A neural network strategy for supervised classification via the Learning Under Privileged Information paradigm.
SEBD(2021)
摘要
Devising new methodologies to handle and analyse Big Data has become a fundamental task in our increasingly service-oriented and interconnected society. One of the problems arising while handling such data is that, for a given set of entities, not all the entities may be described at the same level of detail, i.e., the number of features describing each entity may vary. In general cases, in order to apply classic data science methods, it is necessary to have a common features set over a data set. This will then correspond to the maximum number of common features among the entities, resulting in a loss of information for the entities for which additional information may be available. In order to exploit such additional information, the Learning Using Privileged Information (LUPI) paradigm has been proposed, based on the use of the teacher role in the learning process. In this schema the teacher acquires a strategic position, by exploiting at the training stage some additional privileged information about the entities, which will not be available at the test stage. In this work, we apply this paradigm in the context of neural networks, by proposing a LUPI based deep learning architecture able to exploit a larger set of attributes at training time, with the aim to improve classification performances on a set of entities associated to a reduced attribute set. Experimental results show how the proposed approach improves upon the ones applying the same schema to classic machine learning methods (e.g., SVM).
更多查看译文
关键词
privileged information paradigm,supervised classification,neural network strategy,neural network
AI 理解论文
溯源树
样例
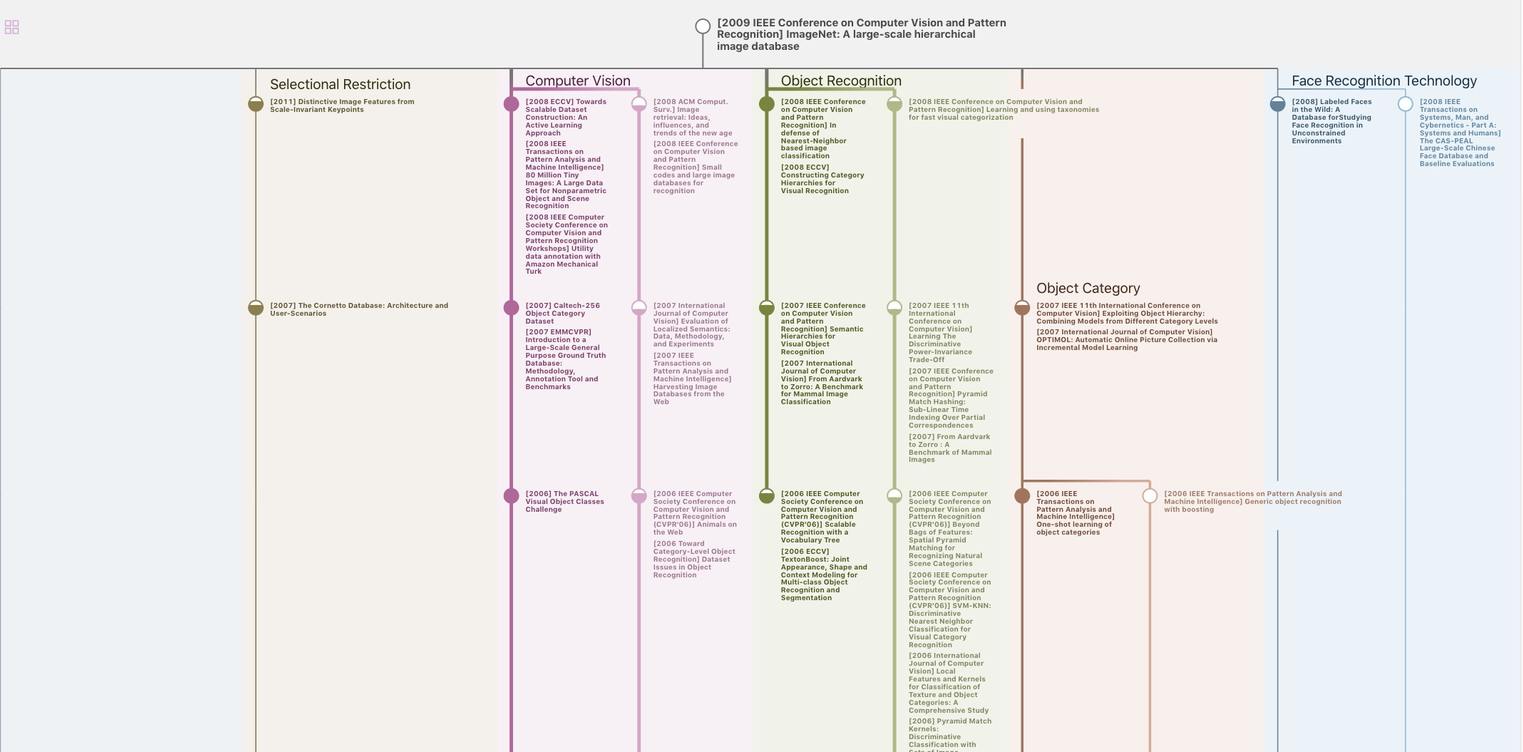
生成溯源树,研究论文发展脉络
Chat Paper
正在生成论文摘要