Attractor Landscapes as a Model Selection Criterion in Data Poor Environments
biorxiv(2021)
摘要
Modeling of systems for which data is limited often leads to underdetermined model identification problems, where multiple candidate models are equally adherent to data. In such situations additional optimality criteria are useful in model selection apart from the conventional minimization of error and model complexity. This work presents the attractor landscape as a domain for novel model selection criteria, where the number and location of attractors impact desirability. A set of candidate models describing immune response dynamics to SARS-CoV infection is used as an example for model selection based on features of the attractor landscape. Using this selection criteria, the initial set of 18 models is ranked and reduced to 7 models that have a composite objective value with a p-value < 0.05. Additionally, the impact of pharmacologically induced remolding of the attractor landscape is presented.
### Competing Interest Statement
The authors have declared no competing interest.
更多查看译文
关键词
model selection criterion,data
AI 理解论文
溯源树
样例
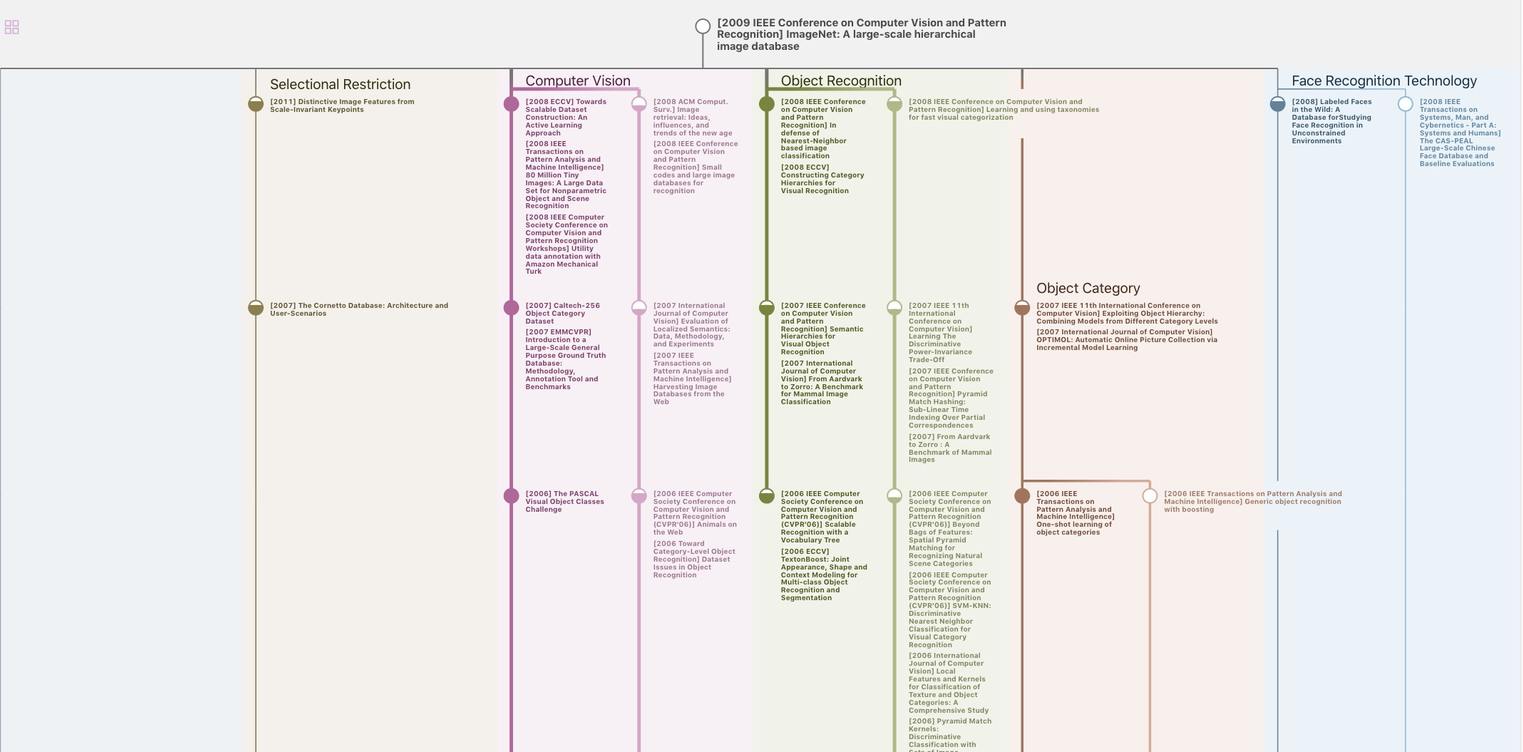
生成溯源树,研究论文发展脉络
Chat Paper
正在生成论文摘要