A BAYESIAN PRECISION MEDICINE FRAMEWORK FOR CALIBRATING INDIVIDUALIZED THERAPEUTIC INDICES IN CANCER
ANNALS OF APPLIED STATISTICS(2022)
摘要
The development and clinical implementation of evidence-based preci-sion medicine strategies has become a realistic possibility, primarily due to the rapid accumulation of large-scale genomics and pharmacological data from diverse model systems: patients, cell lines and drug perturbation studies. We introduce a novel Bayesian modeling framework called the individualized theRapeutic index (iRx) model to integrate high-throughput pharmacoge-nomic data across model systems. Our iRx model achieves three main goals: first, it exploits the conserved biology between patients and cell lines to cal-ibrate therapeutic response of drugs in patients; second, it finds optimal cell line avatars as proxies for patient(s); and finally, it identifies key genomic drivers explaining cell line-patient similarities. This is achieved through a semi-supervised learning approach that conflates (unsupervised) sparse latent factor models with (supervised) penalized regression techniques. We propose a unified and tractable Bayesian model for estimation, and inference is con-ducted via efficient posterior sampling schemes. We illustrate and validate our approach using two existing clinical trial data sets in multiple myeloma and breast cancer studies. We show that our iRx model improves prediction accuracy compared to naive alternative approaches, and it consistently out-performs existing methods in literature in both multiple simulation scenarios as well as real clinical examples.
更多查看译文
关键词
Bayesian methods, genomic data integration, high-dimensional regression, latent factor models, precision medicine, semi-supervised learning
AI 理解论文
溯源树
样例
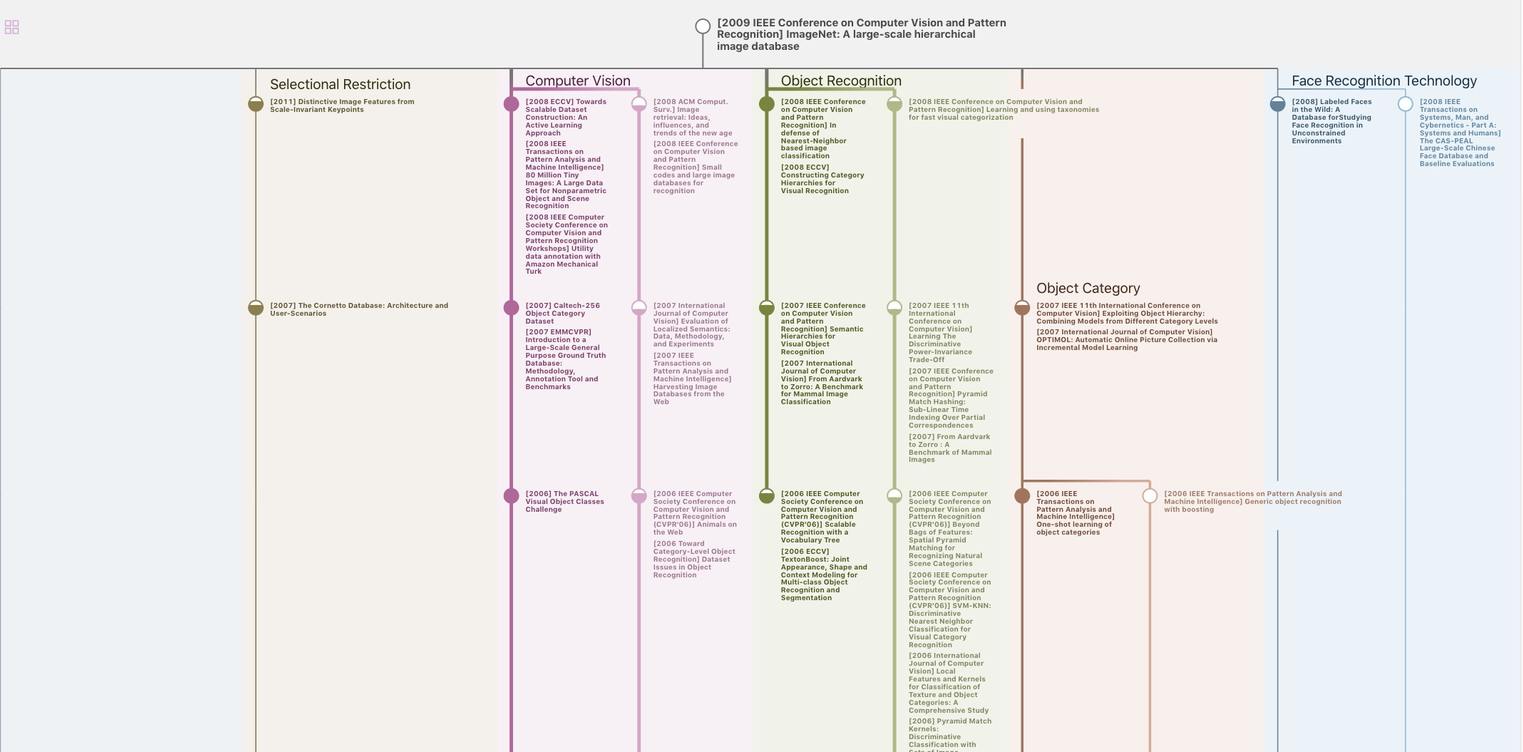
生成溯源树,研究论文发展脉络
Chat Paper
正在生成论文摘要