insideOutside: an accessible algorithm for classifying interior and exterior points, with applications in embryology.
biorxiv(2023)
摘要
A crucial aspect of embryology is relating the position of individual cells to the broader geometry of the embryo. A classic example of this is the first cell-fate decision of the mouse embryo, where interior cells become inner cell mass and exterior cells become trophectoderm. Fluorescent labelling, imaging, and quantification of tissue-specific proteins have advanced our understanding of this dynamic process. However, instances arise where these markers are either not available, or not reliable, and we are left only with the cells' spatial locations. Therefore, a simple, robust method for classifying interior and exterior cells of an embryo using spatial information is required. Here, we describe a simple mathematical framework and an unsupervised machine learning approach, termed insideOutside, for classifying interior and exterior points of a three-dimensional point-cloud, a common output from imaged cells within the early mouse embryo. We benchmark our method against other published methods to demonstrate that it yields greater accuracy in classification of nuclei from the pre-implantation mouse embryos and greater accuracy when challenged with local surface concavities. We have made MATLAB and Python implementations of the method freely available. This method should prove useful for embryology, with broader applications to similar data arising in the life sciences.
更多查看译文
关键词
machine learning,quantitative biology,pre-implantation,embryo,inner cell mass,trophectoderm
AI 理解论文
溯源树
样例
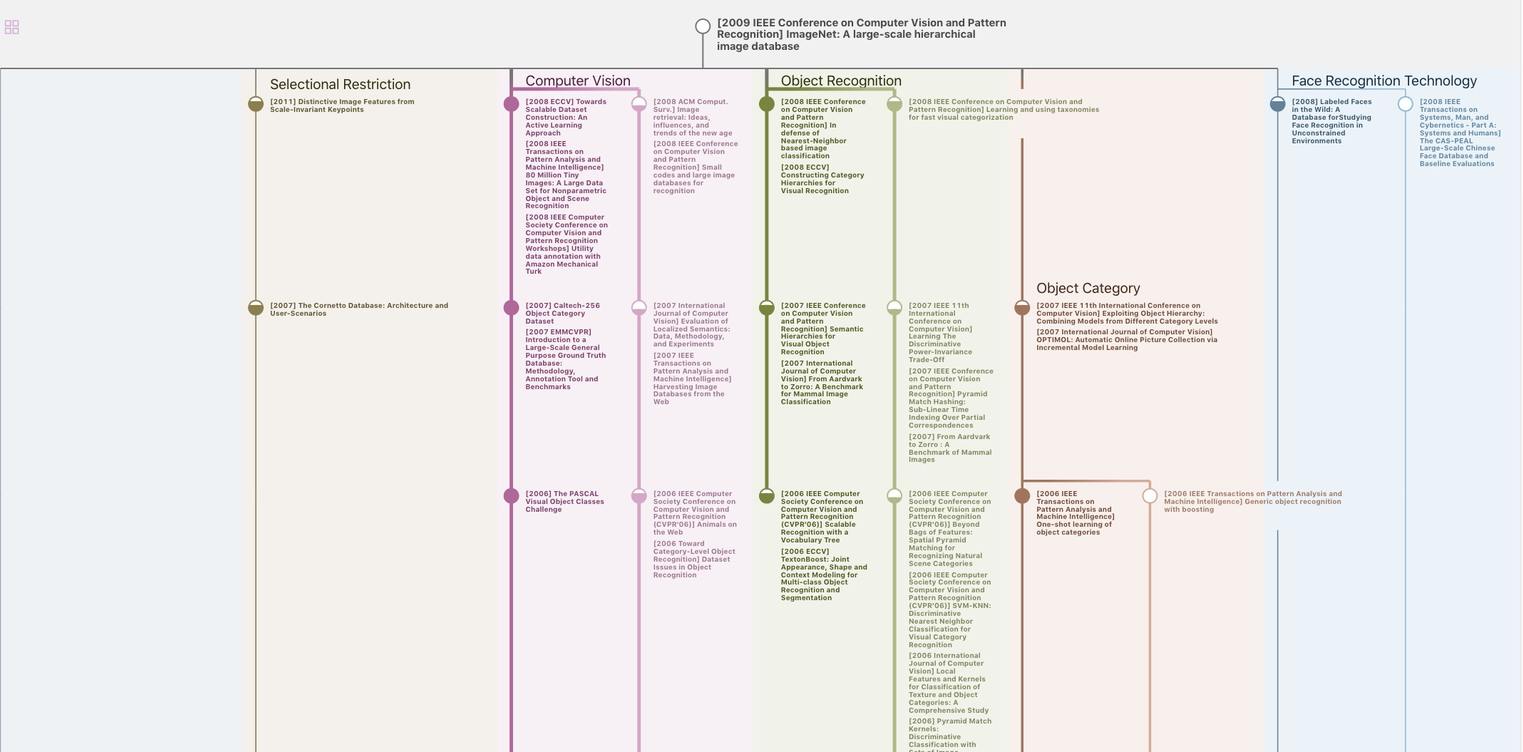
生成溯源树,研究论文发展脉络
Chat Paper
正在生成论文摘要