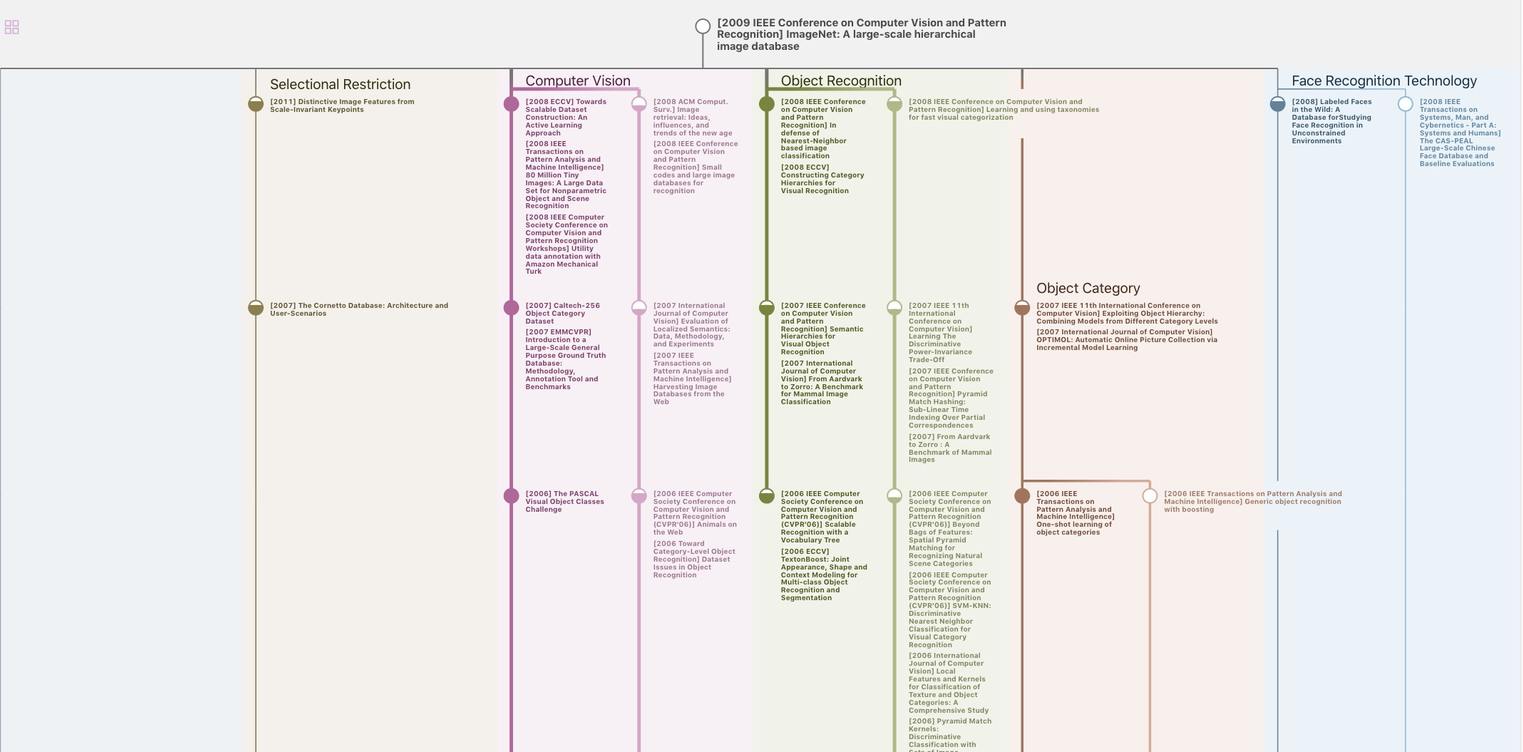
生成溯源树,研究论文发展脉络
Chat Paper
正在生成论文摘要