Multi-Temporal Crop Classification With Machine Learning Techniques
REMOTE SENSING FOR AGRICULTURE, ECOSYSTEMS, AND HYDROLOGY XXI(2019)
摘要
Many approaches for land cover classification rely on the spectral characteristics of the elements on the surface using one single multispectral image. Some land cover elements, as the vegetation and, in particular crops, are changing over seasons and over the growing cycle and may be characterized by their spectral temporal variability. In such cases, the spectral temporal variability can be used to model the crop's phenology and predict the crop type using both spatial and temporal spectral data. In this paper we aim to exploit the temporal dimension on the crop type classification using multi-temporal multispectral data and machine learning techniques. The high revisiting frequency of Sentinel-2 satellite opens new possibilities on the exploitation of high temporal resolution multispectral data. In this investigation, we evaluated the K - nearest neighbor (KNN), Random Forest (RF) and Decision Tree (DT) methods, for mapping 18 summer crops using Sentinel-2 data. Each method was applied to three different combinations of bands: a) all Sentinel-2 spectral bands (except band 10); b) vegetation indices (NDVI, EVI), Water Indices (NDWI, NDWI2, Moisture Index) and Normalized Image Indices and Brightness and c) the combination of the spectral bands and the indices. The best precisions we achieved were 98,6% with KNN, 98,9% with RF and 98.0% using a DT.
更多查看译文
关键词
Earth Observation Data, Machine Learning, Sentinel 2, Remote Sensing, Multi-spectral Imagery, Random Forest, Decision Tree, K - nearest neighbor, Agricultural Land-Cover Classification
AI 理解论文
溯源树
样例
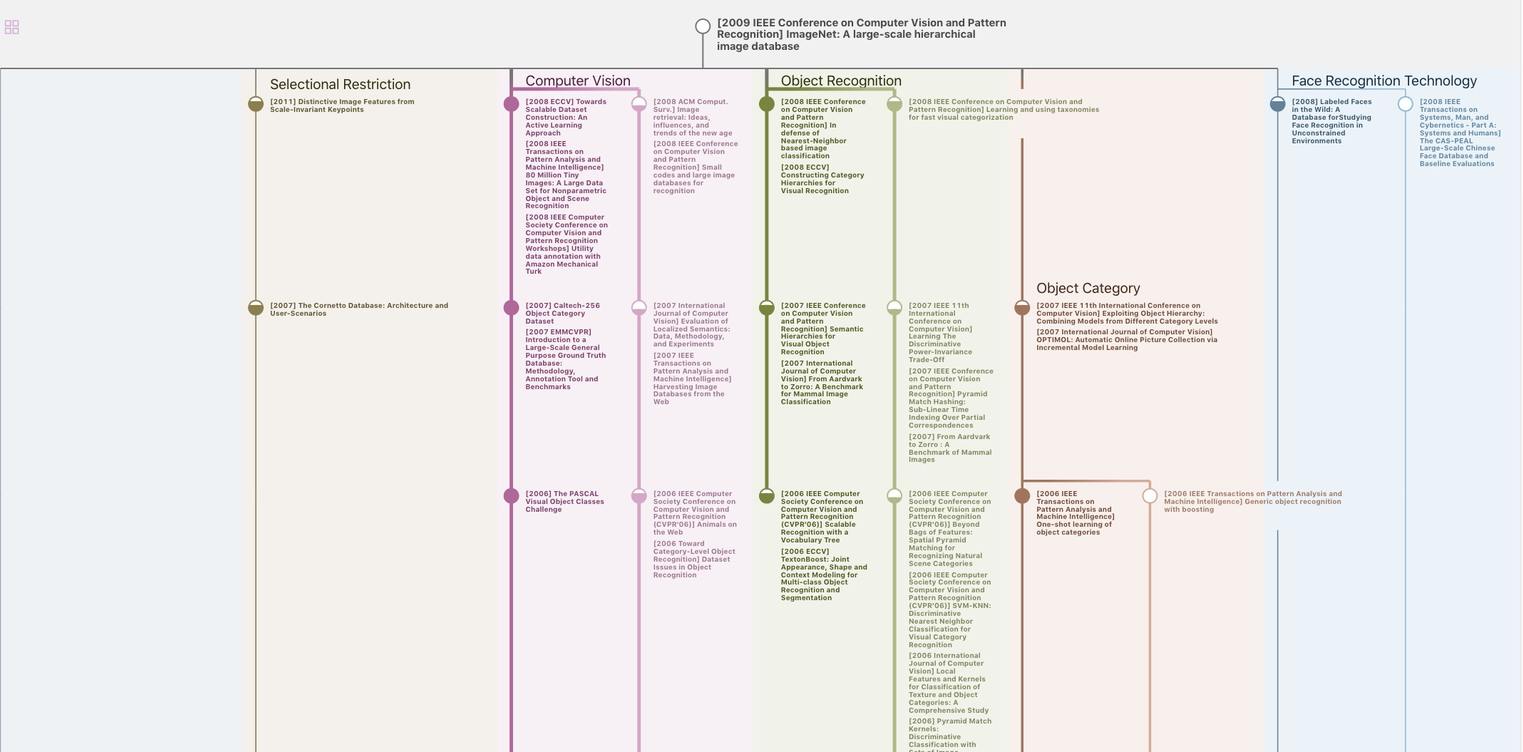
生成溯源树,研究论文发展脉络
Chat Paper
正在生成论文摘要