Using Copula To Model Dependence When Testing Multiple Hypotheses In Dna Microarray Experiments: A Bayesian Approximation
MATHEMATICS(2020)
摘要
Many experiments require simultaneously testing many hypotheses. This is particularly relevant in the context of DNA microarray experiments, where it is common to analyze many genes to determine which of them are differentially expressed under two conditions. Another important problem in this context is how to model the dependence at the level of gene expression. In this paper, we propose a Bayesian procedure for simultaneously testing multiple hypotheses, modeling the dependence through copula functions, where all available information, both objective and subjective, can be used. The approach has the advantage that it can be used with different dependency structures. Simulated data analysis was performed to examine the performance of the proposed approach. The results show that our procedure captures the dependence appropriately classifying adequately a high percentage of true and false null hypotheses when choosing a prior distribution beta skewed to the right for the initial probability of each null hypothesis, resulting in a very powerful procedure. The procedure is also illustrated with real data.
更多查看译文
关键词
bayesian inference, copula function, dependence, DNA microarrays, testing multiple hypotheses
AI 理解论文
溯源树
样例
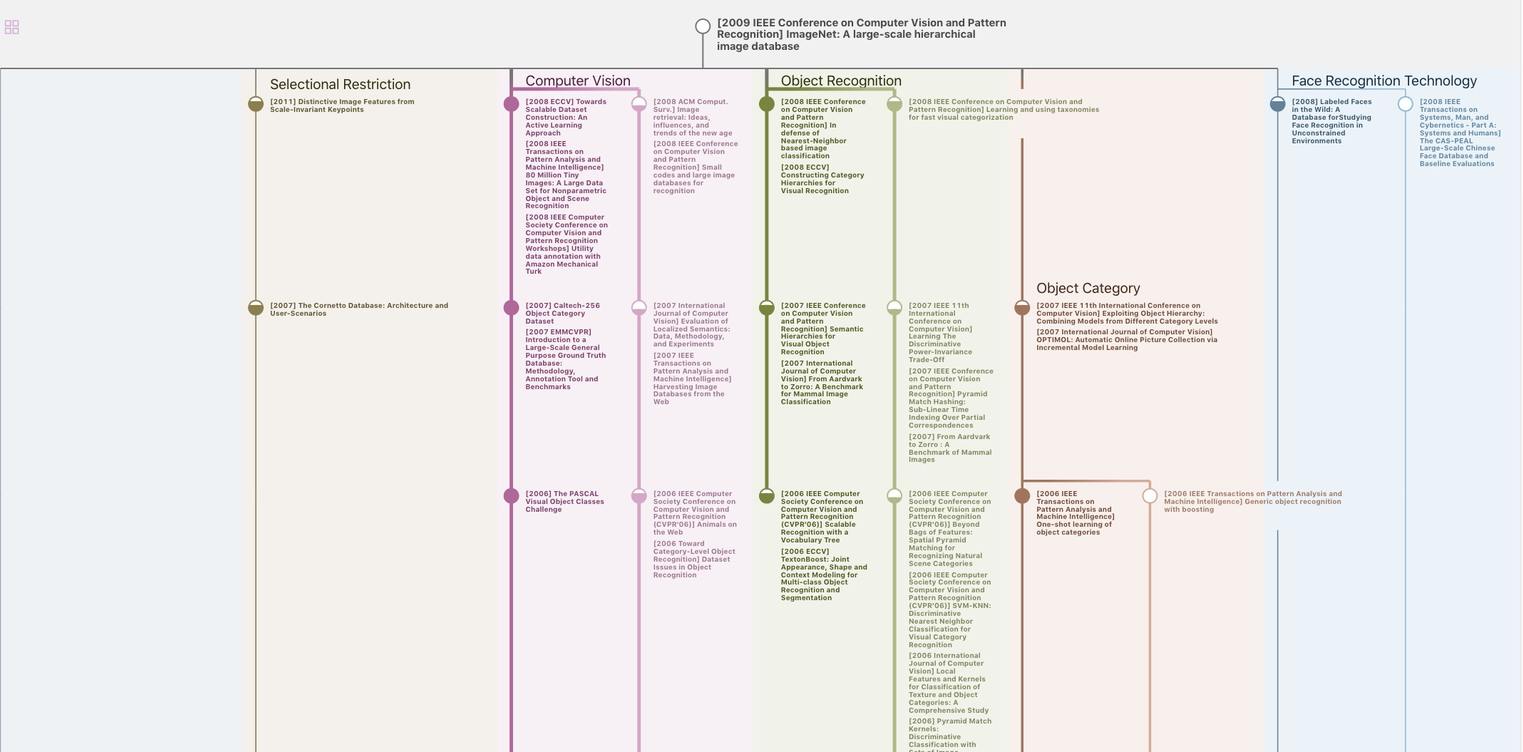
生成溯源树,研究论文发展脉络
Chat Paper
正在生成论文摘要