Video Transcoding Optimization Based On Input Perceptual Quality
APPLICATIONS OF DIGITAL IMAGE PROCESSING XLIII(2020)
摘要
Today's video transcoding pipelines choose transcoding parameters based on rate-distortion curves, which mainly focus on the relative quality difference between original and transcoded videos. By investigating the recently released YouTube UGC dataset, we found that human subjects were more tolerant to changes in low quality videos than in high quality ones, which suggests that current transcoding frameworks can be further optimized by considering perceptual quality of the input. In this paper, an efficient machine learning metric is proposed to detect low quality inputs, whose bitrate can be further reduced without sacrificing perceptual quality. To evaluate the impact of our method on perceptual quality, we conducted a crowd-sourcing subjective experiment, and provided a methodology to evaluate statistical significance among different treatments. The results show that the proposed quality guided transcoding framework is able to reduce the average bitrate up to 5% with insignificant perceptual quality degradation.
更多查看译文
关键词
video transcoding, perceptual video quality, user generated contents, machine learning
AI 理解论文
溯源树
样例
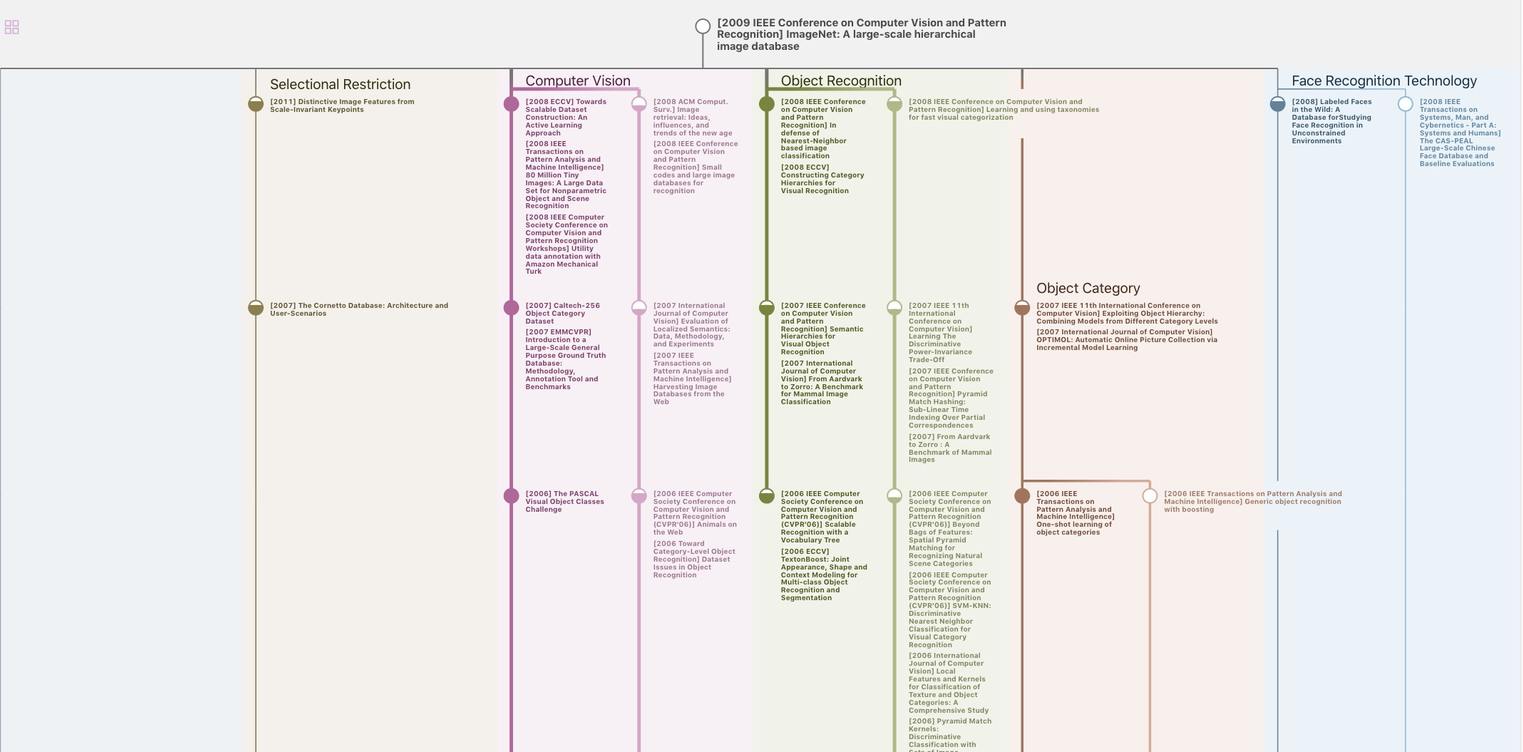
生成溯源树,研究论文发展脉络
Chat Paper
正在生成论文摘要