Configuring a Federated Network of Real-World Patient Health Data for Multimodal Deep Learning Prediction of Health Outcomes
Proceedings of the 37th ACM/SIGAPP Symposium on Applied Computing(2022)
关键词
Federated Data Network,Deep Learning,Comorbidity,Lab Measurements,Electronic Health Records
AI 理解论文
溯源树
样例
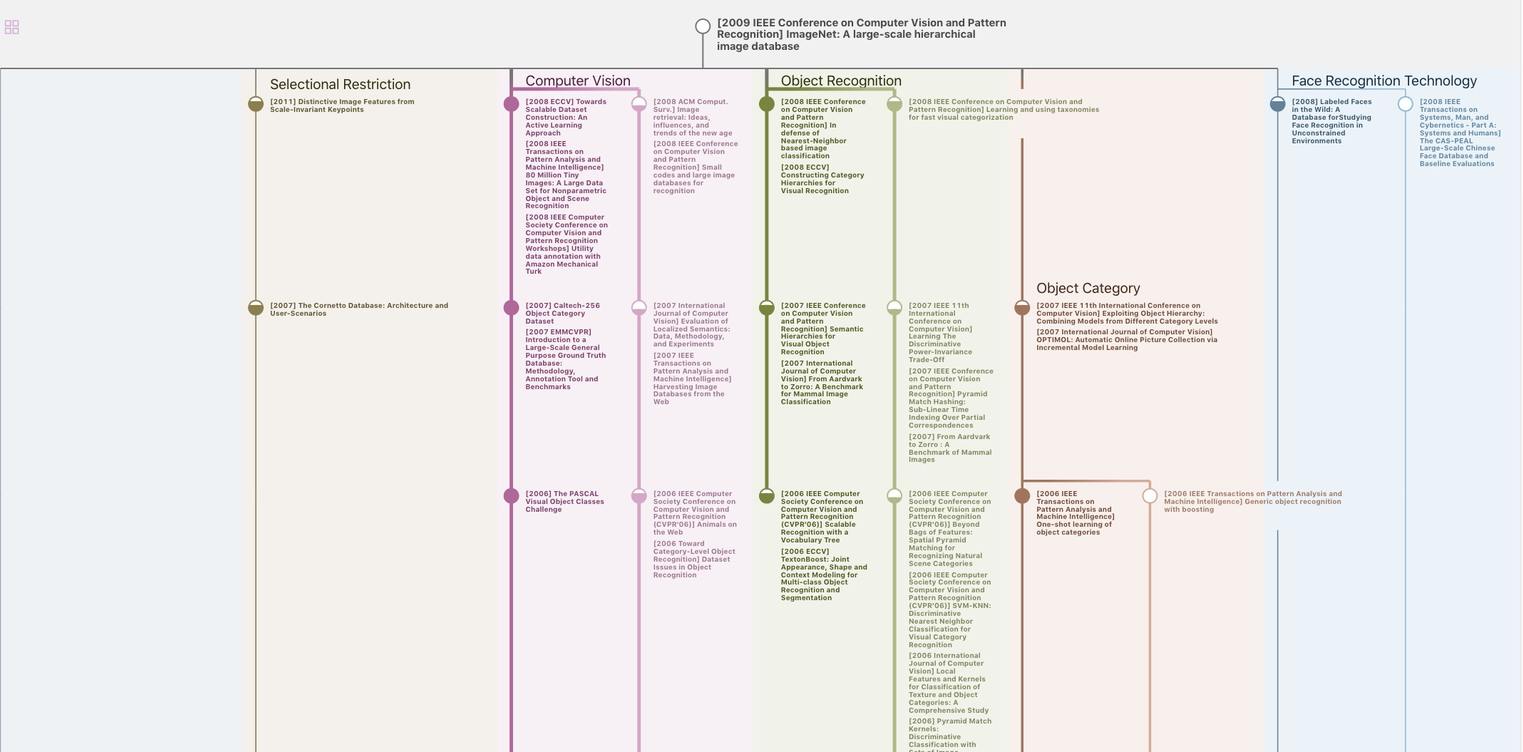
生成溯源树,研究论文发展脉络
Chat Paper
正在生成论文摘要