Predicting structure zone diagrams for thin film synthesis by generative machine learning
COMMUNICATIONS MATERIALS(2020)
摘要
Thin films are ubiquitous in modern technology and highly useful in materials discovery and design. For achieving optimal extrinsic properties, their microstructure needs to be controlled in a multi-parameter space, which usually requires too high a number of experiments to map. Here, we propose to master thin film processing microstructure complexity, and to reduce the cost of microstructure design by joining combinatorial experimentation with generative deep learning models to extract synthesis-composition-microstructure relations. A generative machine learning approach using a conditional generative adversarial network predicts structure zone diagrams. We demonstrate that generative models provide a so far unseen level of quality of generated structure zone diagrams that can be applied for the optimization of chemical composition and processing parameters to achieve a desired microstructure.
更多查看译文
关键词
Structural properties,Synthesis and processing,Materials Science,general
AI 理解论文
溯源树
样例
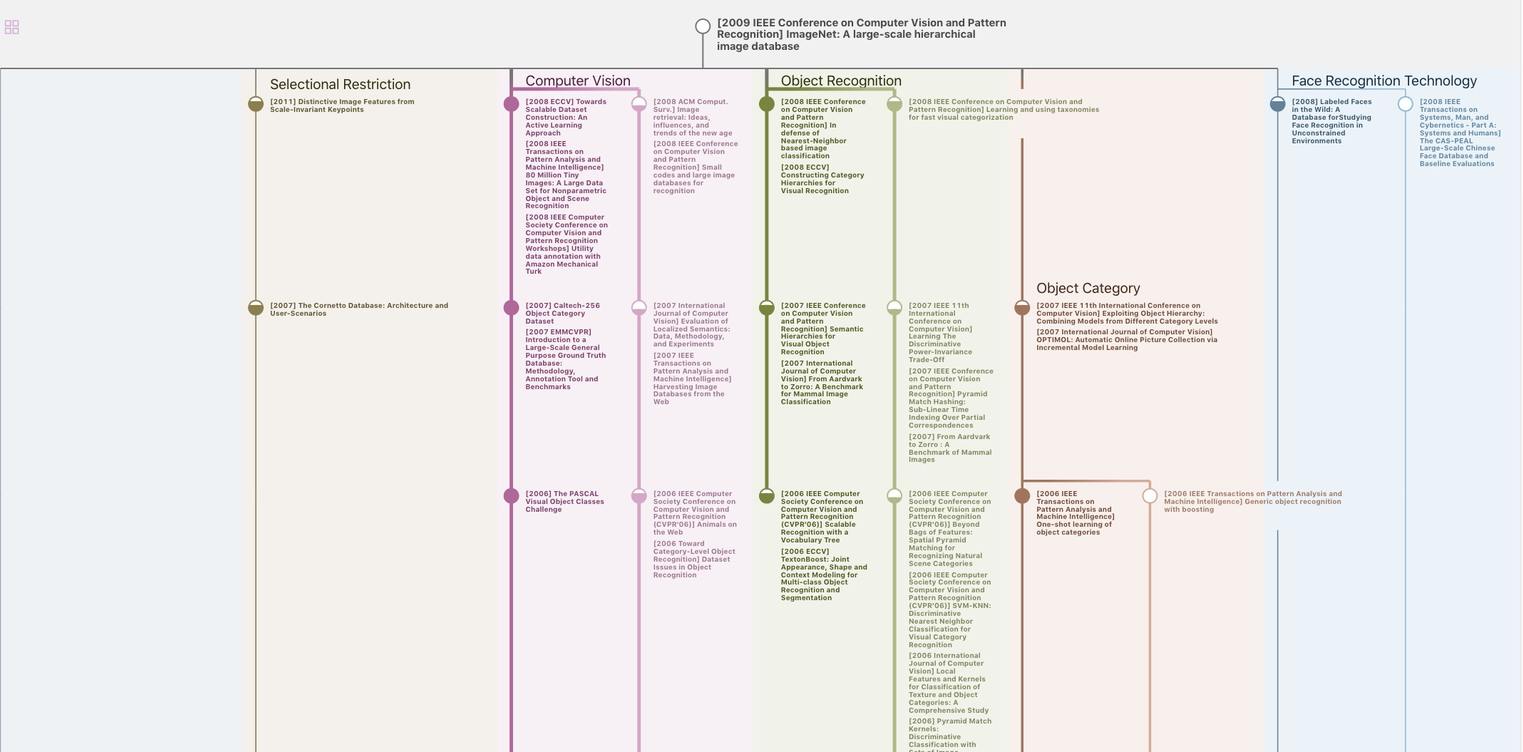
生成溯源树,研究论文发展脉络
Chat Paper
正在生成论文摘要