Data cleaning for image-based profiling enhancement
biorxiv(2021)
摘要
With the advent of high-throughput assays, a large number of biological experiments can be carried out. Image-based assays are among the most accessible and inexpensive technologies for this purpose. Indeed, these assays have proved to be effective in characterizing unknown functions of genes and small molecules. Image analysis pipelines have a pivotal role in translating raw images that are captured in such assays into useful and compact representation, also known as measurements. CellProfiler is a popular and commonly used tool for this purpose through providing readily available modules for the cell/nuclei segmentation, and making various measurements, or features, for each cell/nuclei. Single cell features are then aggregated for each treatment replica to form treatment “profiles.” However, there may be several sources of error in the CellProfiler quantification pipeline that affects the downstream analysis that is performed on the profiles. In this work, we examined various preprocessing approaches to improve the profiles. We consider identification of drug mechanisms of action as the downstream task to evaluate such preprocessing approaches. Our enhancement steps mainly consist of data cleaning, cell level outlier detection, toxic drug detection, and regressing out the cell area from all other features, as many of them are widely affected by the cell area. We also examined unsupervised and weakly-supervised deep learning based methods to reduce the feature dimensionality, and finally suggest possible avenues for future research.
### Competing Interest Statement
The authors have declared no competing interest.
更多查看译文
关键词
profiling,data,enhancement,image-based
AI 理解论文
溯源树
样例
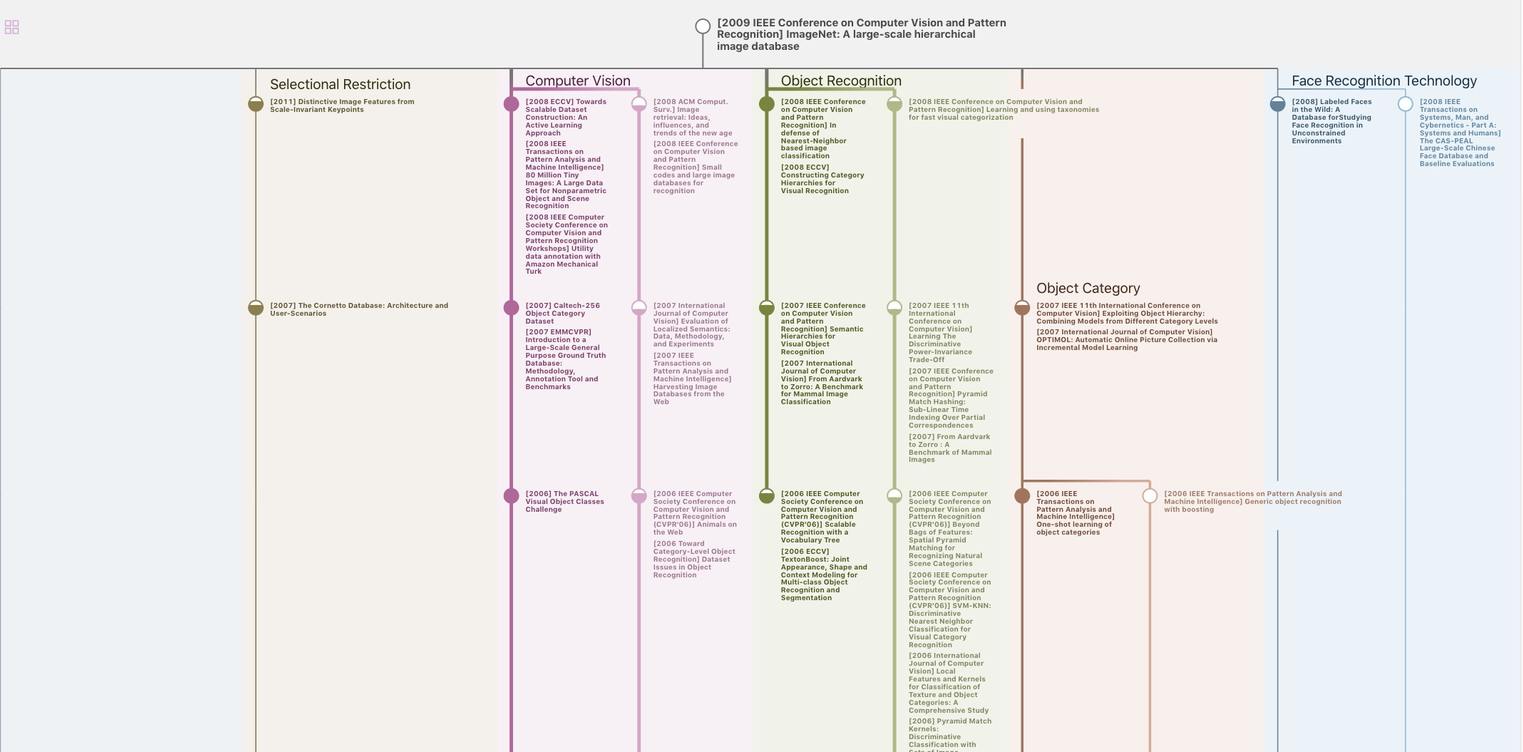
生成溯源树,研究论文发展脉络
Chat Paper
正在生成论文摘要