To Supervise or Not to Supervise: How to Effectively Learn Wireless Interference Management Models?
SPAWC(2021)
摘要
Machine learning has become successful in solving wireless interference management problems. Different kinds of deep neural networks (DNNs) have been trained to accomplish key tasks such as power control, beamforming and admission control. There are two state-of-the-art approaches to train such DNNs based interference management models: supervised learning (i.e., fits labels generated by an optimization algorithm) and unsupervised learning (i.e., directly optimizes some system performance measure). However, it is by no means clear which approach is more effective in practice. In this paper, we conduct some theory and experiment study about these two training approaches. First, we show a somewhat surprising result, that for some special power control problem, the unsupervised learning can perform much worse than its counterpart, because it is more likely to get stuck at some low-quality local solutions. We then provide a series of theoretical results to further understand the properties of the two approaches. To our knowledge, these are the first set of theoretical results trying to understand different training approaches in learning-based wireless communication system design.
更多查看译文
关键词
wireless interference management models,machine learning,deep neural networks,DNNs,admission control,supervised learning,optimization algorithm,system performance measure,experiment study,special power control problem,unsupervised learning,low-quality local solutions,training approaches,learning-based wireless communication system design
AI 理解论文
溯源树
样例
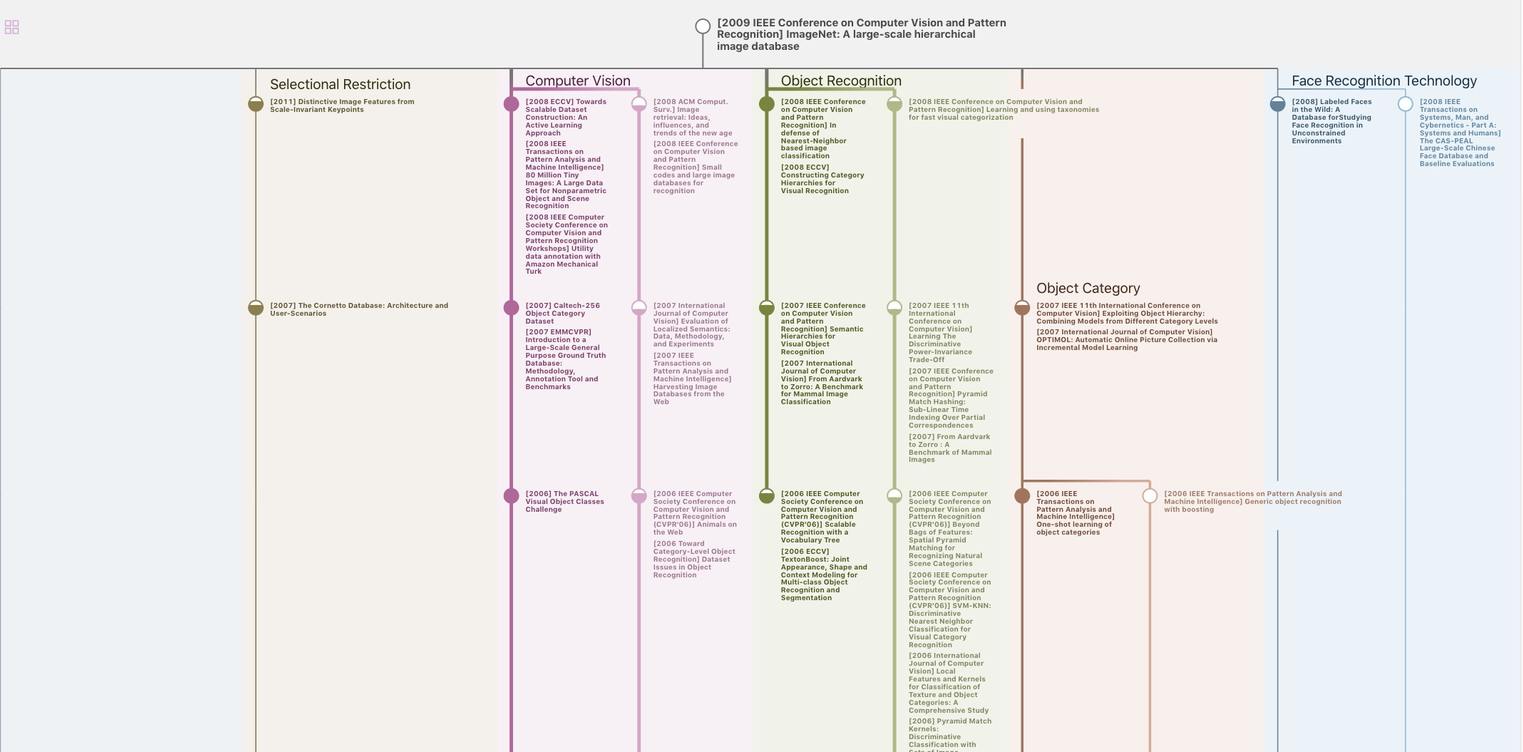
生成溯源树,研究论文发展脉络
Chat Paper
正在生成论文摘要