Knowledge driven rapid development of white box digital twins for industrial plant systems
IECON 2021 - 47TH ANNUAL CONFERENCE OF THE IEEE INDUSTRIAL ELECTRONICS SOCIETY(2021)
摘要
Industrial systems like power plants need a significant domain and engineering expertise for their efficient operations. Experts make decisions for multiple operational objectives such as minimizing cost, maximizing productivity, ensuring compliance to environmental conditions, etc. However, deciding strategies to achieve an objective can be highly non-trivial as it may lead to conflicting outcomes concerning other objectives. Modern digital twin(DT) technologies make it possible to build systems to support such decision-making to validate critical decisions. DTs are developed as one-off solutions for individual plants in the current practice, requiring high efforts and domain knowledge. Our work demonstrates the use of a knowledge-driven approach to reduce DT development efforts significantly. The main contribution of our work is an end-to-end architecture that allows us to explicate, structure, and reuse domain knowledge to semi-automate DT development for industrial plants. We integrate technologies like semantic web, model-driven engineering, and formal methods to realize the architecture. Our approach to developing a DT supporting a fault management scenario in a power plant reduced 70% development time and 50% manual efforts.
更多查看译文
关键词
knowledge-driven, digital twins, fault management, FMEA, transition system, state machines, domain-knowledge
AI 理解论文
溯源树
样例
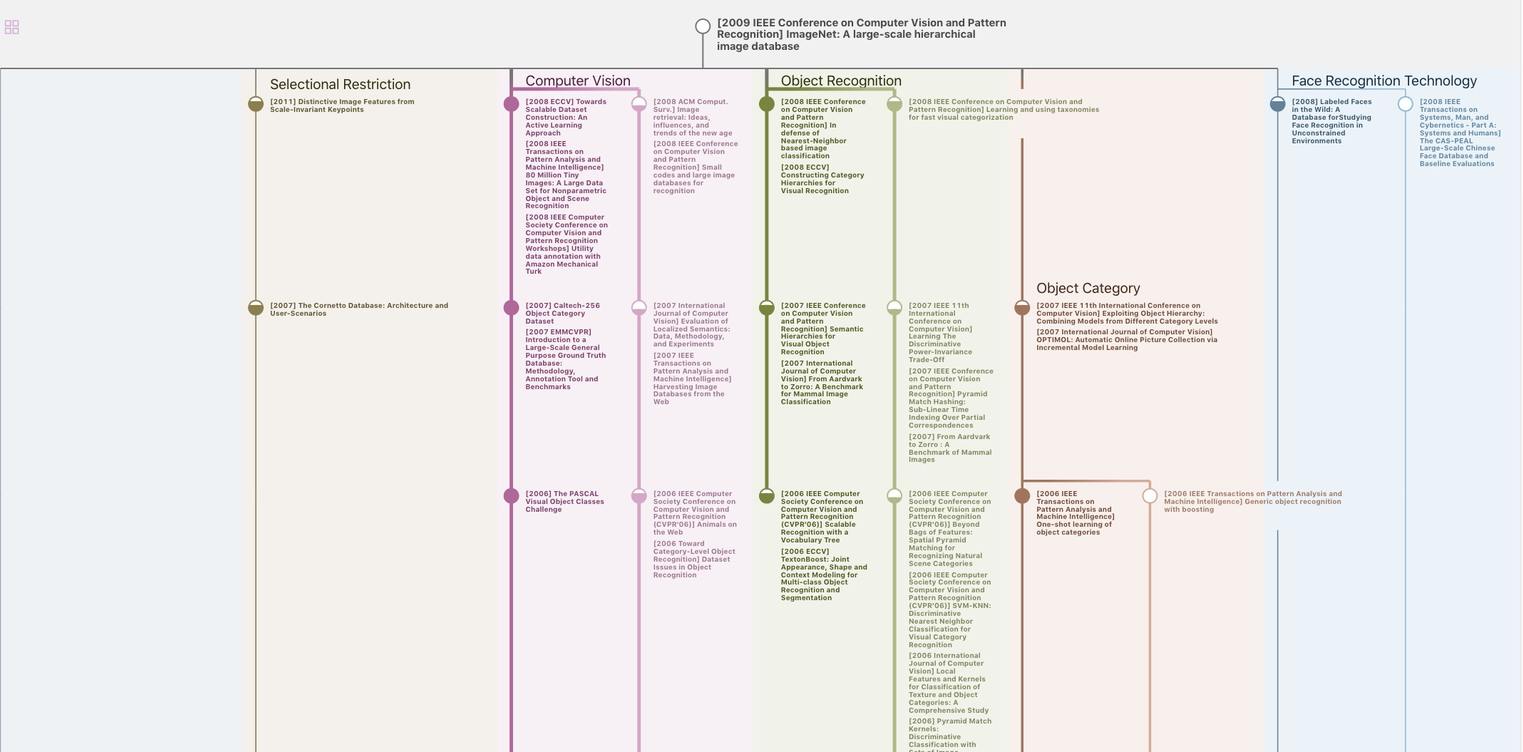
生成溯源树,研究论文发展脉络
Chat Paper
正在生成论文摘要