Nested Sampling Strategy For Bayesian Design Space Characterization
30TH EUROPEAN SYMPOSIUM ON COMPUTER AIDED PROCESS ENGINEERING, PTS A-C(2020)
Abstract
Design space is a key concept in pharmaceutical quality by design, providing better understanding of manufacturing processes and enhancing regulatory flexibility. It is of paramount importance to develop computational techniques for providing quantitative representations of a design space, in accordance with the ICH Q8 guideline. The focus is on Bayesian approaches to design space characterization, which rely on a process model to determine a feasibility probability that is used for measuring reliability and risk. The paper presents three improvements over an existing nested sampling method: two-phase strategy with the first phase using a cheap sorting function based on nominal model parameters; dynamic sampling strategy to refine the target design space; and vectorization to evaluate costly functions in parallel. These improvements are implemented as part of the python package DEUS and demonstrated on an industrial case study.
MoreTranslated text
Key words
pharmaceutical processes, quality-by-design, design space, nested sampling
AI Read Science
Must-Reading Tree
Example
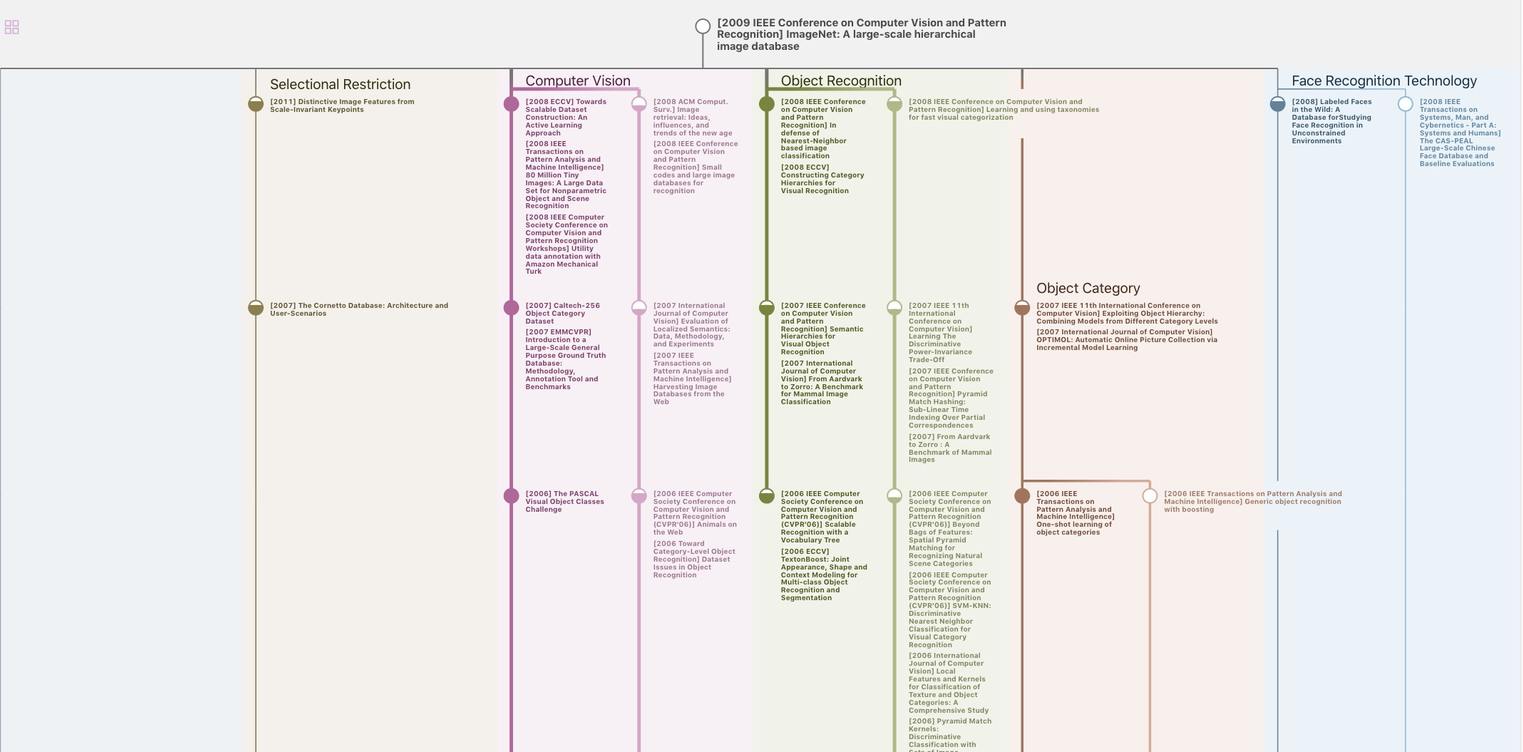
Generate MRT to find the research sequence of this paper
Chat Paper
Summary is being generated by the instructions you defined