Predicting Of Stroke Risk Based On Icd Codes Using Graph-Based Convolutional Neural Networks.
INTERNATIONAL JOURNAL OF STROKE(2020)
摘要
In recent years, convolutional neural networks (CNNs) have emerged as highly efficient architectures for image and audio classification tasks, gaining widespread adoption in state-of-the-art methodologies. While CNNs excel in machine learning scenarios where the data representation exhibits a grid structure, they face challenges in generalizing to other data types. For instance, they struggle with data structured on 3D meshes (e.g., measurements from a network of meteorological stations) or data represented by graph structures (e.g., molecular graphs). To address such challenges, the scientific literature proposes novel graph-based convolutional network architectures, extending the classical convolution concept to data structures defined by graphs. In this paper, we use such a deep learning architecture to examine graphs defined using the ICD-10 codes appearing in the medical data of patients who suffered hemorrhagic stroke in Hungary in the period 2006–2012. The purpose of the analysis is to predict the risk of stroke by examining a patient’s ICD graph. Finally, we also compare the effectiveness of this method with classical machine learning classification methods. The results demonstrate that the graph-based method can predict the risk of stroke with an accuracy of over 73%, which is more than 10% higher than the classical methods.
更多查看译文
AI 理解论文
溯源树
样例
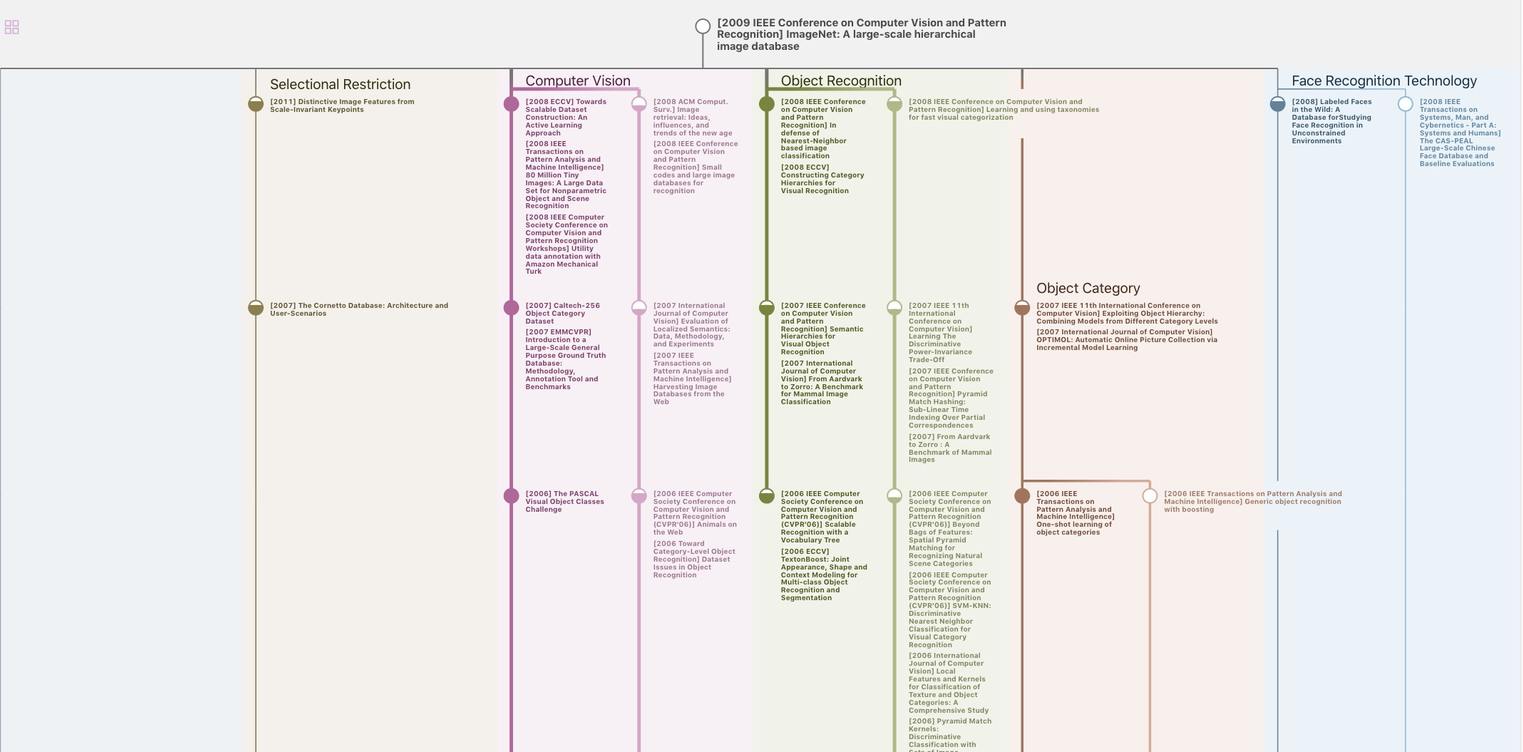
生成溯源树,研究论文发展脉络
Chat Paper
正在生成论文摘要