Deep learning super-resolution electron microscopy based on deep residual attention network
INTERNATIONAL JOURNAL OF IMAGING SYSTEMS AND TECHNOLOGY(2021)
摘要
Field-emission scanning electron microscopy has become a fundamental research tool in the fields of medicine and materials science owing to its effectiveness. However, an inherent contradiction exists between the resolution of field-emission scanning electron microscopy and its field-of-view. To solve this problem, we propose a deep learning-based method for electron microscopy that can simultaneously obtain a large field-of-view and ultrahigh resolution. To solve the super-resolution problem, a deep residual attention network is designed based on residual learning and the attention mechanism, wherein the backbone network employs several residual groups to effectively extract the features; moreover, attention groups append the backbone part to refine and fuse the features. Besides, a high-frequency information retention module is added to acquire high-frequency signals, acting as an effective complement to the deep residual attention network. Owing to the lack of super-resolution datasets for electron microscopy, we created a microscopic butterfly wing dataset. In the experiments, MixUp was also applied to super-resolution problem as a simple and effective data augmentation method, to provide more data to train the model. To evaluate the proposed method, we used standard and self-made datasets with peak signal-to-noise ratio (PSNR) and structural similarity (SSIM) as criteria. The results show that the proposed model exhibits a state-of-the-art performance with a PSNR of 25.12 dB and an SSIM of 0.64, and makes field-emission scanning electron microscopy more practical and promising in the field of optical device optimization.
更多查看译文
关键词
attention mechanism, deep learning, electron microscope, residual learning, super‐, resolution
AI 理解论文
溯源树
样例
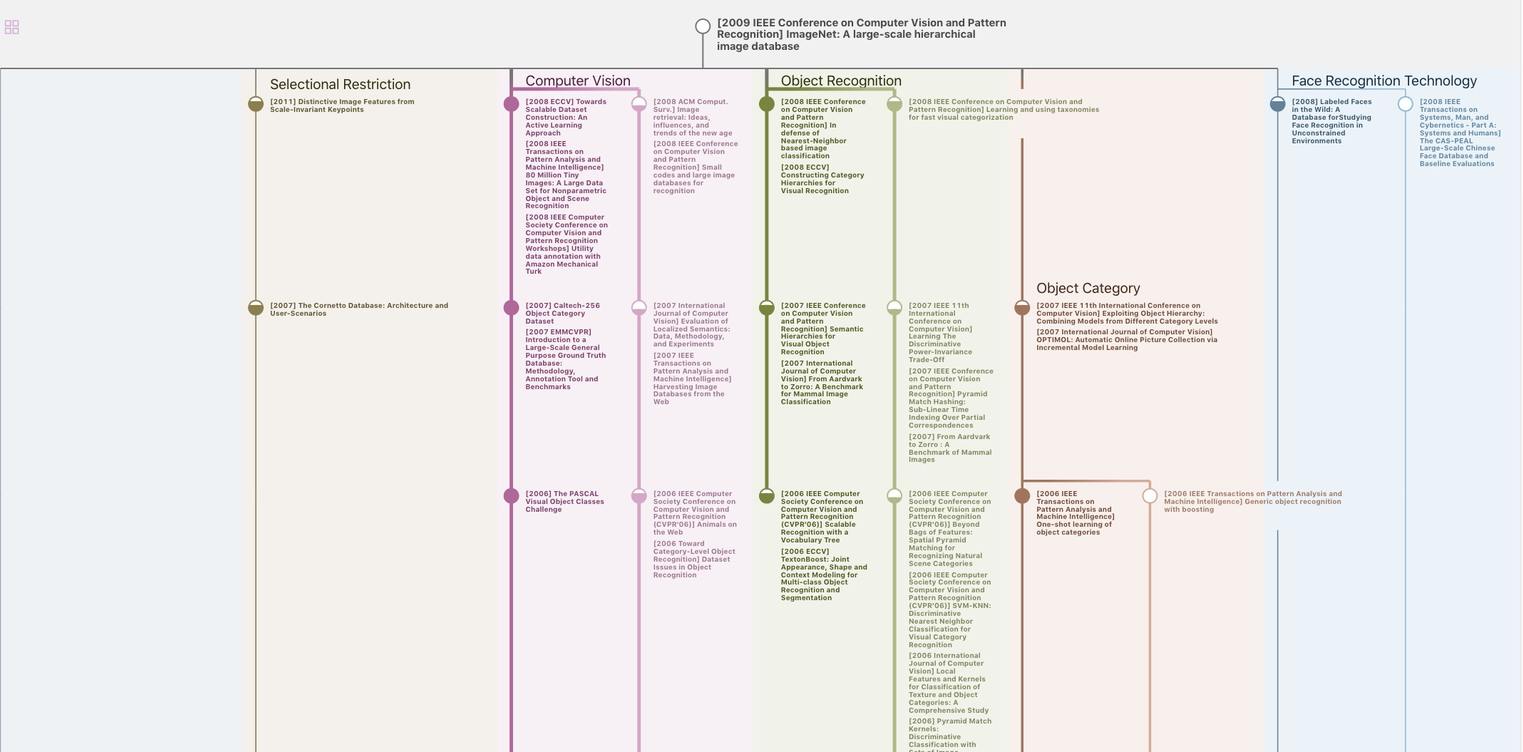
生成溯源树,研究论文发展脉络
Chat Paper
正在生成论文摘要