Learning EEG: Identification of novel electroencephalogram classifications and variability of baseline features in a large clinical dataset
2017 INTERNATIONAL SYMPOSIUM ON WEARABLE ROBOTICS AND REHABILITATION (WEROB)(2017)
摘要
Despite its increasing use and public health importance, very little is known about the consistency and variability of baseline electroencephalogram (EEG) measurements in healthy individuals and in patient populations. This research aims to investigate population variability of EEG features and their ability to classify patients based on patient characteristics. We first propose a data-driven method of evaluating consistency and variability of commonly used EEG features. Using a Kruskal-Wallis test we find normalized features compared across different time intervals within a given recording to be consistent. Further, we find certain features to have better intra-subject consistency than others, notably spectral entropy, skew, and kurtosis. Using these results we apply machine learning techniques to classify patients based on sex, age, medications taken, and clinical impressions. We find statistically significant classifications for age, medications taken, and clinical impressions, achieving accuracies above 86%, 72%, and 74%, respectively.
更多查看译文
关键词
electroencephalogram classifications,baseline electroencephalogram measurements,intra-subject consistency,Kruskal-Wallis test,EEG features,data-driven method
AI 理解论文
溯源树
样例
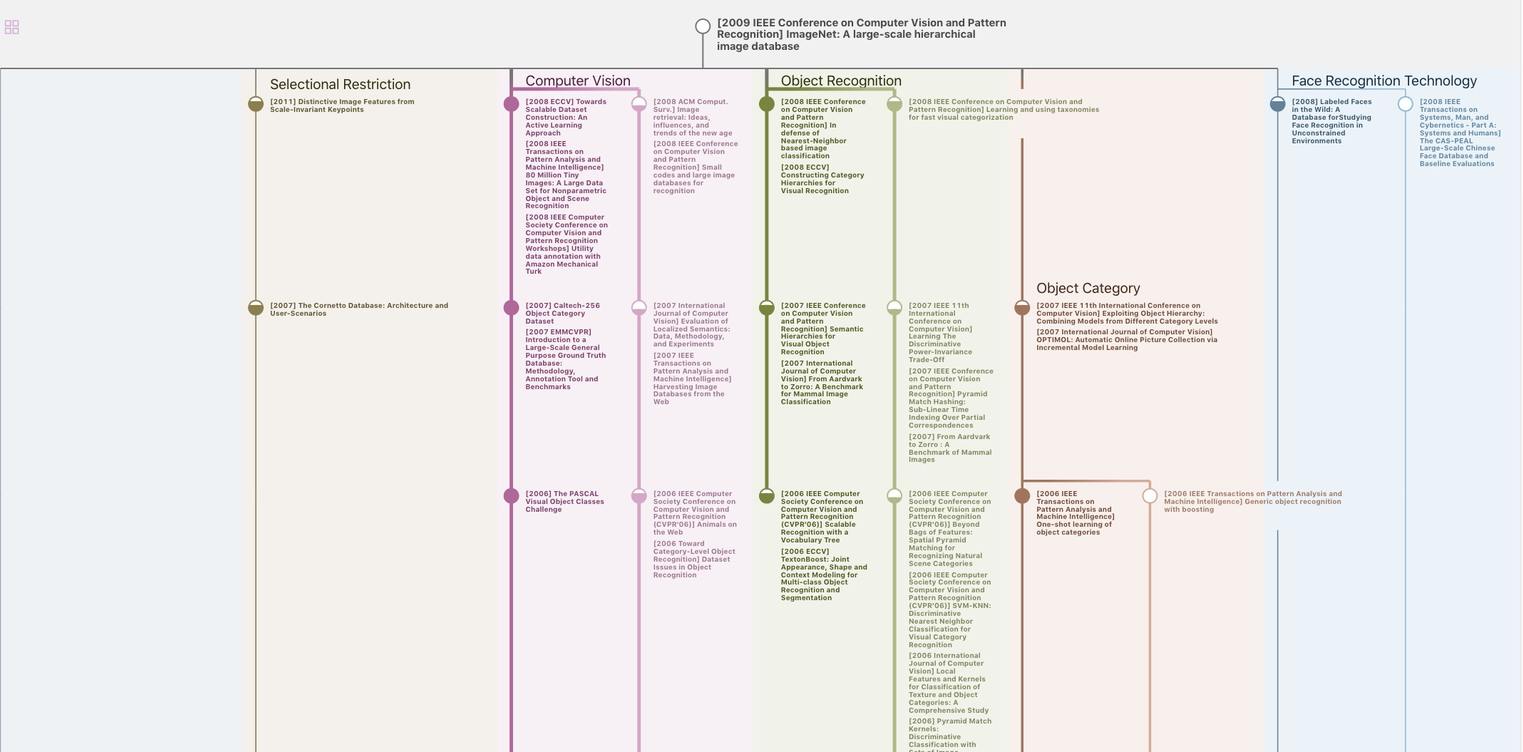
生成溯源树,研究论文发展脉络
Chat Paper
正在生成论文摘要