Identifying Gene-wise Differences in Latent Space Projections Across Cell Types and Species in Single Cell Data using scProject
biorxiv(2021)
Abstract
Latent space techniques have emerged as powerful tools to identify genes and gene sets responsible for cell-type and species-specific differences in single-cell data. Transfer learning methods can compare learned latent spaces across biological systems. However, the robustness that comes from leveraging information across multiple genes in transfer learning is often attained at the sacrifice of gene-wise precision. Thus, methods are needed to identify genes, defined as important within a particular latent space, that significantly differ between contexts. To address this challenge, we have developed a new framework, scProject, and a new metric, projectionDrivers, to quantitatively examine latent space usage across single-cell experimental systems while concurrently extracting the genes driving the differential usage of the latent space between defined contrasts. Here, we demonstrate the efficacy, utility, and scalability of scProject with projectionDrivers and provide experimental validation for predicted species-specific differences between the developing mouse and human retina.
### Competing Interest Statement
The authors have declared no competing interest.
MoreTranslated text
Key words
single cell data,latent space projections,cell types,gene-wise
AI Read Science
Must-Reading Tree
Example
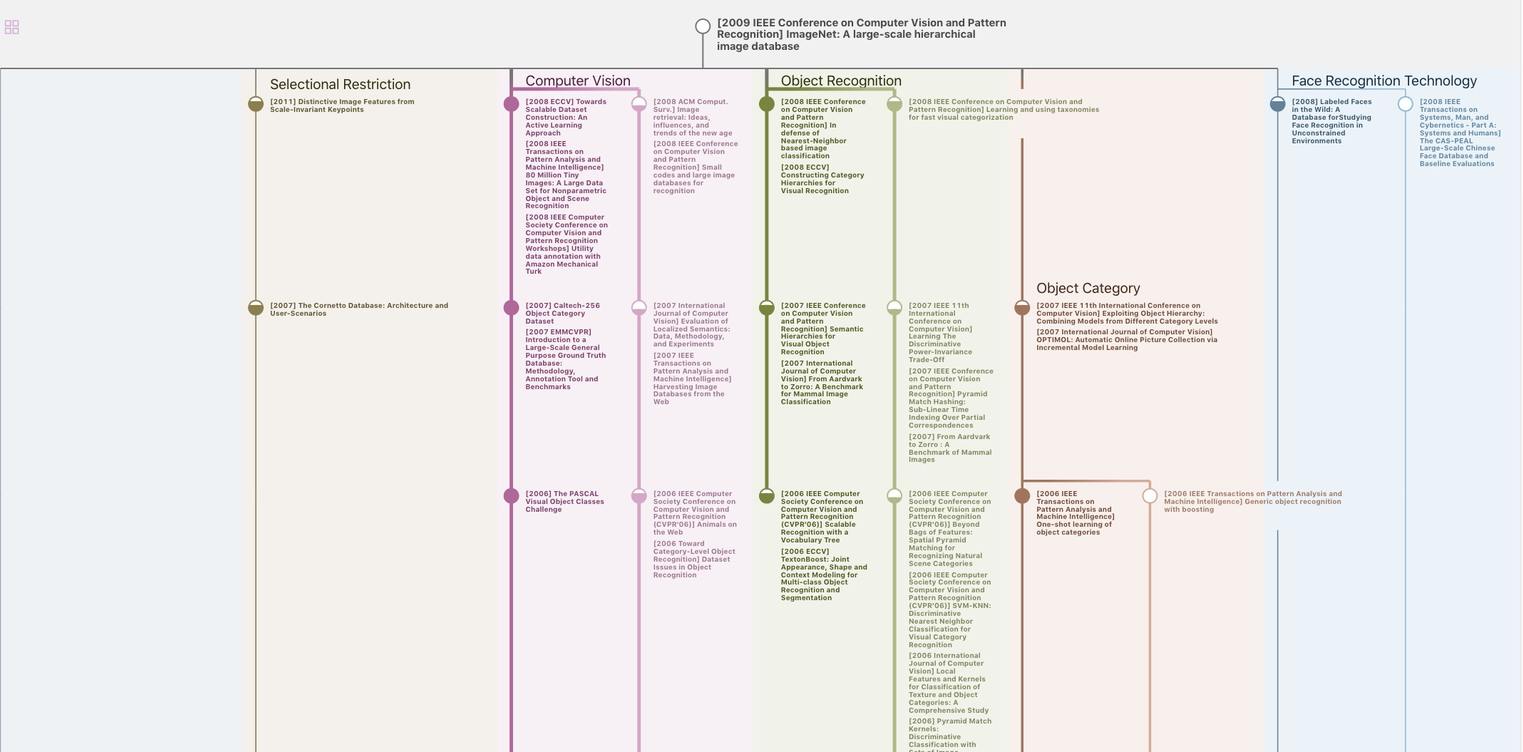
Generate MRT to find the research sequence of this paper
Chat Paper
Summary is being generated by the instructions you defined