Wavelet Transform-Statistical Time Features-Based Methodology For Epileptic Seizure Prediction Using Electrocardiogram Signals
MATHEMATICS(2020)
摘要
Epilepsy is a brain disorder that affects about 50 million persons around the world and is characterized by generating recurrent seizures, which can put patients in permanent because of falls, drowning, burns, and prolonged seizures that they can suffer. Hence, it is of vital importance to propose a methodology with the capability of predicting a seizure with several minutes before the onset, allowing that the patients take their precautions against injuries. In this regard, a methodology based on the wavelet packet transform (WPT), statistical time features (STFs), and a decision tree classifier (DTC) for predicting an epileptic seizure using electrocardiogram (ECG) signals is presented. Seventeen STFs were analyzed to measure changes in the properties of ECG signals and find characteristics capable of differentiating between healthy and 15 min prior to seizure signals. The effectiveness of the proposed methodology for predicting an epileptic event is demonstrated using a database of seven patients with 10 epileptic seizures, which was provided by the Massachusetts Institute of Technology-Beth Israel Hospital (MIT-BIH). The results show that the proposed methodology is capable of predicting an epileptic seizure 15 min before with an accuracy of 100%. Our results suggest that the use of STFs at frequency bands related to heart activity to find parameters for the prediction of epileptic seizures is suitable.
更多查看译文
关键词
seizure prediction, ECG signals, wavelet transform, statistical time features
AI 理解论文
溯源树
样例
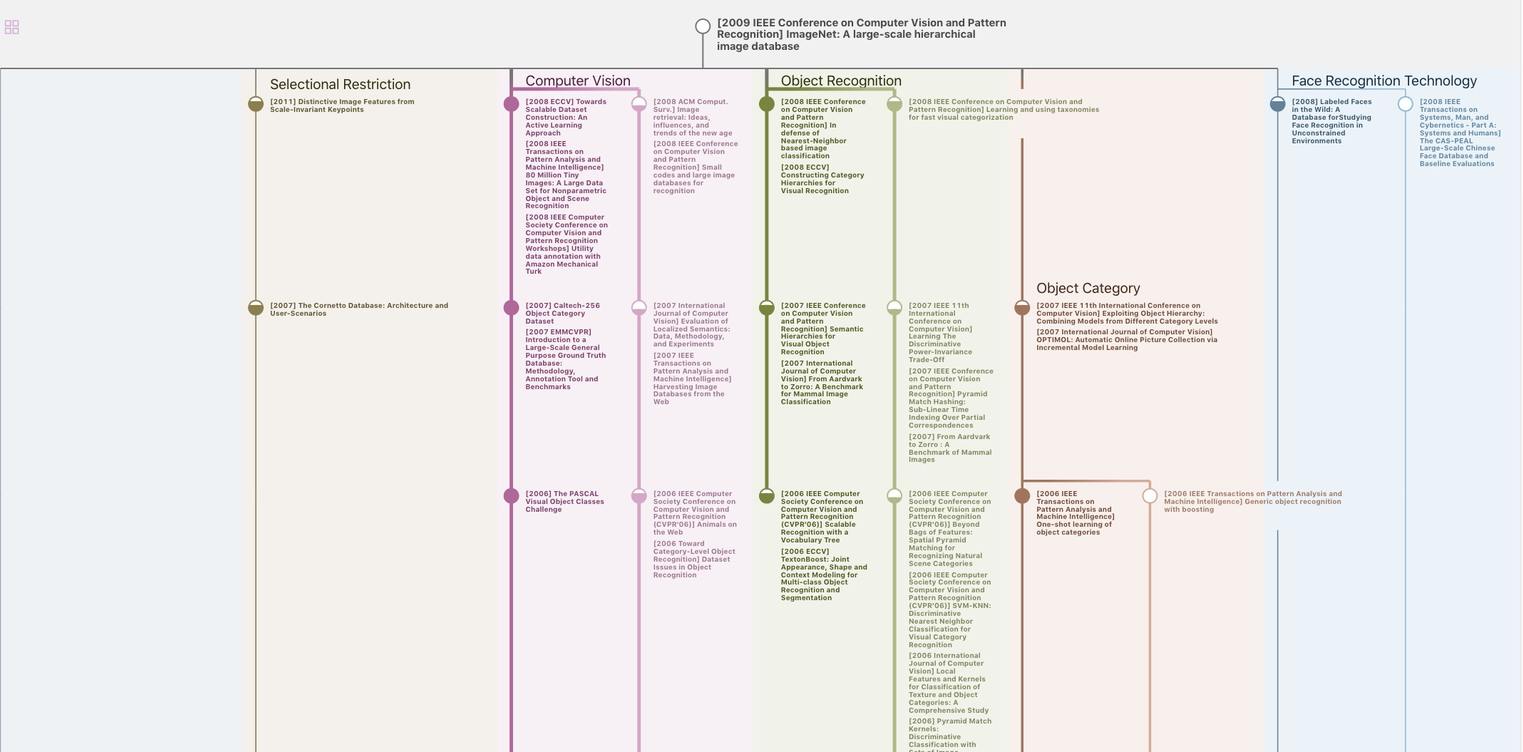
生成溯源树,研究论文发展脉络
Chat Paper
正在生成论文摘要