Faster Deployment for Indoor Visible Light Positioning Using Xgboost Algorithms in Industrial Internet-of-Things
IECON 2021 - 47TH ANNUAL CONFERENCE OF THE IEEE INDUSTRIAL ELECTRONICS SOCIETY(2021)
摘要
In recent years, as an evolution of a distributed control system, the industrial Internet-of-Things (IIoT) enables high-degree automation in smart factories or workshops, and full location-awareness of all the interconnected instruments and devices are crucial for realizing IIoT. In this paper, a machinelearning-based indoor visible light positioning (VLP) system for IIoT is proposed and implemented in pursuit of significant reduction of time for offline preparation. An Xgboost-based position estimator using received signal strength (RSS) from light-emitting diode (LED) lighting is implemented. Several other popular machine-learning-based regression algorithms, including deep neural network (DNN), support vector machine (SVM), random forest (RF) are adopted in the position estimator for experimental validation and performance comparison. In the experiment, the receiver was mounted on a smart trolley in a smart workshop, and the two-dimensional positioning performance was studied by placing the smart trolley in several positions within the coverage area. According to the experimental results, the performance of using Xgboost significantly outperforms others in terms of training speed while maintaining comparable positioning accuracy.
更多查看译文
关键词
Visible light positioning (VLP), received signal strength (RSS), machine learning, XGboost, artificial neural network (ANN), random forest (RF), visible light communication (VLC)
AI 理解论文
溯源树
样例
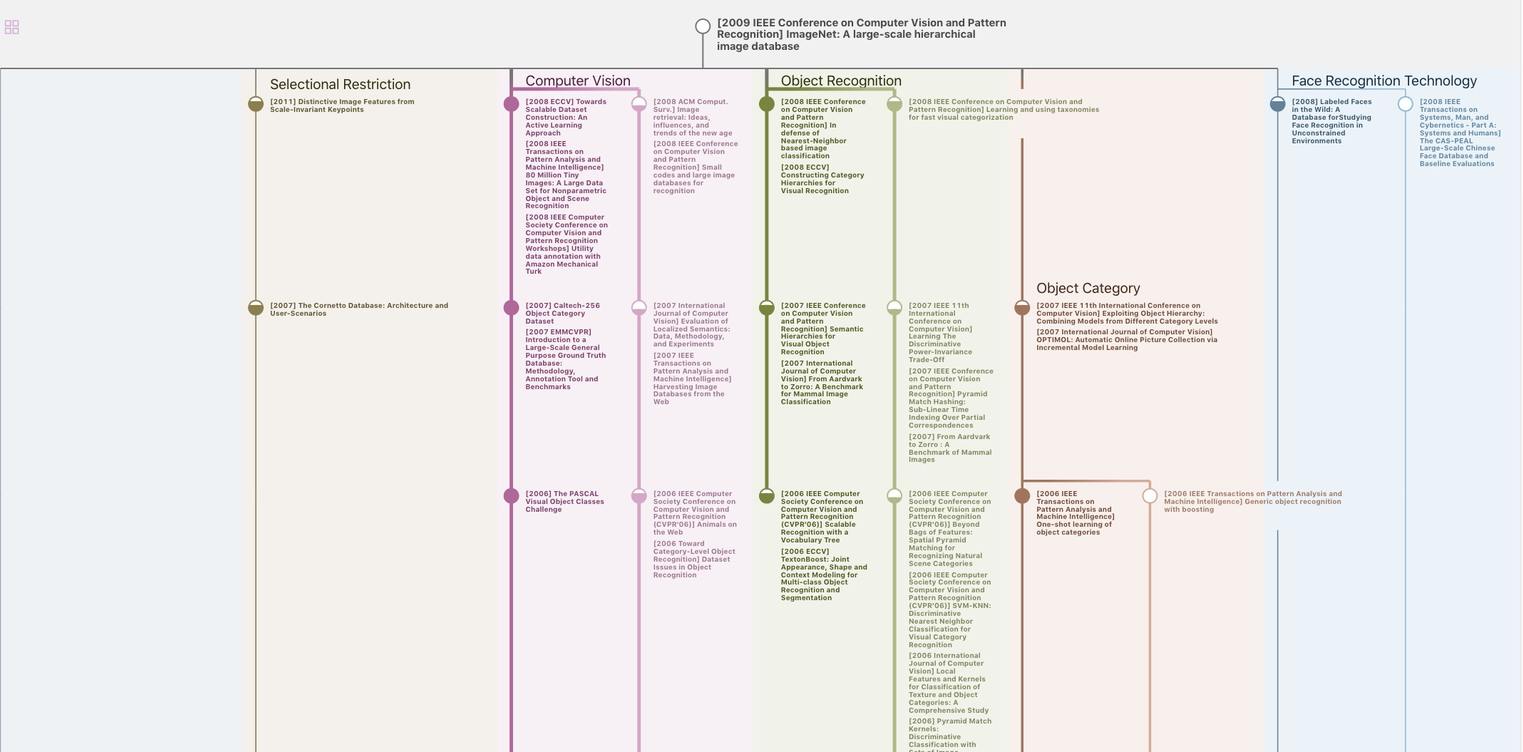
生成溯源树,研究论文发展脉络
Chat Paper
正在生成论文摘要