Effective Constraints Based Evolutionary Algorithm For Constrained Optimization Problems
INTERNATIONAL JOURNAL OF INNOVATIVE COMPUTING INFORMATION AND CONTROL(2012)
摘要
Evolutionary algorithms (EAs) have an enviable success record in solving many constrained optimization problems (COPs) in a variety of areas. However, those problems are afflicted by the highly constrained feasibility - isolated and small feasible regions, since EAs should thoroughly test feasibility for all constraints at every generation. To systematically deal with its limitation, this paper presents a new approach for effectively handling the constraints of COPs. The major idea is to extract an actual subset of meaningful constraints, termed "effective constraints", from the current population. This discovered set plays a key role in satisfying the feasibility within the certain tolerance specified by the statistics on feasible solutions and several prefixed criteria on feasibility. Thanks to the proposed effective constraints, it is able to evolve the population toward the legitimate region of the search space without assessing all the constraints; thus, the better feasible space is yielded than that of originals. The proposed constraint-handling technique is combined with a widely-used EA, stochastic ranking evolutionary strategy, for achieving the optimal solutions of COPs. The proposed algorithm is compared with several well-known references on three real-world engineering optimization problems. Computational studies verify that the proposed algorithm achieves better solutions than those of the existing algorithms.
更多查看译文
关键词
Effective constraints, Feasibility statistics, Stochastic ranking evolutionary strategy, Engineering optimization problems
AI 理解论文
溯源树
样例
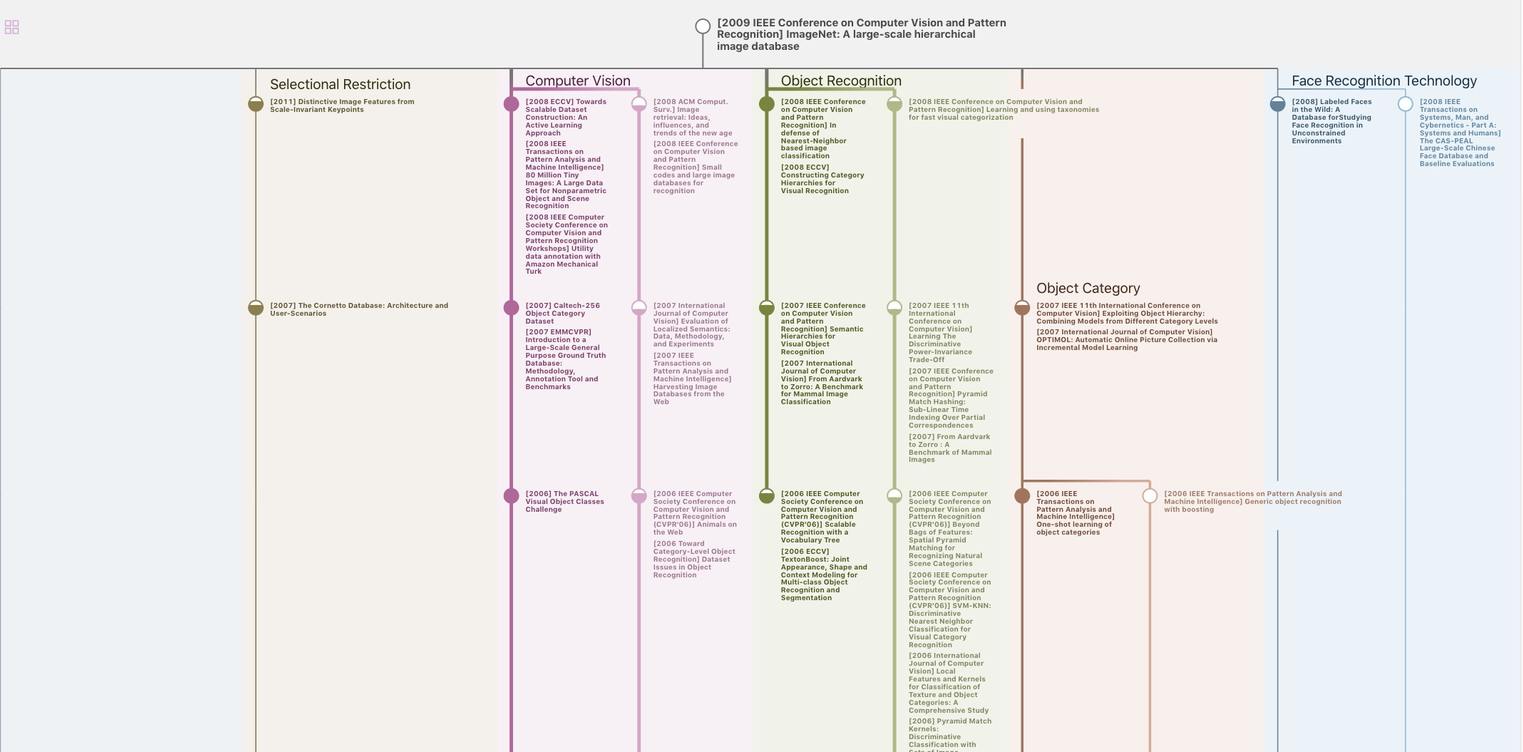
生成溯源树,研究论文发展脉络
Chat Paper
正在生成论文摘要