Automated Surface Inspection Using 3d Point Cloud Data In Manufacturing - A Case Study
PROCEEDINGS OF THE ASME 13TH INTERNATIONAL MANUFACTURING SCIENCE AND ENGINEERING CONFERENCE, 2018, VOL 3(2018)
摘要
With the latest advancements in three-dimensional (3D) measurement technologies, obtaining 3D point cloud data for inspection purposes in manufacturing is becoming more common. While 3D point cloud data allows for better inspection capabilities, their analysis is typically challenging. Especially with unstructured 3D point cloud data, containing coordinates at random locations, the challenges increase with higher levels of noise and larger volumes of data. Hence, the objective of this paper is to extend the previously developed Adaptive Generalized Likelihood Ratio (AGLR) approach to handle unstructured 3D point cloud data used for automated surface defect inspection in manufacturing. More specifically, the AGLR approach was implemented in a practical case study to inspect twenty-seven samples, each with a unique fault. These faults were designed to cover an array of possible faults having three different sizes, three different magnitudes, and located in three different locations. The results show that the AGLR approach can indeed differentiate between non-faulty and a varying range of faulty surfaces while being able to pinpoint the fault location. This work also serves as a validation for the previously developed AGLR approach in a practical scenario.
更多查看译文
关键词
3d point cloud data,surface,cloud data
AI 理解论文
溯源树
样例
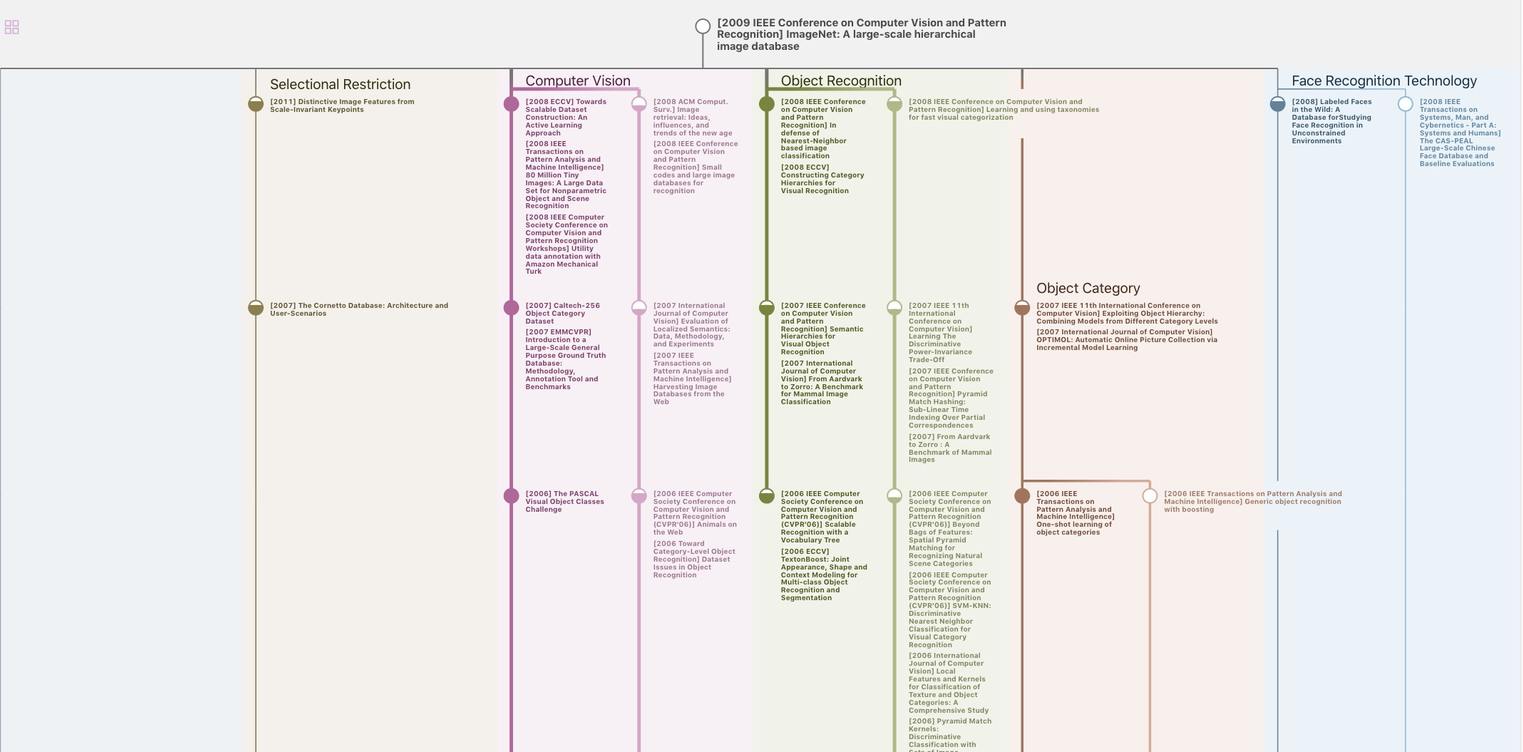
生成溯源树,研究论文发展脉络
Chat Paper
正在生成论文摘要