Robust Estimation Of Diffusion-Optimized Ensembles For Enhanced Sampling
BIOPHYSICAL JOURNAL(2014)
摘要
Molecular dynamics and Monte Carlo simulation can provide detailed structural insights into biological systems by exploring the distribution of accessible conformational states. However, due to the rough free energy landscapes characterizing many systems, obtaining converged statistics in such simulation remains an important challenge. In order to address this issue, over the past decades, a number of enhanced sampling strategies have been proposed, including methods like simulated annealing, replica exchange, metadynamics and generalized ensemble approaches such as multicanonical (flat histogram) sampling. Most of these methods were designed to soften the landscape, making it easier to overcome the free energy barriers. Recently, it was proposed that additional performance gains could be obtained by taking the position-dependent diffusion coefficient along the reaction coordinate into account, thus placing greater emphasis on regions diffusing slowly so that the round trip rate is maximized (Trebst et al. 2004. 10.1103/PhysRevE.70.046701). Although the diffusion-optimized ensemble approach has shown great promise, the practical application of it has been hindered by the serious challenge of estimating the diffusion coefficient accurately, especially at the preliminary stage of a simulation when only a small part of the phase space has been explored. In this study, a simple yet robust solution to this problem is presented. We propose replacing the standard global estimation procedure with a local estimation technique that was previously introduced in the context of trajectory analysis and reaction coordinate optimization (Krivov et al. 2008. 10.1073/pnas.0800228105). We demonstrate that the precision of the proposed estimation method is dramatically increased, requiring considerable fewer data compared to current state-of-the-art procedures. Finally, we show that with our method, diffusion-optimized sampling arises as a natural generalization of the standard multicanonical ensemble, estimating a simple histogram of transitions rather than the usual histogram of states.
更多查看译文
关键词
ensembles,robust estimation,diffusion-optimized
AI 理解论文
溯源树
样例
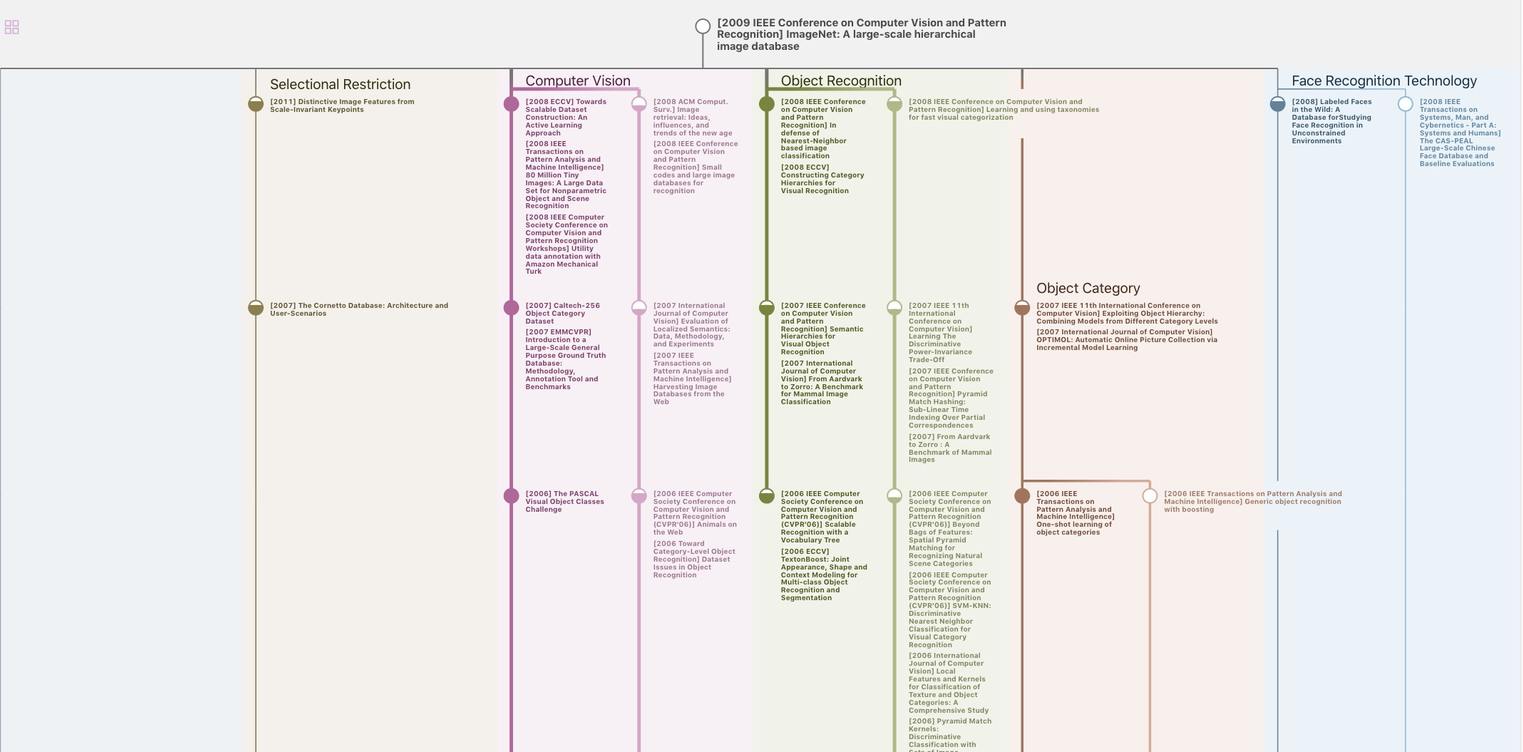
生成溯源树,研究论文发展脉络
Chat Paper
正在生成论文摘要