Learning 6D Pose of Textureless Objects Via Multi-Scale Dense Relation
2020 INTERNATIONAL CONFERENCE ON IMAGE, VIDEO PROCESSING AND ARTIFICIAL INTELLIGENCE(2020)
摘要
6D object pose estimation is a fundamental problem for many computer vision and robotics applications. Recent work has shown that data-driven approaches could enable accurate 6D pose estimation for objects with sufficient texture on the surface. However, few works have focused on estimation 6D pose for texture-less objects. In this paper, we present a network that estimating 6D pose for texture-less objects by using the multi-scale relational features. The proposed network, which leverages both the appearance and geometry features from multi-scale point groups, is able to extract distinctive features for texture-less region. In particular, the multi-scale features encode relational information of the point groups, are more informative compared to the feature comes from vanilla convolutional neural networks and PointNet. The proposed network is end-to-end trainable. Experiments on T-LESS dataset demonstrate our method achieves competitive results on 6D pose estimation task of texture-less objects.
更多查看译文
关键词
6D pose estimation,texture-less objects,deep learning,relational networks
AI 理解论文
溯源树
样例
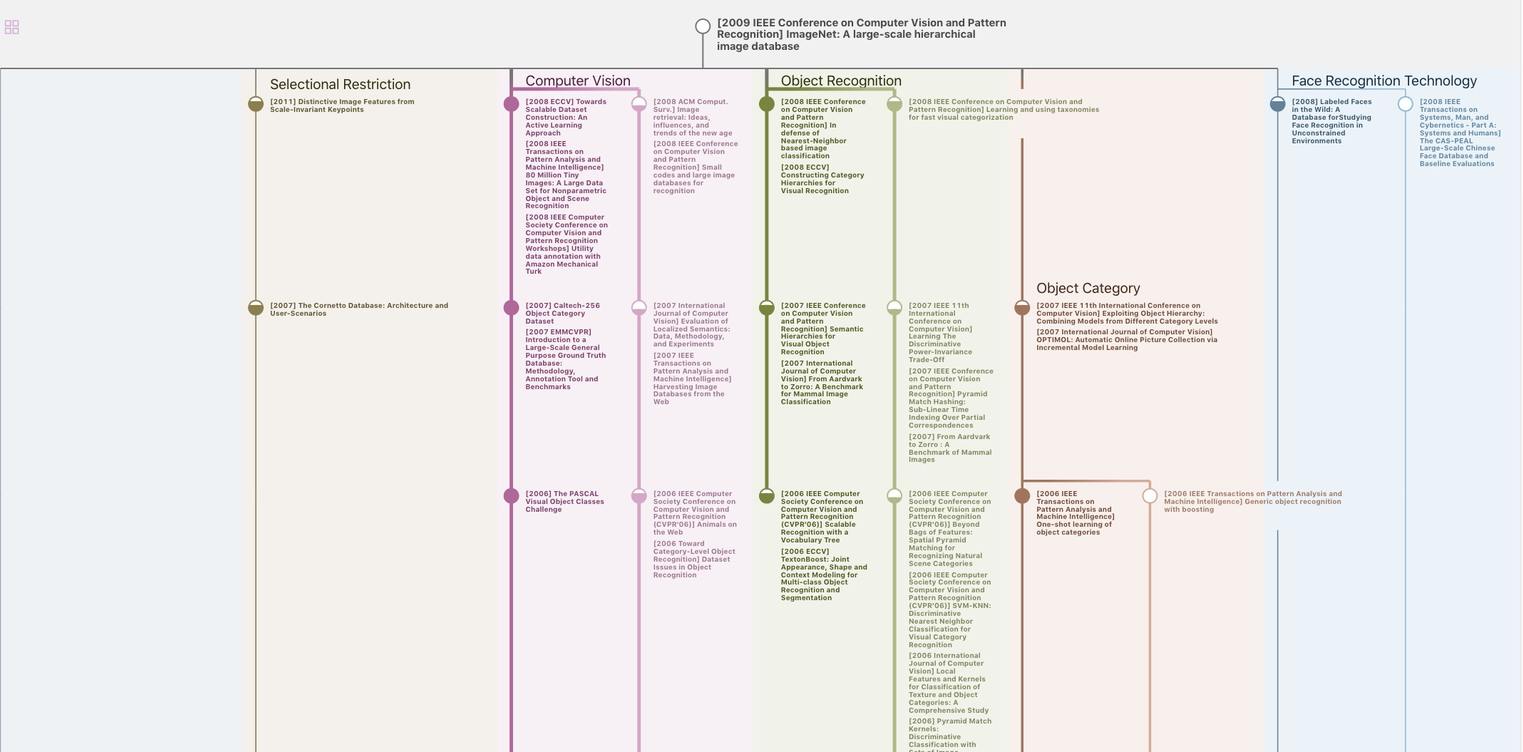
生成溯源树,研究论文发展脉络
Chat Paper
正在生成论文摘要