Elastic Weight Consolidation Continual Learning Based Signal Detection in Multiple Channel MIMO System
ICCC(2021)
摘要
In the multi-input multi-output (MIMO) signal detection based on deep learning, there is a wireless channel overtraining problem, that is, the signal detection network generally only remembers a single wireless channel model. An artificial neural network (ANN)-assisted receiver trained for a specific channel model is often not suitable for another channel model. In mobile communication, the wireless channel is greatly randomly affected by the wireless propagation environment. Variations in channel parameters will change the channel model, and this makes the signal detector based on deep learning use limited and poor robustness. In this paper, a continual learning method based on elastic weight consolidation(EWCCL) is proposed to solve the issue. The basic idea of this paper is to calculate the importance of each parameter to the task through the EWCCL method after the completion of one training. Then, in the next training, regular terms are added into the loss function to avoid the result of the last training being completely covered due to excessive deviation of these parameters. This method can make the neural network memorize the training results of multiple wireless channels, so it has stronger robustness to the transformation of wireless channels, and also reduces the cost of retraining. Finally, we verified this hypothesis through simulation experiments. The simulation experiment results show that the EWCCL method proposed in this paper has better detection performance and better robustness than the fine-tune method.
更多查看译文
关键词
massive multiple input multiple output,elastic weight consolidation,continual learning,deep learning,signal detection
AI 理解论文
溯源树
样例
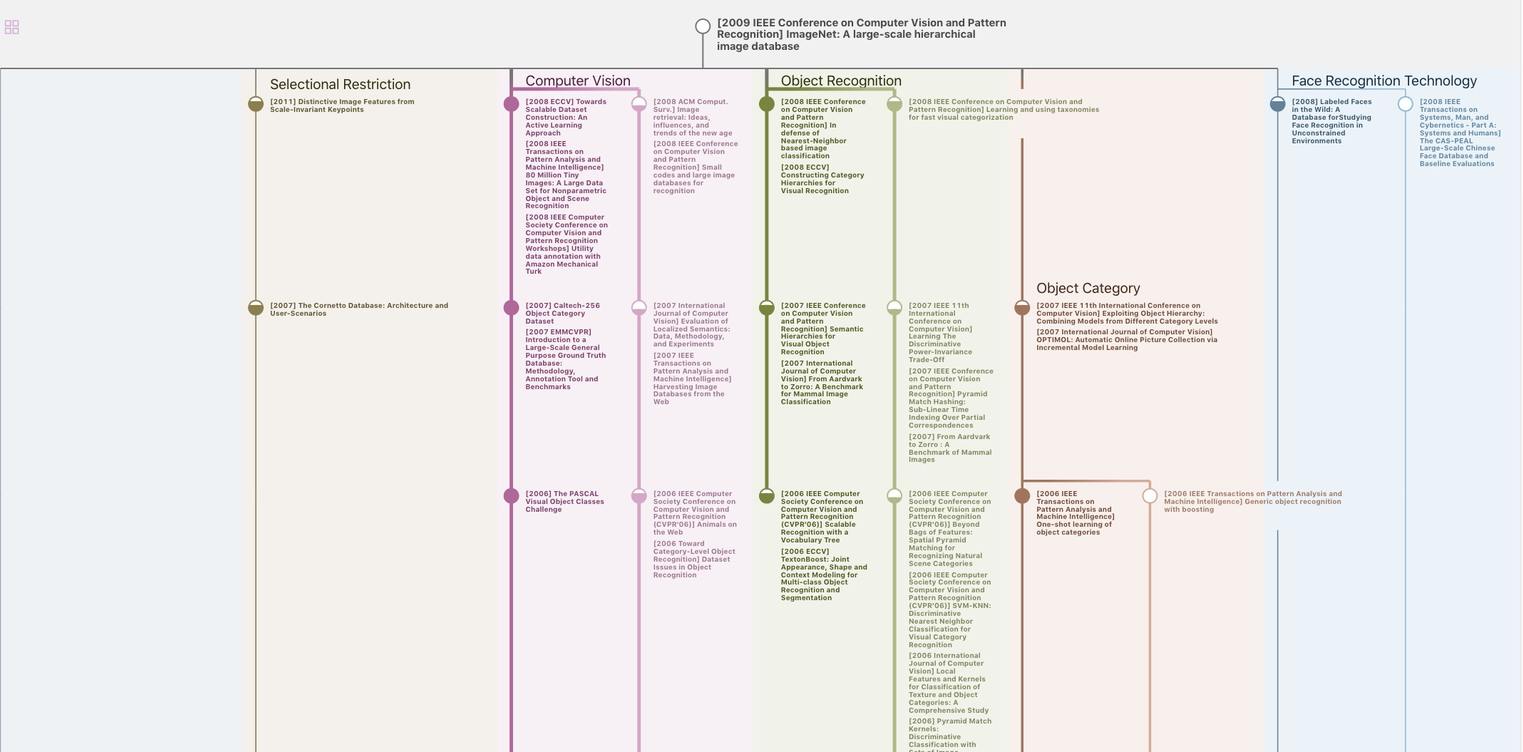
生成溯源树,研究论文发展脉络
Chat Paper
正在生成论文摘要