Patient Informed Domain Adaptation Improves Clinical Drug Response Prediction
bioRxiv (Cold Spring Harbor Laboratory)(2021)
摘要
In-silico modeling of patient clinical drug response (CDR) promises to revolutionize personalized cancer treatment. State-of-the-art CDR predictions are usually based on cancer cell line drug perturbation profiles. However, prediction performance is limited due to the inherent differences between cancer cell lines and primary tumors. In addition, current computational models generally do not leverage both chemical information of a drug and a gene expression profile of a patient during training, which could boost prediction performance. Here we develop a Patient Adapted with Chemical Embedding (PACE) dual convergence deep learning framework that a) integrates gene expression along with drug chemical structures, and b) is adapted in an unsupervised fashion by primary tumor gene expression. We show that PACE achieves better discrimination between sensitive and resistant patients compared to the state-of-the-art linear regularized method (9/12 VS 3/12 drugs with available clinical outcomes) and alternative methods.
### Competing Interest Statement
The authors have declared no competing interest.
* GCN
: Graph Convolutional Network
MorganFP
: Morgan Fingerprint
SMILES
: Simplified Molecular Input Line Entry System for annotating chemical structures using character strings
ML/DL
: machine learning/deep learning
CDR
: Clinician Drug Response
CDI
: Cell-line-Drug-IC50
EM
: Expression Module
DM
: Drug Module
PM
: Prediction Module
OOD
: Out of Distribution
CL
: Cell Line
更多查看译文
关键词
adaptation,prediction,drug,domain
AI 理解论文
溯源树
样例
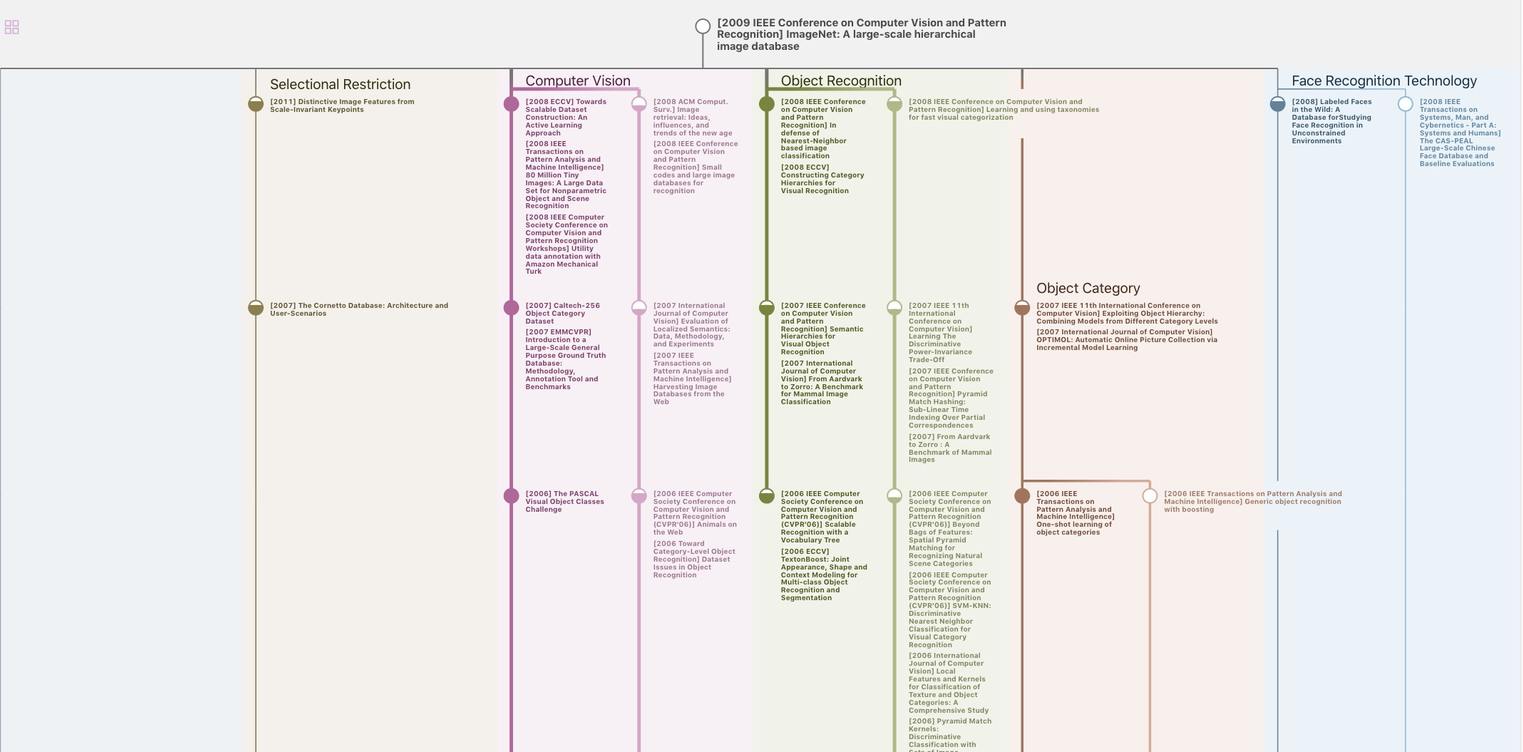
生成溯源树,研究论文发展脉络
Chat Paper
正在生成论文摘要