An interactive deep learning-based approach reveals mitochondrial cristae topologies
biorxiv(2023)
摘要
The convolution of membranes called cristae is a critical structural and functional feature of mitochondria. Crista structure is highly diverse between different cell types, reflecting their role in metabolic adaptation. However, their precise three-dimensional (3D) arrangement requires volumetric analysis of serial electron microscopy and has therefore been limiting for unbiased quantitative assessment. Here, we developed a novel, publicly available, deep learning (DL)-based image analysis platform called Python-based human-in-the-loop workflow (PHILOW) implemented with a human-in-the-loop (HITL) algorithm. Analysis of dense, large, and isotropic volumes of focused ion beam-scanning electron microscopy (FIB-SEM) using PHILOW reveals the complex 3D nanostructure of both inner and outer mitochondrial membranes and provides deep, quantitative, structural features of cristae in a large number of individual mitochondria. This nanometer-scale analysis in micrometer-scale cellular contexts uncovers fundamental parameters of cristae, such as total surface area, orientation, tubular/lamellar cristae ratio, and crista junction density in individual mitochondria. Unbiased clustering analysis of our structural data unraveled a new function for the dynamin-related GTPase Optic Atrophy 1 (OPA1) in regulating the balance between lamellar versus tubular cristae subdomains. By developing a novel platform for a deep learning-based analysis of volume electron microscopy images, this study provides unprecedented nanometer-scale and comprehensive cristae structure in more than 400 individual mitochondria, revealing a previously unknown function of OPA1.
更多查看译文
关键词
learning-based
AI 理解论文
溯源树
样例
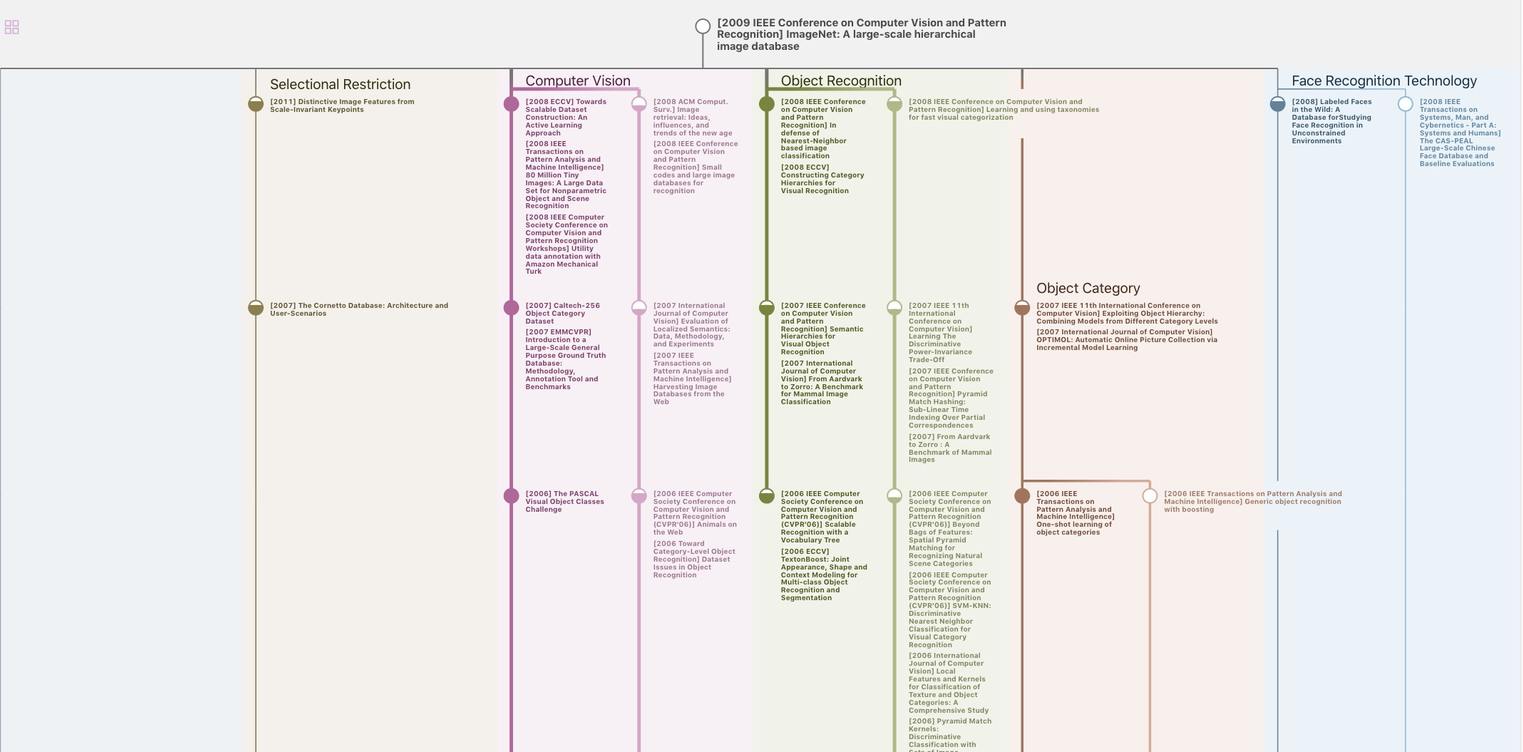
生成溯源树,研究论文发展脉络
Chat Paper
正在生成论文摘要