Analysis Of Rank-1 Updating In Decentralized Estimation For Collaborative Navigation
PROCEEDINGS OF THE 32ND INTERNATIONAL TECHNICAL MEETING OF THE SATELLITE DIVISION OF THE INSTITUTE OF NAVIGATION (ION GNSS+ 2019)(2019)
Abstract
Relative positioning of networked platforms is a key technical enabler for many missions particularly in environments where GPS is challenged or denied. Collaborative navigation of such networked platforms makes use of relative range, range rate, and/or bearing measurements to each other and/or to a third party so as to improve the relative positioning accuracy and possibly the absolute one. A centralized processing scheme, which gathers all measurements from all nodes and then disseminates the processing results, though being theoretically optimal, is not scalable as the network size increases. Besides, it presents the risk of a single point failure.A distributed decentralized estimation strategy, though suboptimal, is practical. The navigation states of active nodes in the network are communicated to a node via data link and those neighboring nodes that are involved in common view and/or internodal sensing are used in assisting the node to improve its navigation. However, each distributed estimator needs to take into account the uncertainty of the assisting states. In addition, the use of common view and/or inter-nodal measurements, though not dynamically coupled, makes their state estimation errors correlated. This cross-correlation, if not accounted for properly, would make the estimation too optimistic (inconsistent) and may lead to divergence (de-weighting and discarding new measurements). The adjacent nodal uncertainty and inter-nodal cross-correlation are two major issues to address for a viable scalable distributed estimation mechanization.In this paper, we perform a theoretical analysis of inter-nodal rank-1 updating. The behavior of collaborative navigation with inter-nodal measurements differs from a standalone navigation with similar measurements to a reference point. It is shown that the error reduction is not symmetric for collaborative navigation, depending on relative errors of each node. The direction of minimum error of a node can serve as a reference for others with large errors in that direction, which has implications in sensor selection and/or path planning. Furthermore, there is an initial error reduction when two nodes first communicate (a teamwork effect due to spatial averaging) and no further reduction afterwards due to cross-correlation (a reset effect without temporal averaging). However, as the agents propagate in space and time, their state estimates become de-correlated due to inertial error growth and local updates with different sensors. At this point, inter-nodal updating becomes more cost-effective again. Simulation results are presented to support the analyses with discussion on implications for algorithm design and implementation.
MoreTranslated text
Key words
collaborative navigation,decentralized estimation
AI Read Science
Must-Reading Tree
Example
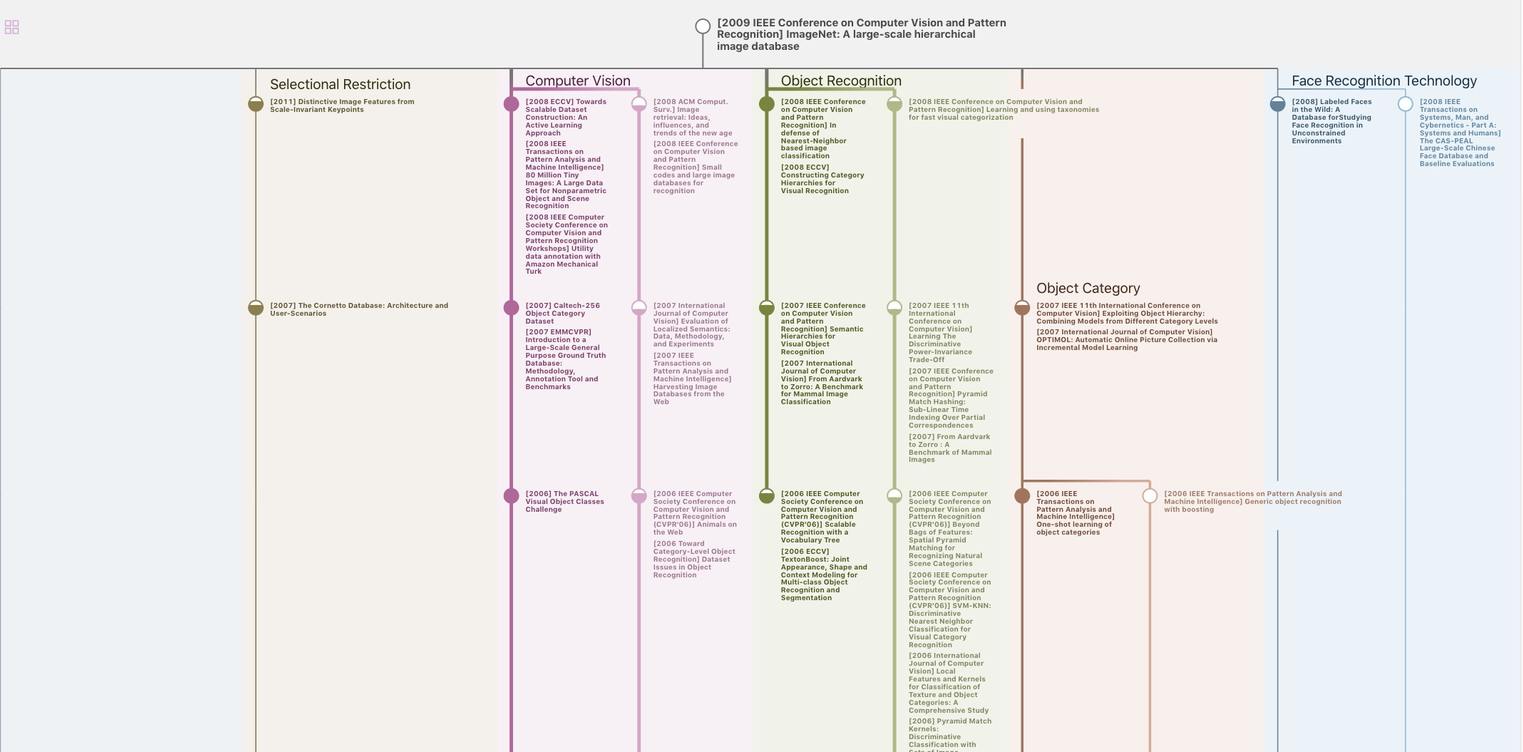
Generate MRT to find the research sequence of this paper
Chat Paper
Summary is being generated by the instructions you defined