Neutral Theory Based Mutation Operator For Differential Evolutionary Algorithms To Enhance Population Diversity
2019 INTERNATIONAL CONFERENCE ON ADVANCED ELECTRONIC MATERIALS, COMPUTERS AND MATERIALS ENGINEERING (AEMCME 2019)(2019)
Abstract
As an easily used and powerful heuristic search technique based on population, Differential Evolution (DE) algorithm has been widely applied for various global optimization and real engineering problems. Nevertheless, as with other Evolutionary Algorithms (EA), DE could not avoid from premature convergence due to over concentrated population, which could be called losing population diversity. In order to enhance its performance, we propose a Neutral Mutation (NM) operator for DE algorithm. This novel operator is inspired by neutral theory of molecular evolution, which claims that most mutations at the molecular level are neutral. That is to say, most variations observed are with neither advantage nor disadvantage fitness. Thus, they would not affect an organism's ability to survive and reproduce. The NM operator maintains slightly deleterious trial vectors, which we called neutral or nearly neutral, with a certain probability in the conventional selection operator of DE. Besides, some of these trial vectors have a chance to be neutrally mutated within the search domain randomly. As a result, the population is diversified with costing negligible Function Evaluations (FEs). Comprehensive experimental results demonstrate that the presented NM operator could improve population diversity to some extent, especially when the population is not divergent at all.
MoreTranslated text
Key words
differential evolutionary algorithms,mutation operator,population diversity
AI Read Science
Must-Reading Tree
Example
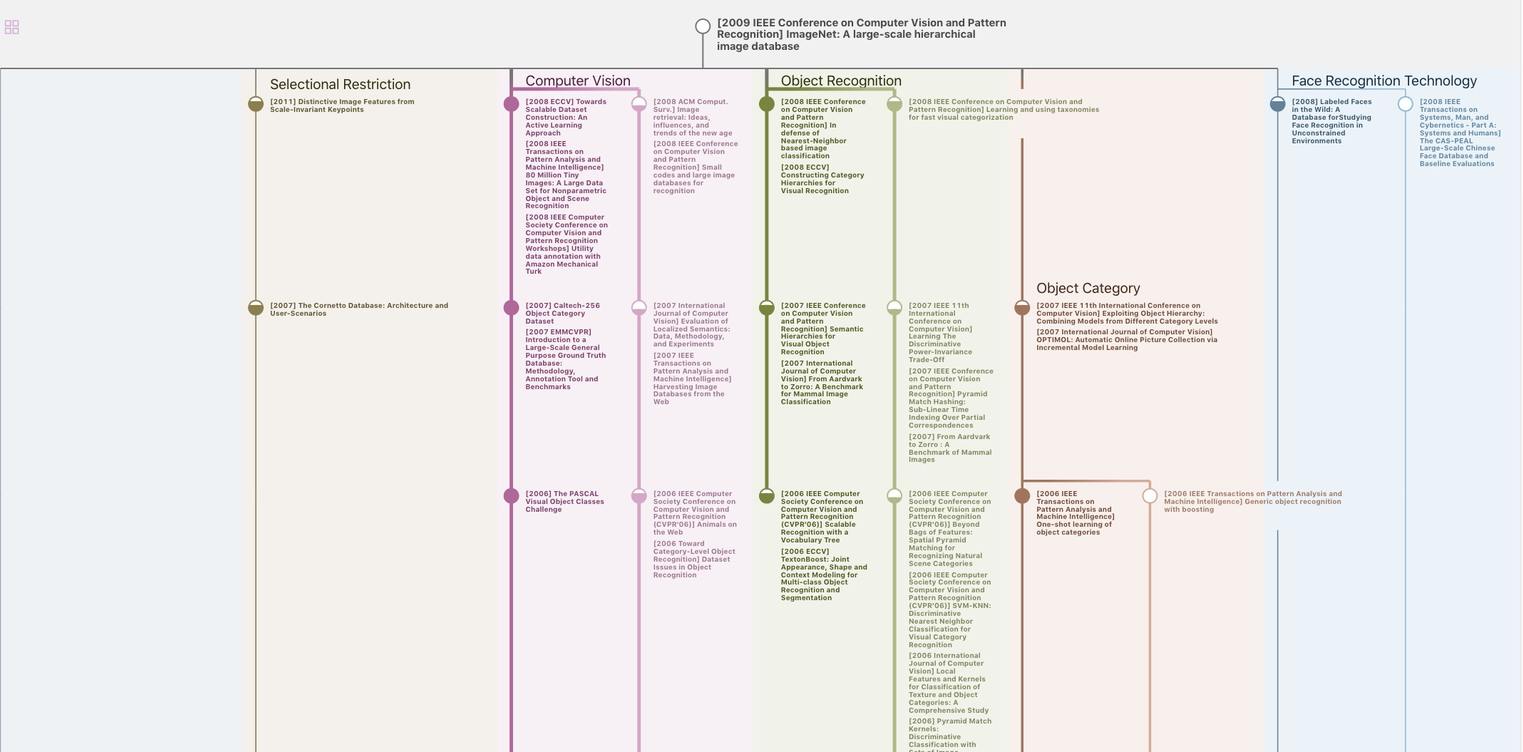
Generate MRT to find the research sequence of this paper
Chat Paper
Summary is being generated by the instructions you defined