Recognition Of Heavy Metal Contamination Using Tegillarca Granosa Libs Data
4TH INTERNATIONAL CONFERENCE ON ADVANCES IN ENERGY RESOURCES AND ENVIRONMENT ENGINEERING(2019)
摘要
The problem of heavy metal pollution in food and the environment has drawn increasing public concern, because of its irreversible damage. Traditional methods of detecting heavy metals require complicated laboratory conditions and skilled experimenters, and cannot meet cost-effectiveness, rapidity and ease of use. In this paper, we propose a principal component analysis and linear discriminant analysis (PCA-LDA) pattern recognition algorithm for rapid detection of heavy metals in tegillarca granosa by using laser-induced breakdown spectroscopy (LIBS) data. First, the principal component analysis (PCA) algorithm is used to reduce the dimension of spectral data from the previous 2811 dimension to 16 dimensions. Second, the linear discriminant analysis (LDA) algorithm is used for classification. Results show that PCA-LDA has the best classification accuracy compared with the partial least squares discriminant analysis (PLS-DA), soft independent modeling of class analogy (SIMCA) and support vector machine (SVM) algorithms, and achieves a discrimination accuracy of 87%. This work indicates that by combining pattern recognition with LIBS technology, healthy tegillarca granosa can be quickly distinguished from heavy metal polluted samples, which offers possibility of environmental monitoring and in field food detection.
更多查看译文
关键词
tegillarca granosa libs data,heavy metal contamination
AI 理解论文
溯源树
样例
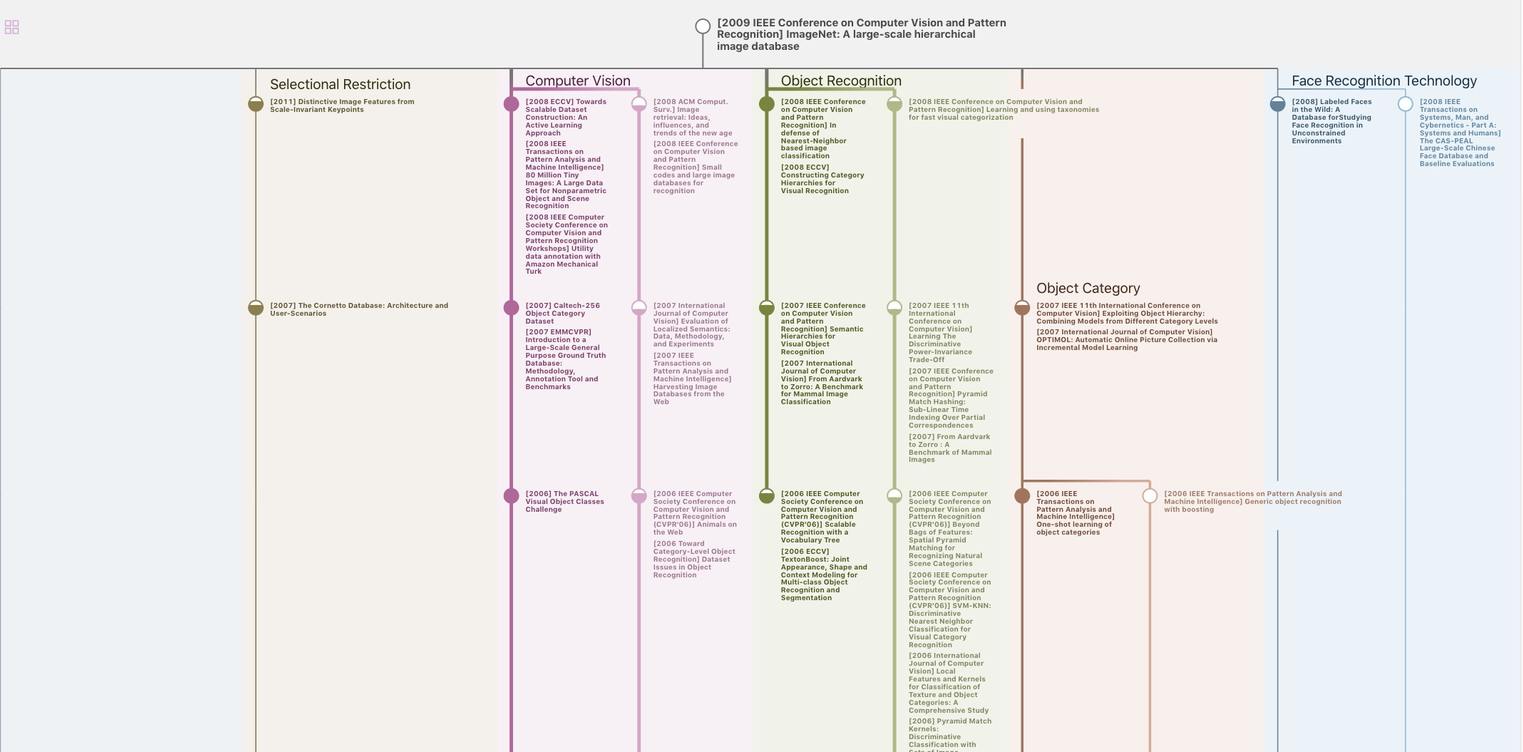
生成溯源树,研究论文发展脉络
Chat Paper
正在生成论文摘要