Privacy Preserving RNA-Model Validation Across Laboratories
biorxiv(2021)
摘要
Reproducibility of results obtained using RNA data across labs remains a major hurdle in cancer research. Often, molecular predictors trained on one dataset cannot be applied to another due to differences in RNA library preparation and quantification. While current RNA correction algorithms may overcome these differences, they require access to all patient-level data, which necessitates the sharing of training data for predictors when sharing predictors. Here, we describe SpinAdapt, an unsupervised RNA correction algorithm that enables the transfer of molecular models without requiring access to patient-level data. It computes data corrections only via aggregate statistics of each dataset, thereby maintaining patient data privacy. Furthermore, SpinAdapt can correct new samples, thereby enabling evaluation of validation cohorts. Despite an inherent tradeoff between privacy and performance, SpinAdapt outperforms current correction methods that require patient-level data access. We expect this novel correction paradigm to enhance research reproducibility and patient privacy. Finally, SpinAdapt lays a mathematical framework that can be extended to other -omics modalities.
### Competing Interest Statement
All authors have a financial relationship as employees of Tempus Labs, Inc.
* #### Algorithm Details: Glossary
![Graphic][1]
: The train source dataset
![Graphic][2]
: The train target dataset
![Graphic][3]
: The held-out source dataset
X s,i ∈ R p
: The i-th column of X s
X t, i ∈ R p
: The i-th column of X t
m s ∈ R p
: The empirical gene-wise mean of source dataset
m t ∈ R p
: The empirical gene-wise mean of target dataset
s s ∈ R p
: The empirical gene-wise variance of source dataset
s t ∈ R p
: The empirical gene-wise variance of target dataset
C s ∈ R p × d
: The empirical covariance of source dataset
C t ∈ R p × d
: The empirical covariance of target dataset
![Graphic][4]
: Principal Component factors for source dataset
![Graphic][5]
: Principal Component factors for target dataset
![Graphic][6]
: Transformation matrix
![Graphic][7]
: The corrected output source dataset
X ( i,j )
: The i-th row and j-th column of any matrix X
ν ( i )
: The i-th entry of any vector ν
F t
: Classifier trained on the target dataset
[1]: /embed/inline-graphic-1.gif
[2]: /embed/inline-graphic-2.gif
[3]: /embed/inline-graphic-3.gif
[4]: /embed/inline-graphic-4.gif
[5]: /embed/inline-graphic-5.gif
[6]: /embed/inline-graphic-6.gif
[7]: /embed/inline-graphic-7.gif
更多查看译文
关键词
rna-model
AI 理解论文
溯源树
样例
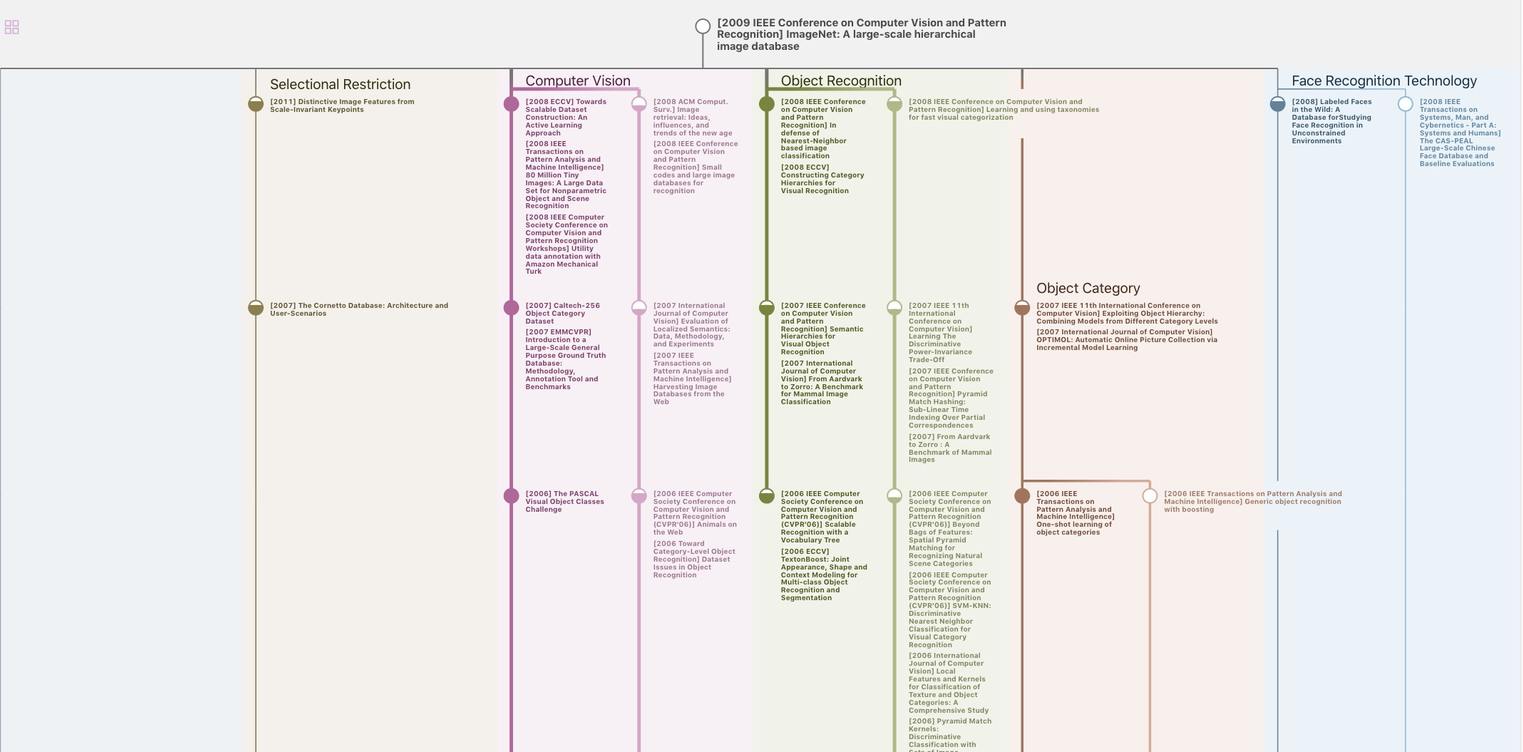
生成溯源树,研究论文发展脉络
Chat Paper
正在生成论文摘要