Computing all identifiable functions of parameters for ODE models
SYSTEMS & CONTROL LETTERS(2021)
摘要
Parameter identifiability is a structural property of an ODE model for recovering the values of parameters from the data (i.e., from the input and output variables). This property is a prerequisite for meaningful parameter identification in practice. In the presence of nonidentifiability, it is important to find all functions of the parameters that are identifiable. The existing algorithms check whether a given function of parameters is identifiable or, under the solvability condition, find all identifiable functions. However, this solvability condition is not always satisfied, which presents a challenge. Our first main result is an algorithm that computes all identifiable functions without any additional assumptions, which is the first such algorithm as far as we know. Our second main result concerns the identifiability from multiple experiments (with generically different inputs and initial conditions among the experiments). For this problem, we prove that the set of functions identifiable from multiple experiments is what would actually be computed by input-output equation-based algorithms (whether or not the solvability condition is fulfilled), which was not known before. We give an algorithm that not only finds these functions but also provides an upper bound for the number of experiments to be performed to identify these functions. We provide an implementation of the presented algorithms. (c) 2021 The Authors. Published by Elsevier B.V.
更多查看译文
关键词
Parameter identifiability, Multiple experiments, Input-output equations, Differential algebra, Characteristic sets
AI 理解论文
溯源树
样例
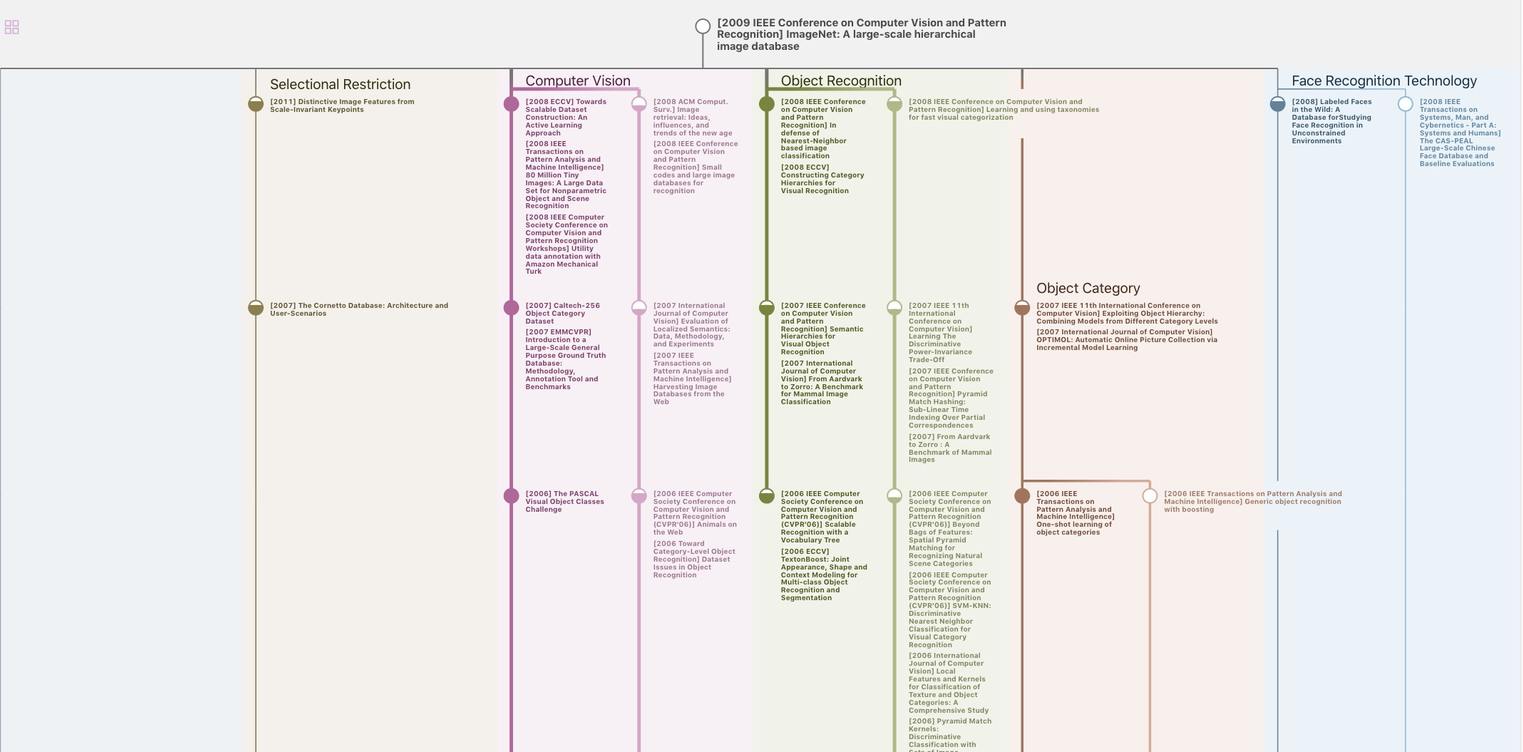
生成溯源树,研究论文发展脉络
Chat Paper
正在生成论文摘要