Inferring Cost Functions Using Reward Parameter Search and Policy Gradient Reinforcement Learning
IECON 2021 - 47TH ANNUAL CONFERENCE OF THE IEEE INDUSTRIAL ELECTRONICS SOCIETY(2021)
摘要
This study focuses on inferring cost functions of obtained movement data using reward parameter search and policy gradient based Reinforcement Learning (RL). The behavior data for this task is obtained through a series of squat-to-stand movements of human participants under dynamic perturbations. The key parameter searched in the cost function is the weight of total torque used in performing the squat-to-stand action. An approximate model is used to learn squat-to-stand movements via a policy gradient method, namely Proximal Policy Optimization(PPO). A behavioral similarity metric based on Center of Mass(COM) is used to find the most likely weight parameter. The stochasticity in the training result of PPO is dealt with multiple runs, and as a result, a reasonable and a stable Inverse Reinforcement Learning(IRL) algorithm is obtained in terms of performance. The results indicate that for some participants, the reward function parameters of the experts were inferred successfully.
更多查看译文
关键词
cost functions,reward parameter search,policy gradient reinforcement learning,behavior data,cost function,policy gradient method,behavioral similarity metric,reward function parameters,proximal policy optimization,PPO,IRL algorithm
AI 理解论文
溯源树
样例
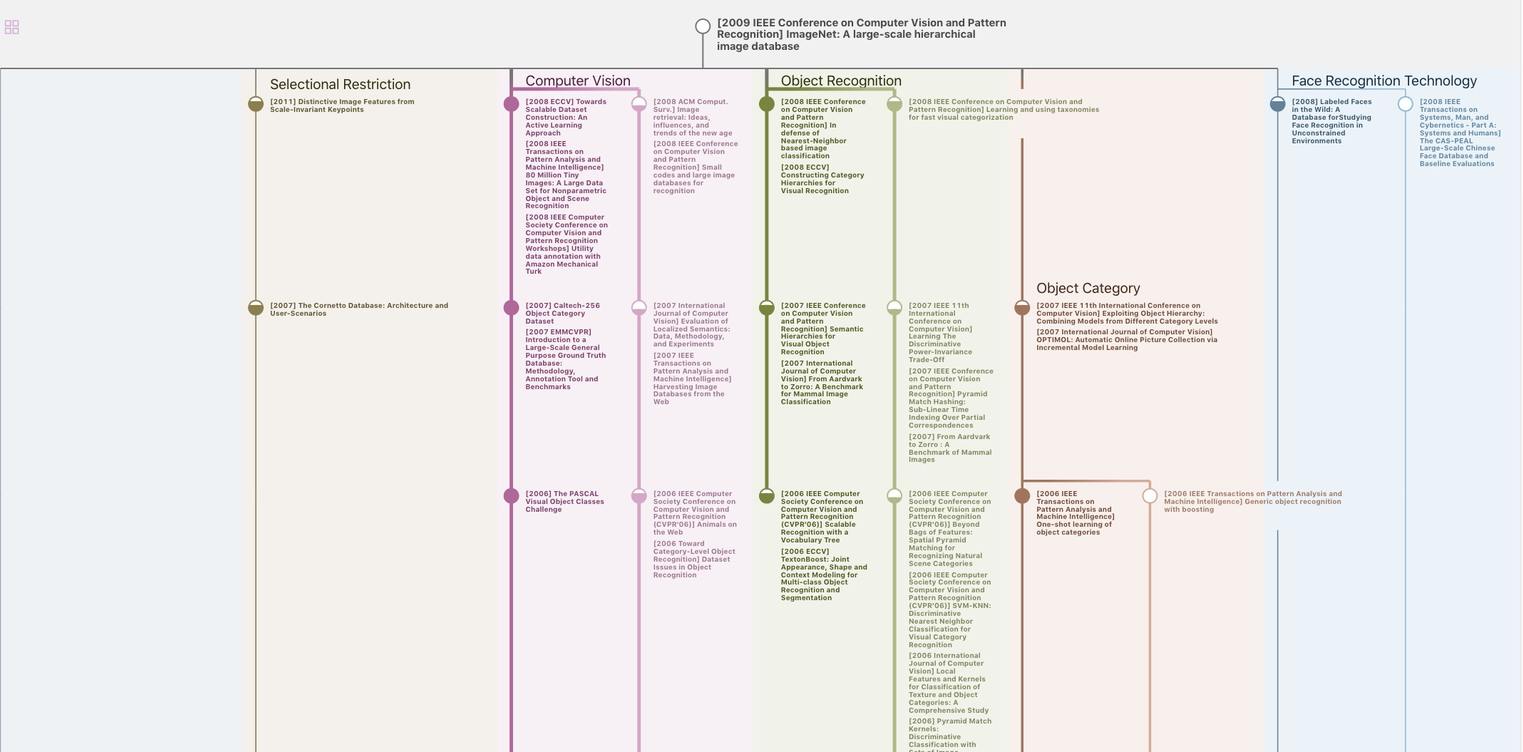
生成溯源树,研究论文发展脉络
Chat Paper
正在生成论文摘要