Image-based Motion Artifact Reduction on Liver Dynamic Contrast Enhanced MRI
Physica Medica(2021)
摘要
Liver MRI images often suffer degraded quality from ghosting or blurring artifact caused by patient respiratory or bulk motion. In this study, we developed a two-stage deep learning model to reduce motion artifact on dynamic contrast enhanced (DCE) liver MRIs. The stage-I network utilized a deep residual network with a densely connected multi-resolution block (DRN-DCMB) network to remove the majority of motion artifacts. The stage-II network applied the perceptual loss to preserve image structural features by updating the parameters of the stage-I network via backpropagation. The stage-I network was trained using small image patches simulated with five types of motion, i.e., rotational, sinusoidal, random, elastic deformation and through-plane, to mimic actual liver motion patterns. The stage-II network training used full-size images with the same types of motion as the stage-I network. The motion reduction deep learning model was testing using simulated motion images and images with real motion artifacts. The resulted images after two-stage processing demonstrated substantially reduced motion artifacts while preserved anatomic details without image blurriness. This model outperformed existing methods of motion reduction artifact on liver DCE-MRI.
### Competing Interest Statement
The authors have declared no competing interest.
* DCE-MRI
: dynamic contrast enhanced magnetic resonance imaging
DRN-DCMB
: deep residual network with densely connected multi-resolution block
CS
: compressed sensing
CNNs
: convolutional neural networks
DL
: deep learning
3D
: three-dimensional
FFT
: Fast Fourier Transform
BN
: batch normalization
LeakyReLU
: Leaky rectified linear unit
MSE
: mean square error
SSIM
: structural similarity index
TR
: repetition time
TE
: echo time
FA
: flip angle
MARC
: motion artifact reduction with convolution
更多查看译文
AI 理解论文
溯源树
样例
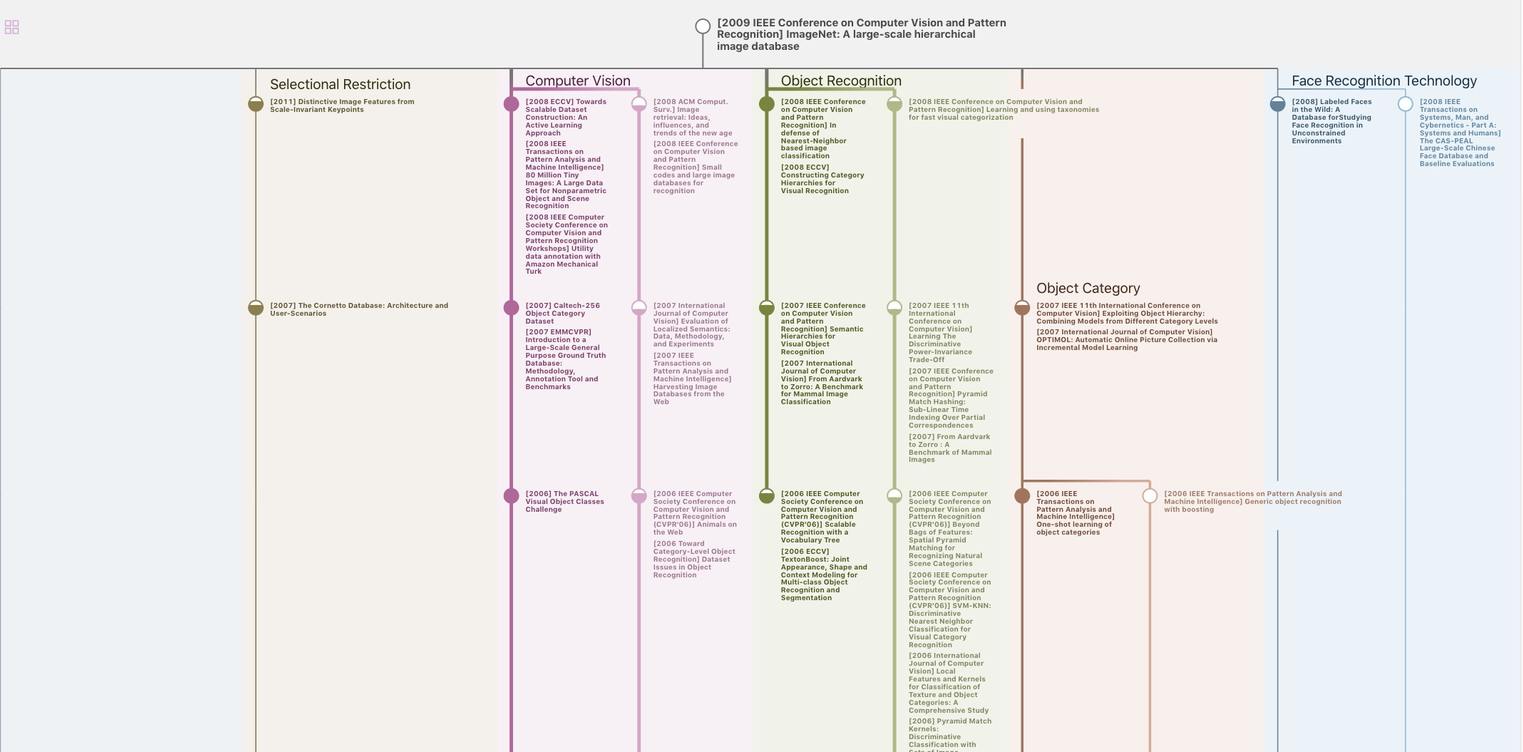
生成溯源树,研究论文发展脉络
Chat Paper
正在生成论文摘要