Similarity Measures For Learning In Lattice Based Biomimetic Neural Networks
MATHEMATICS(2020)
Abstract
This paper presents a novel lattice based biomimetic neural network trained by means of a similarity measure derived from a lattice positive valuation. For a wide class of pattern recognition problems, the proposed artificial neural network, implemented as a dendritic hetero-associative memory delivers high percentages of successful classification. The memory is a feedforward dendritic network whose arithmetical operations are based on lattice algebra and can be applied to real multivalued inputs. In this approach, the realization of recognition tasks, shows the inherent capability of prototype-class pattern associations in a fast and straightforward manner without need of any iterative scheme subject to issues about convergence. Using an artificially designed data set we show how the proposed trained neural net classifies a test input pattern. Application to a few typical real-world data sets illustrate the overall network classification performance using different training and testing sample subsets generated randomly.
MoreTranslated text
Key words
biomimetic neural networks,dendritic computing,lattice neural networks,lattice valuations,pattern recognition,similarity measures
AI Read Science
Must-Reading Tree
Example
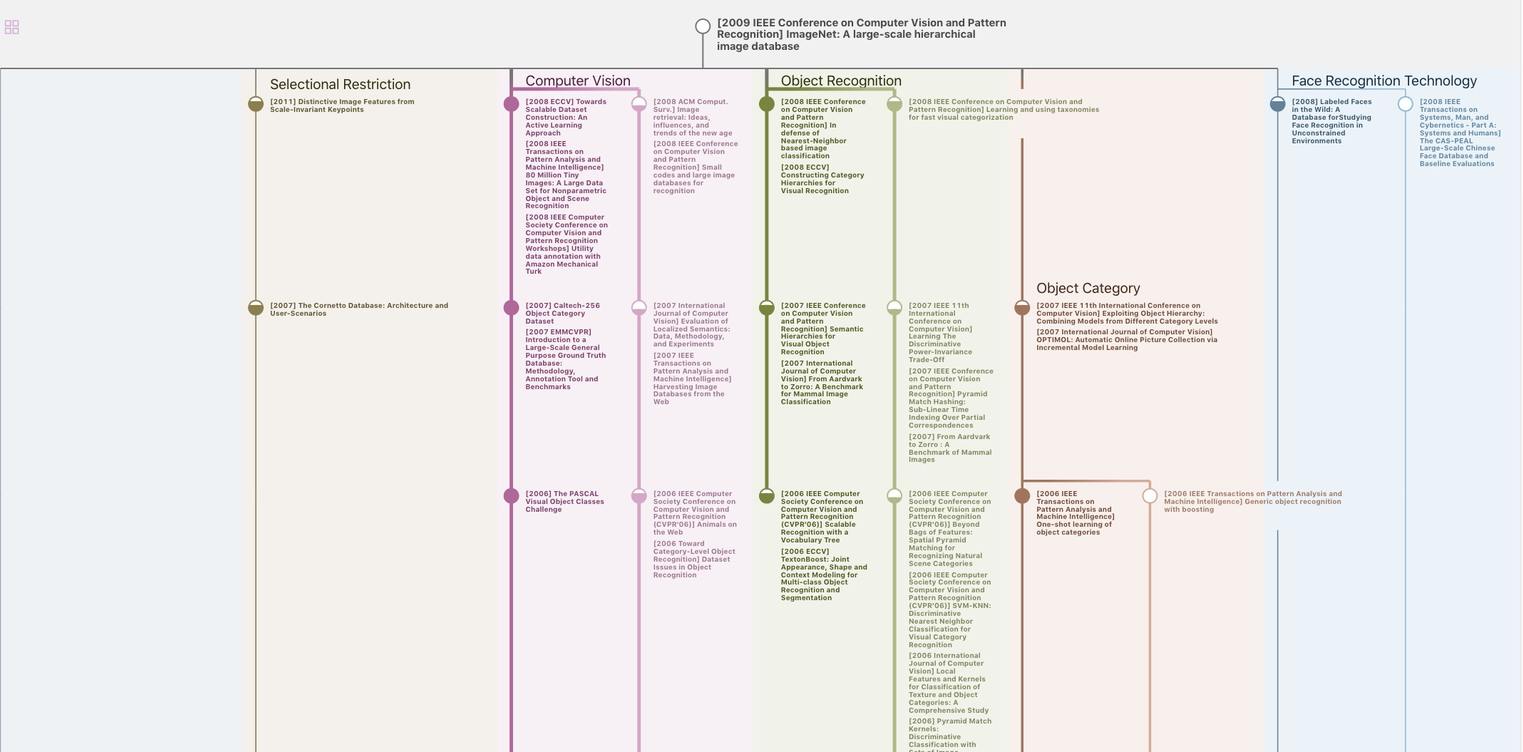
Generate MRT to find the research sequence of this paper
Chat Paper
Summary is being generated by the instructions you defined