Robust Segmentation of Cellular Ultrastructure on Sparsely Labeled 3D Electron Microscopy Images using Deep Learning
Frontiers in Bioinformatics(2021)
Abstract
A deeper understanding of the cellular and subcellular organization of tumor cells and their interactions with the tumor microenvironment will shed light on how cancer evolves and guide effective therapy choices. Electron microscopy (EM) images can provide detailed view of the cellular ultrastructure and are being generated at an ever-increasing rate. However, the bottleneck in their analysis is the delineation of the cellular structures to enable interpretable rendering. We have mitigated this limitation by using deep learning, specifically, the ResUNet architecture, to segment cells and subcellular ultrastructure. Our initial prototype focuses on segmenting nuclei and nucleoli in 3D FIB-SEM images of tumor biopsies obtained from patients with metastatic breast and pancreatic cancers. Trained with sparse manual labels, our method results in accurate segmentation of nuclei and nucleoli with best Dice score of 0.99 and 0.98 respectively. This method can be extended to other cellular structures, enabling deeper analysis of inter- and intracellular state and interactions.
### Competing Interest Statement
JWG has licensed technologies to Abbott Diagnostics; has ownership positions in Convergent Genomics, Health Technology Innovations, Zorro Bio and PDX Pharmaceuticals; serves as a paid consultant to New Leaf Ventures; has received research support from Thermo Fisher Scientific (formerly FEI), Zeiss, Miltenyi Biotech, Quantitative Imaging, Health Technology Innovations and Micron Technologies; and owns stock in Abbott Diagnostics, AbbVie, Alphabet, Amazon, Amgen, Apple, General Electric, Gilead, Intel, Microsoft, Nvidia, and Zimmer Biomet.
MoreTranslated text
AI Read Science
Must-Reading Tree
Example
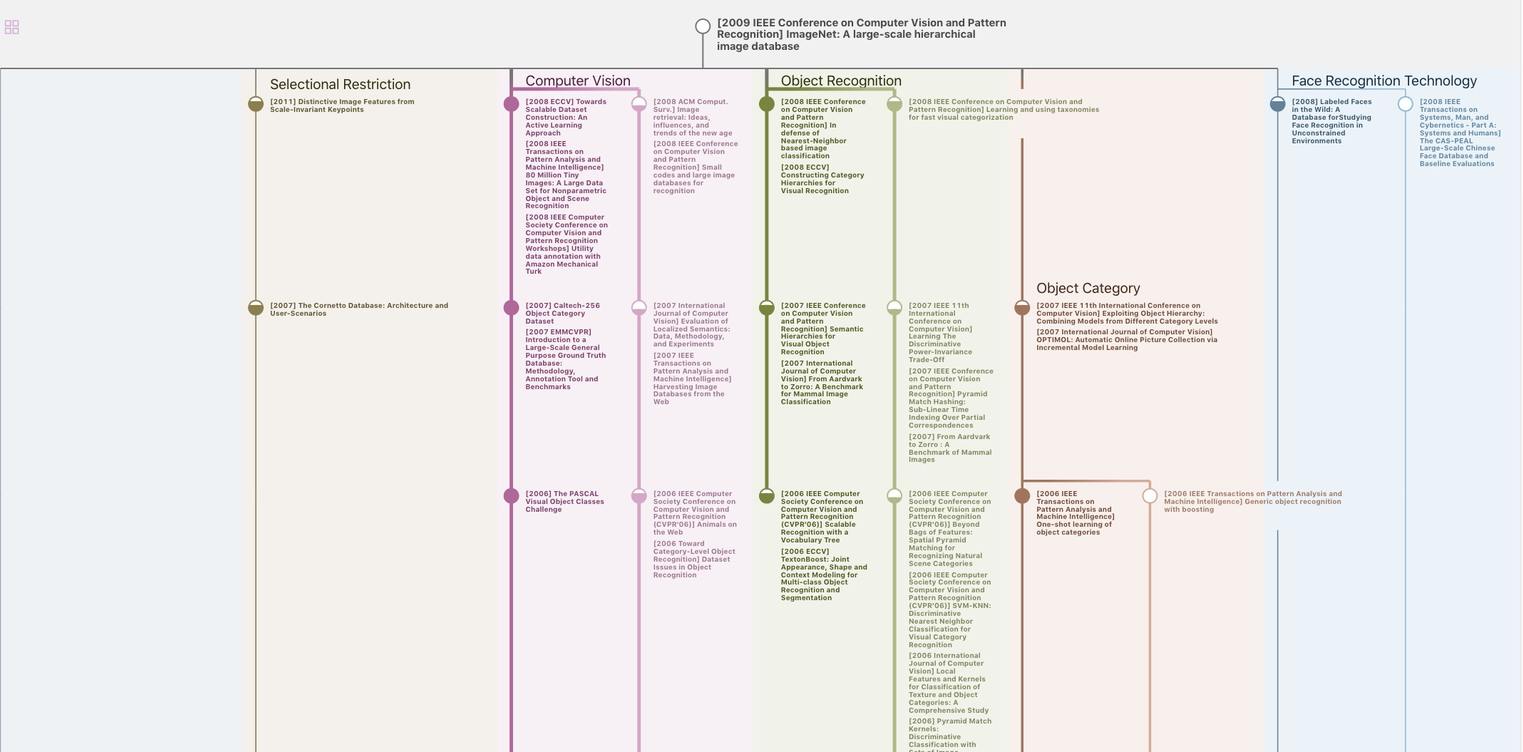
Generate MRT to find the research sequence of this paper
Chat Paper
Summary is being generated by the instructions you defined