Common permutation methods in animal social network analysis do not control for non-independence
Behavioral ecology and sociobiology(2022)
摘要
The non-independence of social network data is a cause for concern among behavioural ecologists conducting social network analysis. This has led to the adoption of several permutation-based methods for testing common hypotheses. One of the most common types of analysis is nodal regression, where the relationships between node-level network metrics and nodal covariates are analysed using a permutation technique known as node-label permutations. We show that, contrary to accepted wisdom, node-label permutations do not automatically account for the non-independences assumed to exist in network data, because regression-based permutation tests still assume exchangeability of residuals. The same assumption also applies to the quadratic assignment procedure (QAP), a permutation-based method often used for conducting dyadic regression. We highlight that node-label permutations produce the same p -values as equivalent parametric regression models, but that in the presence of non-independence, parametric regression models can also produce accurate effect size estimates. We also note that QAP only controls for a specific type of non-independence between edges that are connected to the same nodes, and that appropriate parametric regression models are also able to account for this type of non-independence. Based on this, we suggest that standard parametric models could be used in the place of permutation-based methods. Moving away from permutation-based methods could have several benefits, including reducing over-reliance on p- values, generating more reliable effect size estimates, and facilitating the adoption of causal inference methods and alternative types of statistical analysis.
更多查看译文
关键词
Animal social network analysis,Mixed models,Node-label permutations,Permutation tests
AI 理解论文
溯源树
样例
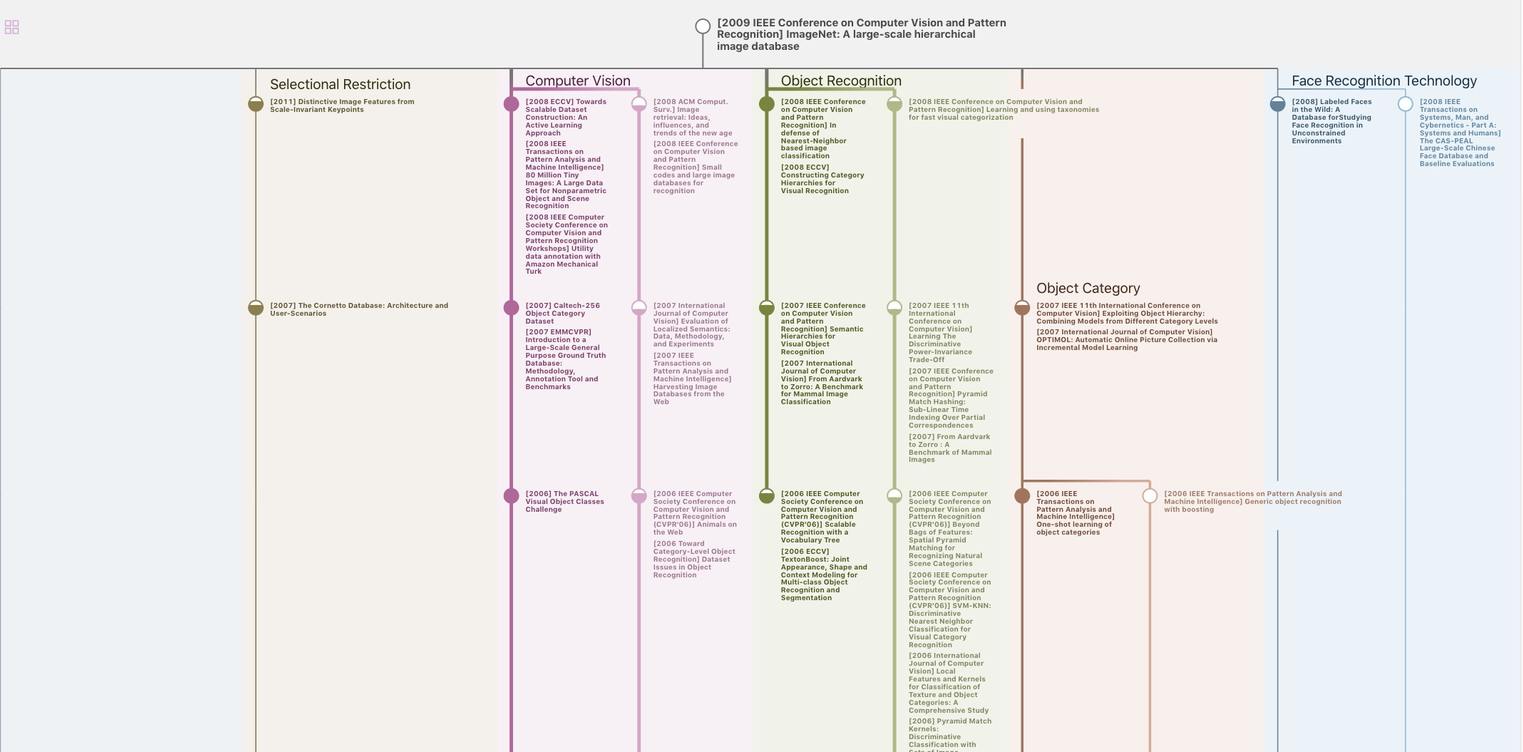
生成溯源树,研究论文发展脉络
Chat Paper
正在生成论文摘要